Introduction #
The authors of the MineralImage5k dataset make a significant contribution by introducing an open benchmark for zero-shot raw mineral visual recognition. The dataset consits of more than 5 thousand mineral species, covering almost the span of existing minerals on the Earth. Beyond the zero-shot classification dataset, subsets are provided for segmentation, mineral size estimation, and few-shot classification. Baseline solutions for these computer vision problems are published, inviting the community to surpass them.
Importance of Mineral Diagnostics
Nature boasts about 6000 known minerals and their varieties, with only a fraction being rock-forming or of industrial interest. Accurate mineral diagnostics is crucial for geological work, enabling geological mapping and deposit exploration. However, exact mineral identification is a complex and time-consuming task, requiring significant skill and time investment by geologists. The involvement of machine intelligence in visual diagnostics aims to optimize this process, freeing up professional mineralogistsβ time for more complex tasks and identifying errors in visual diagnostics.
The integration of machine intelligence in mineral diagnostics, particularly in raw samples, holds promising implications. If an algorithm can enable visual diagnostics of minerals at the level of an ordinary geologist, it opens avenues for creating search robots for exploring challenging terrains on Earth and potentially on other planets. The addition of visual diagnostics capabilities complements existing methods like IR spectroscopy and Raman spectroscopy, enhancing the selection of objects for analysis and expanding research possibilities.
Dataset Collection and preprocessing
For dataset creation, the authors utilized the Fersman mineralogical museum image set, one of the largest mineralogical collections globally, containing over 170000 samples of about 5000 mineral species. The dataset provides a diverse and representative view of mineral diversity, offering advantages over other datasets.
The authors implemented a comprehensive image preprocessing pipeline to enhance the datasetβs quality and representation. This involved removing uninformative images, handling duplicates, and resizing images to a standardized format. The pipeline also addressed potential issues related to text on images, distinguishing between minerals, reference cubes, and text plates.
Formation of Multiple Datasets
The filtered and cropped images were used to create multiple datasets, including a zero-shot classification dataset and subsets for few-shot classification. Additionally, auxiliary datasets for segmentation and mineral size estimation were provided, featuring manually labeled mineral and satellite masks and manually measured mineral sizes, respectively.
Classes | Images | Minimum images per class | Task | Subsets |
---|---|---|---|---|
5139 | 44784 | 1 | Classification | Test |
360 | 31982 | 17 | Classification | Train&Test |
98 | 23496 | 78 | Classification | Train&Test |
36 | 16001 | 187 | Classification | Train&Test |
10 | 8549 | 462 | Classification | Train&Test |
β | >100 | β | Segmentation | Test |
β | 18076 | β | Regression (size estimation) | Test |
The proposed subsets with different classes definition.
Summary #
MineralImage5k: A Benchmark for Zero-Shot Raw Mineral Visual Recognition and Description is a dataset for object detection, semantic segmentation, and classification tasks. It is used in the geological research.
The dataset consists of 19207 images with 31393 labeled objects belonging to 6 different classes including stone, rock, mineral, and other: gem, crystal, and mineral ore.
Images in the MineralImage5k dataset have bounding box annotations. All images are labeled (i.e. with annotations). There are 8 splits in the dataset: 1_syst (15005 images), 7_stepanov (1361 images), 5_PDK (1057 images), 3_op (745 images), 2_mest (561 images), 4_cryst (220 images), 10_meteor (151 images), and segm (107 images). Additionally, the images have following tags: name, description (dsc), size_sm. The dataset was released in 2023 by the Sber AI, Russia, Artificial Intelligence Research Institute, Russia, and Fersman Mineralogical Museum, Russia.
Here is the visualized example grid with annotations:
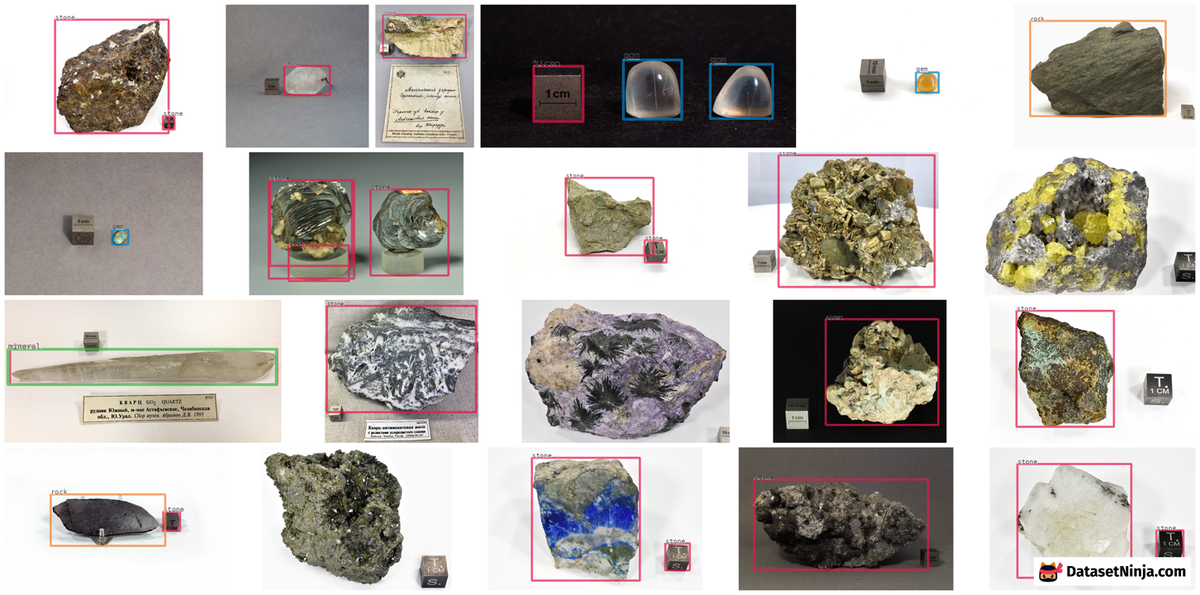
Explore #
MineralImage5k dataset has 19207 images. Click on one of the examples below or open "Explore" tool anytime you need to view dataset images with annotations. This tool has extended visualization capabilities like zoom, translation, objects table, custom filters and more. Hover the mouse over the images to hide or show annotations.


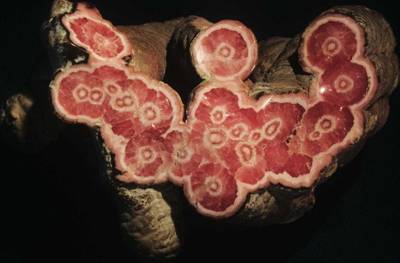
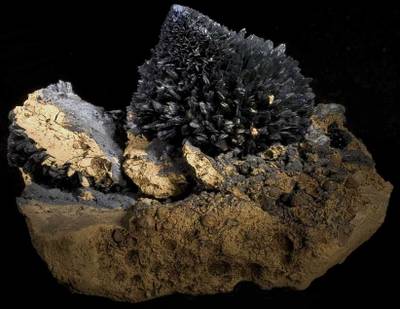
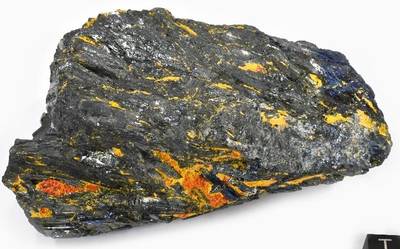
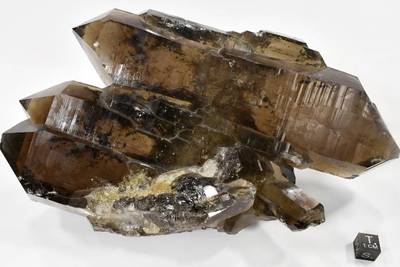
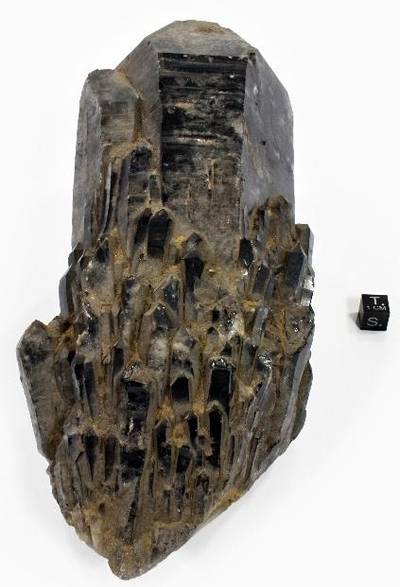

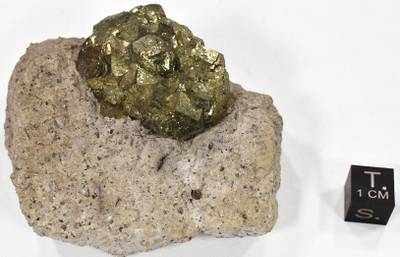
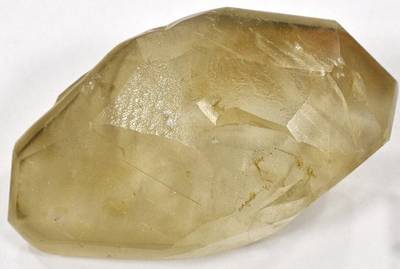
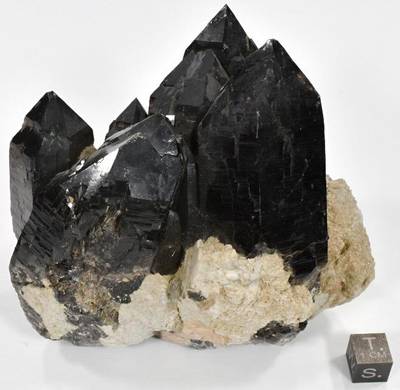
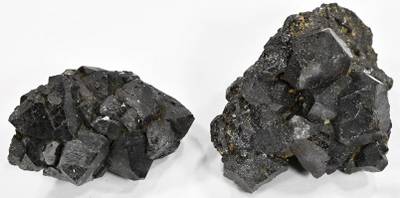
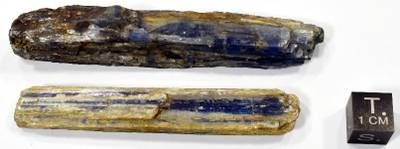
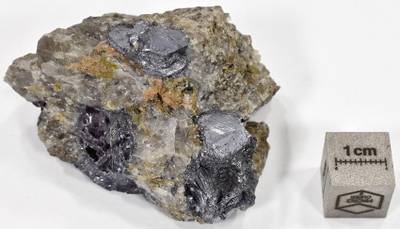
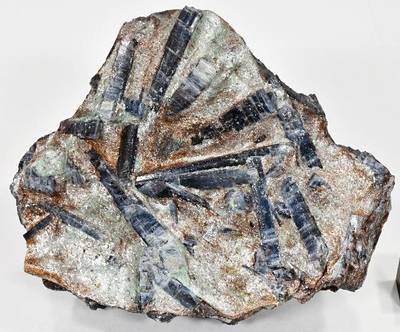
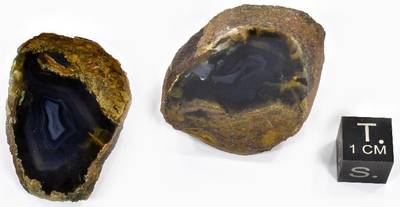
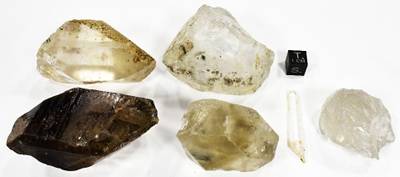
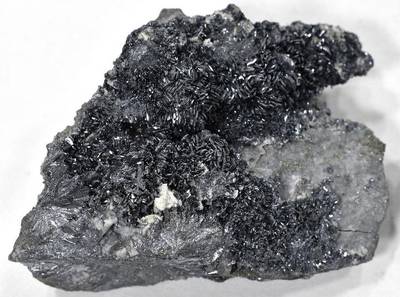
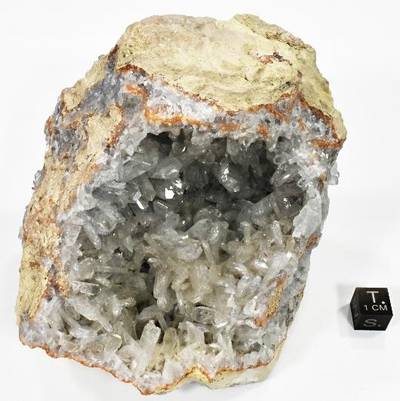
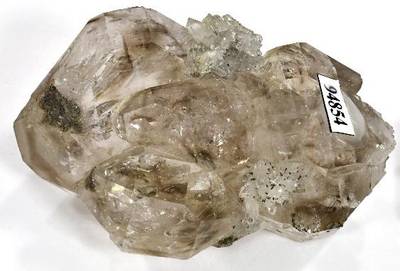
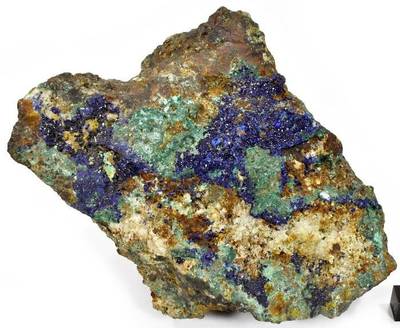
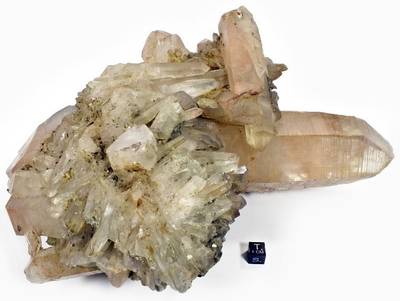
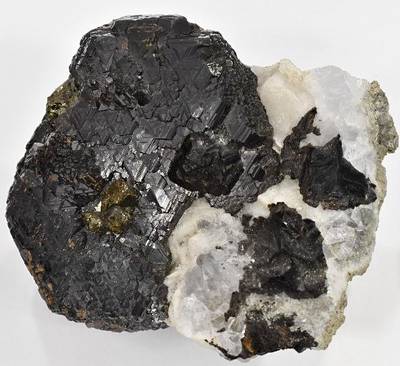
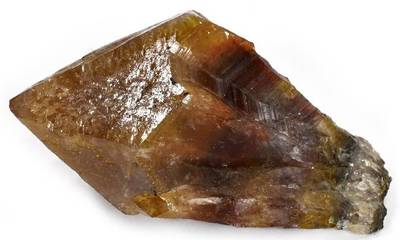


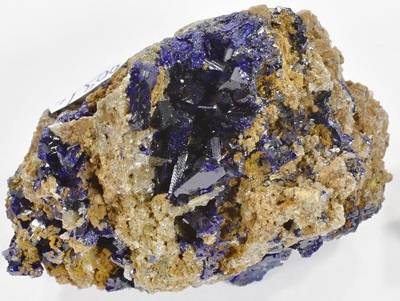
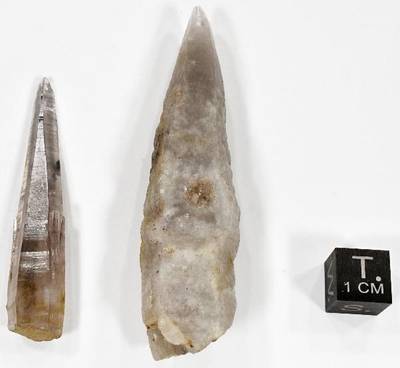
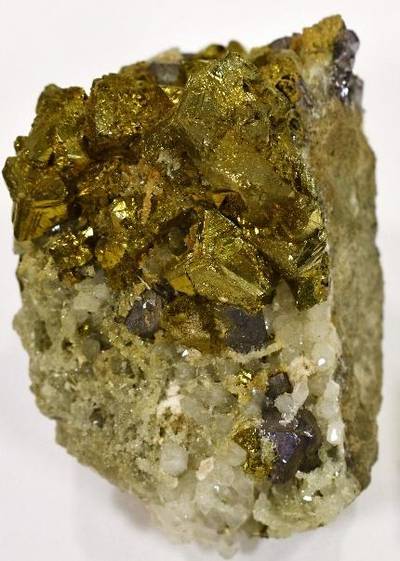
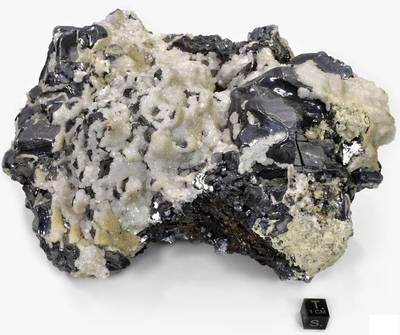
Class balance #
There are 6 annotation classes in the dataset. Find the general statistics and balances for every class in the table below. Click any row to preview images that have labels of the selected class. Sort by column to find the most rare or prevalent classes.
Class γ
€ | Images γ
€ | Objects γ
€ | Count on image average | Area on image average |
---|---|---|---|---|
stoneβ rectangle | 15486 | 21630 | 1.4 | 40.05% |
rockβ rectangle | 2874 | 3211 | 1.12 | 38.68% |
mineralβ any | 2279 | 3306 | 1.45 | 26.58% |
gemβ rectangle | 1223 | 1728 | 1.41 | 20.53% |
crystalβ rectangle | 656 | 766 | 1.17 | 33.08% |
mineral oreβ rectangle | 419 | 752 | 1.79 | 14.72% |
Co-occurrence matrix #
Co-occurrence matrix is an extremely valuable tool that shows you the images for every pair of classes: how many images have objects of both classes at the same time. If you click any cell, you will see those images. We added the tooltip with an explanation for every cell for your convenience, just hover the mouse over a cell to preview the description.
Images #
Explore every single image in the dataset with respect to the number of annotations of each class it has. Click a row to preview selected image. Sort by any column to find anomalies and edge cases. Use horizontal scroll if the table has many columns for a large number of classes in the dataset.
Object distribution #
Interactive heatmap chart for every class with object distribution shows how many images are in the dataset with a certain number of objects of a specific class. Users can click cell and see the list of all corresponding images.
Class sizes #
The table below gives various size properties of objects for every class. Click a row to see the image with annotations of the selected class. Sort columns to find classes with the smallest or largest objects or understand the size differences between classes.
Class | Object count | Avg area | Max area | Min area | Min height | Min height | Max height | Max height | Avg height | Avg height | Min width | Min width | Max width | Max width |
---|---|---|---|---|---|---|---|---|---|---|---|---|---|---|
stone rectangle | 21630 | 29.02% | 99.9% | 0.24% | 31px | 4.69% | 1011px | 100% | 385px | 49.91% | 36px | 3.52% | 1023px | 99.9% |
mineral any | 3306 | 18.92% | 94.58% | 0.28% | 35px | 3.8% | 940px | 99.8% | 285px | 40.21% | 43px | 4.2% | 1020px | 100% |
rock rectangle | 3211 | 34.87% | 99.9% | 0.47% | 37px | 7.62% | 1003px | 100% | 409px | 56.13% | 60px | 5.86% | 1023px | 99.9% |
gem rectangle | 1728 | 14.87% | 93.68% | 0.15% | 30px | 4.4% | 978px | 96.89% | 270px | 36% | 36px | 3.52% | 982px | 98.37% |
crystal rectangle | 766 | 29.81% | 93.37% | 0.64% | 59px | 7.6% | 1017px | 99.86% | 545px | 60.03% | 65px | 6.54% | 1014px | 99.35% |
mineral ore rectangle | 752 | 8.57% | 99.66% | 0.15% | 28px | 4.69% | 990px | 100% | 182px | 25.42% | 30px | 2.93% | 1023px | 99.9% |
Spatial Heatmap #
The heatmaps below give the spatial distributions of all objects for every class. These visualizations provide insights into the most probable and rare object locations on the image. It helps analyze objects' placements in a dataset.
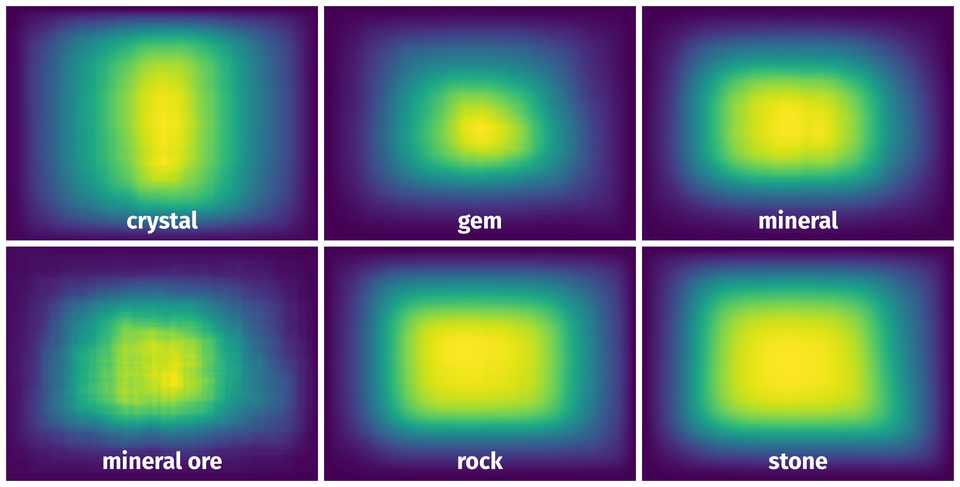
Objects #
Table contains all 31393 objects. Click a row to preview an image with annotations, and use search or pagination to navigate. Sort columns to find outliers in the dataset.
Object ID γ
€ | Class γ
€ | Image name click row to open | Image size height x width | Height γ
€ | Height γ
€ | Width γ
€ | Width γ
€ | Area γ
€ |
---|---|---|---|---|---|---|---|---|
1β | mineral any | FMM_7_504.JPG | 542 x 592 | 520px | 95.94% | 555px | 93.75% | 52.43% |
2β | mineral any | FMM_1_5507.JPG | 518 x 732 | 497px | 95.95% | 667px | 91.12% | 20.44% |
3β | mineral any | FMM_1_87323.JPG | 595 x 490 | 529px | 88.91% | 321px | 65.51% | 36.08% |
4β | mineral any | FMM_7_6588.JPG | 537 x 591 | 518px | 96.46% | 345px | 58.38% | 39.31% |
5β | mineral any | FMM_1_8223.JPG | 508 x 807 | 498px | 98.03% | 756px | 93.68% | 52.8% |
6β | mineral any | FMM_1_93978.JPG | 415 x 671 | 407px | 98.07% | 662px | 98.66% | 73.06% |
7β | mineral any | FMM_4_5290.JPG | 210 x 489 | 174px | 82.86% | 251px | 51.33% | 33.53% |
8β | mineral any | FMM_1_94854.JPG | 382 x 563 | 367px | 96.07% | 547px | 97.16% | 65.03% |
9β | mineral any | FMM_7_5717.JPG | 567 x 870 | 512px | 90.3% | 605px | 69.54% | 11.81% |
10β | mineral any | FMM_1_86636.JPG | 520 x 592 | 511px | 98.27% | 587px | 99.16% | 73.62% |
License #
Citation #
If you make use of the MineralImage5k data, please cite the following reference:
@dataset{MineralImage5k,
author={Sergey Nesteruk and Julia Agafonova and Igor Pavlov and Maxim Gerasimov and Nikolay Latyshev and Denis Dimitrov and Andrey Kuznetsov and Artur Kadurin and Pavel Plechov},
title={MineralImage5k: A Benchmark for Zero-Shot Raw Mineral Visual Recognition and Description},
year={2023},
url={https://github.com/ai-forever/mineral-recognition}
}
If you are happy with Dataset Ninja and use provided visualizations and tools in your work, please cite us:
@misc{ visualization-tools-for-mineral-image-5k-dataset,
title = { Visualization Tools for MineralImage5k Dataset },
type = { Computer Vision Tools },
author = { Dataset Ninja },
howpublished = { \url{ https://datasetninja.com/mineral-image-5k } },
url = { https://datasetninja.com/mineral-image-5k },
journal = { Dataset Ninja },
publisher = { Dataset Ninja },
year = { 2025 },
month = { jan },
note = { visited on 2025-01-15 },
}
Download #
Dataset MineralImage5k can be downloaded in Supervisely format:
As an alternative, it can be downloaded with dataset-tools package:
pip install --upgrade dataset-tools
β¦ using following python code:
import dataset_tools as dtools
dtools.download(dataset='MineralImage5k', dst_dir='~/dataset-ninja/')
Make sure not to overlook the python code example available on the Supervisely Developer Portal. It will give you a clear idea of how to effortlessly work with the downloaded dataset.
The data in original format can be downloaded here.
Disclaimer #
Our gal from the legal dep told us we need to post this:
Dataset Ninja provides visualizations and statistics for some datasets that can be found online and can be downloaded by general audience. Dataset Ninja is not a dataset hosting platform and can only be used for informational purposes. The platform does not claim any rights for the original content, including images, videos, annotations and descriptions. Joint publishing is prohibited.
You take full responsibility when you use datasets presented at Dataset Ninja, as well as other information, including visualizations and statistics we provide. You are in charge of compliance with any dataset license and all other permissions. You are required to navigate datasets homepage and make sure that you can use it. In case of any questions, get in touch with us at hello@datasetninja.com.