Introduction #
The authors create the METU-ALET: A Dataset for Tool Detection in the Wild for detecting farming, gardening, office, stonemasonry, vehicle, woodworking, and workshop tools. The scenes in the dataset are snapshots of sophisticated environments with or without humans using the tools. The scenes the authors consider introduce several challenges for object detection, including the small scale of the tools, their articulated nature, occlusion, inter-class invariance, etc.
Motivation
In the foreseeable future, robots and humans are anticipated to coexist and collaborate on tasks that are particularly challenging, exhausting, or ergonomically unfavorable for humans. This collaboration necessitates robots to possess capabilities for perceiving humans, tasks, and environments. A crucial aspect of this perceptual ability is the detection of objects, particularly tools. Regrettably, the robotics community has largely overlooked the significance of human-utilized tools. While some studies have delved into tool affordances or their detection and transfer, these investigations have primarily been conducted in isolated and constrained environments. Additionally, they have typically examined only a limited array of tools. Compounding this oversight, existing literature lacks exploration into tool detection, and there is currently no available dataset for this purpose.
Dataset description
The authors focus on the detection of tools in realistic, cluttered environments where collaboration between humans and robots is expected. To be more specific, they study detection of tools in real work environments that are composed of many objects (tools) that look alike and that occlude each other. For this end, the authors first collect an extensive tool detection dataset composed of 49 tool categories. Then, they compare the widely used state-of-the-art object detectors on their dataset, as a baseline. The results suggest that detecting tools is very challenging owing to tools being too small and articulated, and bearing too much
inter-class similarity.
Samples from the METU-ALET dataset, illustrating the wide range of challenging scenes and tools that a robot is expected to recognize in a clutter, possible with human co-workers using the tools. Since annotations are too dense, only a small subset is displayed.
The need for a dedicated dataset for tool detection arises from several distinct challenges:
- Size Variation: Many tools are small objects, presenting a challenge for standard object detectors optimized for detecting larger objects.
- Articulation: A significant number of tools are articulated, introducing additional complexities such as changes in viewpoint, scale, and illumination. Object detectors must accommodate these variations.
- Cluttered Environments: Tools are commonly used in environments with high clutter, leading to challenges related to clutter, occlusion, appearance, and illumination.
- Low Inter-Class Differences: Some tools exhibit minimal differences between classes, making it difficult for detectors to distinguish between similar objects, such as screwdrivers, chisels, and files, or putty knives and scrapers.
In ALET(Automated Labeling of Equipment and Tools), the authors explore a range of 49 distinct tools categorized across six broad contexts or purposes: farming, gardening, office supplies, stonemasonry, vehicle maintenance, woodworking, and workshop tools. Notably, the dataset comprises the 20 most frequently occurring tools, including chisels, clamps, drills, files, gloves, hammers, mallets, meters, pens, pencils, planes, pliers, safety glasses, safety helmets, saws, screwdrivers, spades, tapes, trowels, and wrenches. Excluded from consideration are tools typically used in kitchen settings, as dedicated datasets already exist for this purpose. Additionally, the authors limited their focus to tools that can be readily grasped, pushed, or manipulated by a robot. Consequently, larger tools such as ladders, forklifts, and power tools exceeding the size of a handheld drill were omitted from the study.
The dataset comprises three distinct sets of images:
- Web-collected Images: The authors conducted web searches using specific keywords and usage descriptions, gathering royalty-free images from various online platforms including Creativecommons, Wikicommons, Flickr, Pexels, Unsplash, Shopify, Pixabay, Everystock, and Imfree.
- Author-Photographed Images: Additionally, the authors captured photographs of office and workshop environments on their campus.
- Synthetic Images: To ensure a minimum of 200 instances for each tool, the authors created synthetic images. They achieved this by developing a simulation environment using the Unity3D platform and incorporating 3D models of the tools.
Some examples from the Synthetic Images.
For each scene to be generated, the following steps were followed:
Scene Setup: The authors constructed an environment resembling a room, featuring four walls and 10 assorted objects like chairs, sofas, corner pieces, and televisions placed in fixed positions. At the room’s center, they introduced one of six different tables selected randomly from a range of 1 to 6. To enhance variability, they also scattered unrelated objects like mugs and bottles randomly throughout the scene.
Camera Configuration: The camera’s position in each dimension (x, y, z) was determined by random sampling from a uniform distribution ranging from -3 to 3. The camera’s viewing direction was oriented towards the center of the top surface of the table.
Tool Placement: For each scene, the authors randomly spawned a variable number of tools, N, ranging from 5 to 20, chosen randomly from a pool of 49 options. These tools were dropped onto the table from positions [x, y, z] randomly selected from a uniform distribution ranging from 0 to 1 above the table surface. The initial orientation of each tool, along each dimension, was sampled uniformly from 0 to 360 degrees.
For annotating the tools in the downloaded and the photographed images, the authors used the VGG Image Annotation (VIA) tool.
Dataset statistics
The dataset includes 22,835 bounding boxes (BBs). For each tool category, there are more than 200 BBs, which is on an order similar to the widely used object detection datasets such as PASCAL. As shown in Table II, METUALET includes tools that appear small (area < 322), medium (322 < area < 962 ) and large (962 < area) – following the naming convention from MS-COCO.
Subset | Small BBs | Medium BBs | Large BBs | Total |
---|---|---|---|---|
Downloaded | 809 | 4650 | 5661 | 11114 |
Photographed | 13 | 309 | 443 | 765 |
Synthesized | 813 | 6934 | 3209 | 10956 |
Total | 1629 | 11893 | 9313 | 22835 |
The sizes of the bounding boxes (BB) of the annotated tools in the dataset.
The dataset is composed of 2699 images in total, and on average, has size 1138 × 903. Although the number of images may appear low, the number of bounding boxes (22835) is sufficient since there are more than 200 BBs per tools, and the avg. number of BBs per image is rather large (6.6).
Subset | Cardinality | Avg. Resolution |
---|---|---|
Downloaded | 1870 | 924 × 786 |
Photographed | 89 | 3663 × 3310 |
Synthesized | 740 | 1374 × 917 |
Total/Avg | 2699 | 1138 × 903 |
The cardinality and the resolution of the images in the dataset.
Summary #
METU-ALET: A Dataset for Tool Detection in the Wild is a dataset for object detection and identification tasks. It is used in the robotics and safety industries.
The dataset consists of 2644 images with 22068 labeled objects belonging to 49 different classes including safety helmet, gloves, safety glass, and other: hammer, chisel, pliers, wrench, screwdriver, drill, mallet, saw, meter, pen, tape, safety headphones, spade, scissors, clamp, pencil, safety mask, trowel, bench vise, plane, aviation snip, grinder, ruler, file, brush, stapler, level, marker, ratchet, knife, square, rake, caulk gun, hex key, putty knife, tape dispenser, axe, staple gun, hole punch, crowbar, soldering iron, caliper, anvil, flashlight, riveter, and pencil sharpener.
Images in the METU-ALET dataset have bounding box annotations. There are 34 (1% of the total) unlabeled images (i.e. without annotations). There are 3 splits in the dataset: train (2112 images), val (268 images), and test (264 images). Alternatively, the dataset could be split into 5 sources: wikimgs (909 images), synthetic (740 images), other (438 images), flickr (416 images), and pixabay (141 images). The dataset was released in 2020 by the Middle East Technical University, Turkey.
Here is a visualized example for randomly selected sample classes:
Explore #
METU-ALET dataset has 2644 images. Click on one of the examples below or open "Explore" tool anytime you need to view dataset images with annotations. This tool has extended visualization capabilities like zoom, translation, objects table, custom filters and more. Hover the mouse over the images to hide or show annotations.
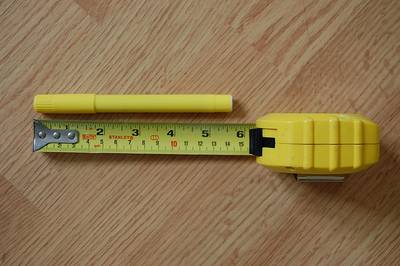
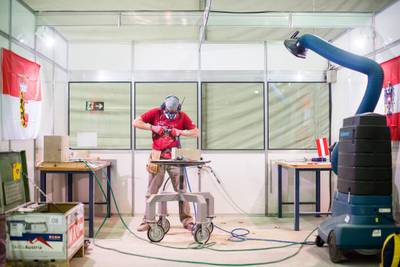


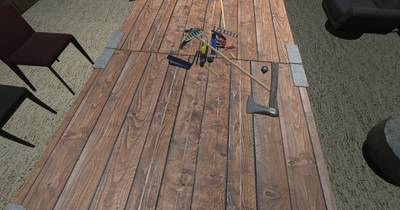
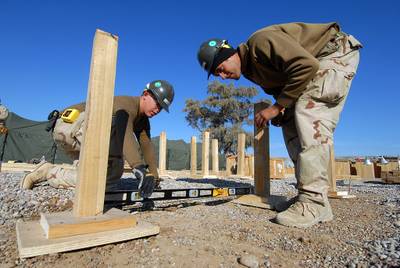
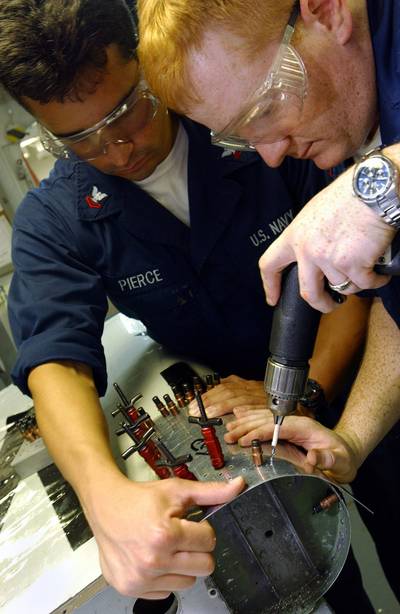
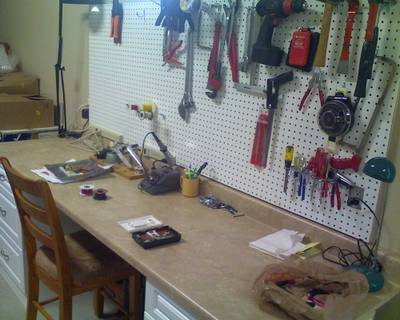
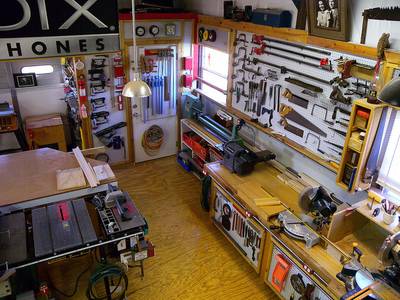
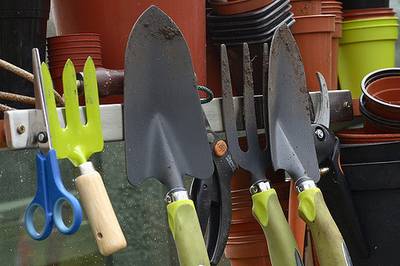
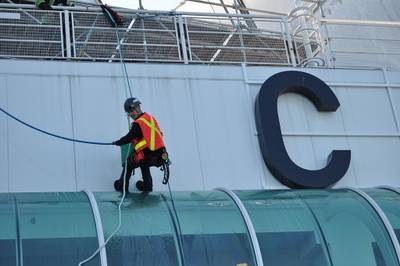
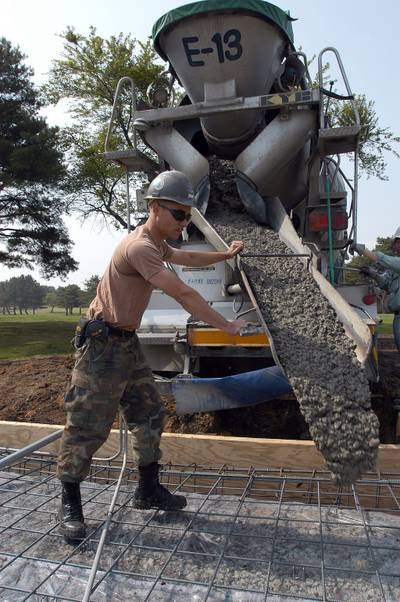
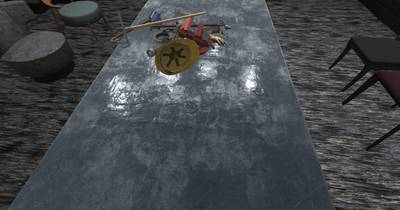
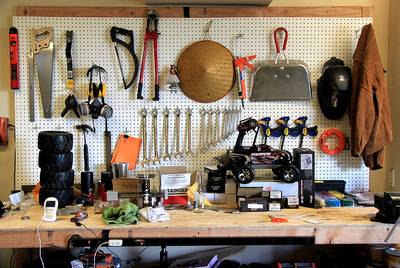
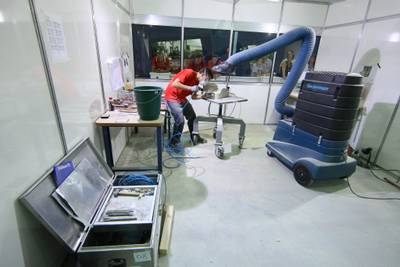
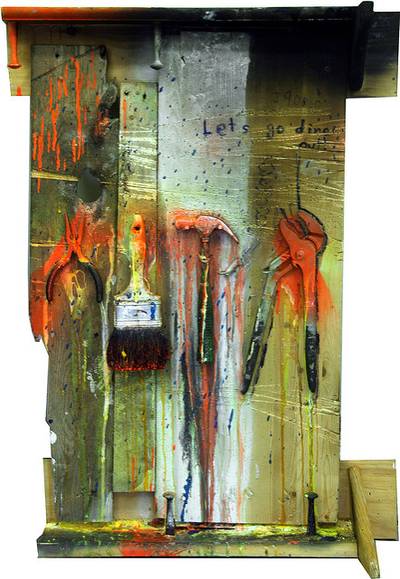
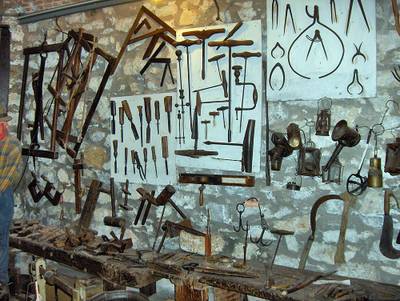
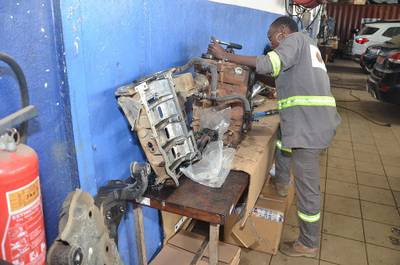
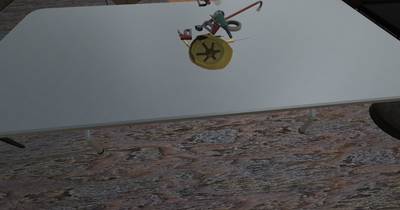
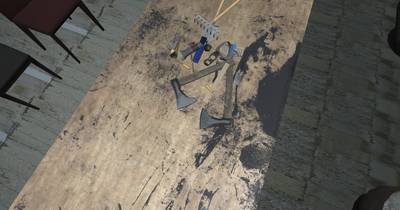
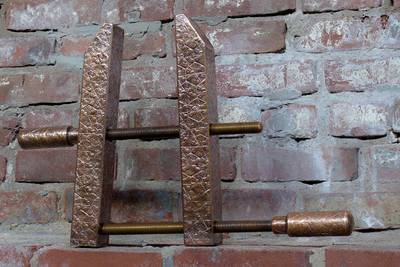

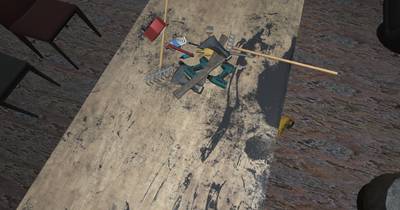
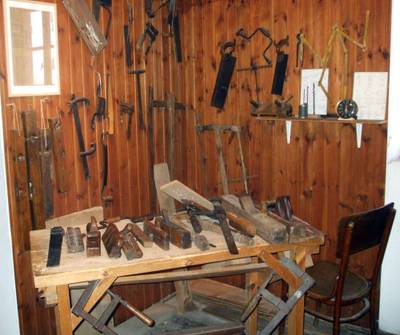
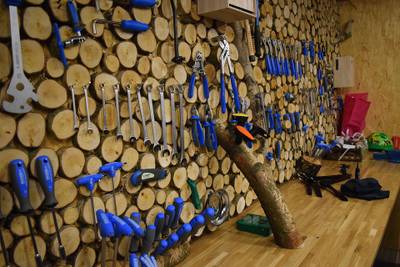
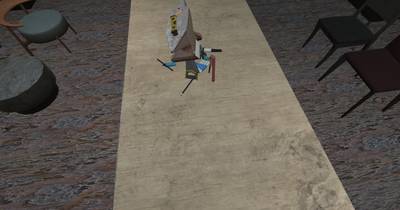


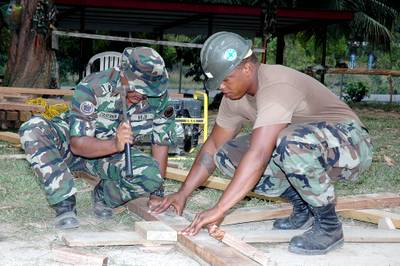
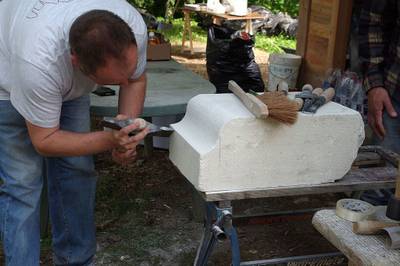
Class balance #
There are 49 annotation classes in the dataset. Find the general statistics and balances for every class in the table below. Click any row to preview images that have labels of the selected class. Sort by column to find the most rare or prevalent classes.
Class ㅤ | Images ㅤ | Objects ㅤ | Count on image average | Area on image average |
---|---|---|---|---|
safety helmet➔ rectangle | 617 | 1328 | 2.15 | 3.41% |
gloves➔ rectangle | 539 | 874 | 1.62 | 3.01% |
safety glass➔ rectangle | 530 | 665 | 1.25 | 1.23% |
hammer➔ rectangle | 524 | 734 | 1.4 | 5.38% |
chisel➔ rectangle | 470 | 1410 | 3 | 5.37% |
pliers➔ rectangle | 465 | 1037 | 2.23 | 8.09% |
wrench➔ rectangle | 406 | 1405 | 3.46 | 4.44% |
screwdriver➔ rectangle | 405 | 1032 | 2.55 | 2.75% |
drill➔ rectangle | 362 | 468 | 1.29 | 6.66% |
mallet➔ rectangle | 358 | 430 | 1.2 | 6.01% |
Images #
Explore every single image in the dataset with respect to the number of annotations of each class it has. Click a row to preview selected image. Sort by any column to find anomalies and edge cases. Use horizontal scroll if the table has many columns for a large number of classes in the dataset.
Object distribution #
Interactive heatmap chart for every class with object distribution shows how many images are in the dataset with a certain number of objects of a specific class. Users can click cell and see the list of all corresponding images.
Class sizes #
The table below gives various size properties of objects for every class. Click a row to see the image with annotations of the selected class. Sort columns to find classes with the smallest or largest objects or understand the size differences between classes.
Class | Object count | Avg area | Max area | Min area | Min height | Min height | Max height | Max height | Avg height | Avg height | Min width | Min width | Max width | Max width |
---|---|---|---|---|---|---|---|---|---|---|---|---|---|---|
chisel rectangle | 1410 | 3.1% | 91.51% | 0.03% | 5px | 0.78% | 1348px | 99.86% | 141px | 18.95% | 4px | 0.65% | 1260px | 97.2% |
wrench rectangle | 1405 | 1.44% | 71.85% | 0.01% | 6px | 0.66% | 1660px | 92.84% | 90px | 14.53% | 1px | 0.16% | 1332px | 94.92% |
safety helmet rectangle | 1328 | 1.6% | 40.06% | 0.02% | 9px | 0.85% | 673px | 69.48% | 98px | 11.02% | 12px | 1.17% | 650px | 68.44% |
pliers rectangle | 1037 | 3.8% | 90.35% | 0.02% | 3px | 0.28% | 3271px | 98.33% | 160px | 19.04% | 7px | 0.65% | 1646px | 96.78% |
screwdriver rectangle | 1032 | 1.25% | 31.41% | 0.02% | 9px | 1.13% | 1613px | 86.25% | 147px | 14.71% | 4px | 0.62% | 1765px | 84.69% |
gloves rectangle | 874 | 1.93% | 42.81% | 0.02% | 8px | 1.56% | 812px | 78.3% | 121px | 12.36% | 10px | 1.07% | 842px | 78.85% |
hammer rectangle | 734 | 4.02% | 80.61% | 0.08% | 11px | 1.72% | 1925px | 98.59% | 142px | 18.78% | 9px | 1.41% | 1042px | 98.34% |
safety glass rectangle | 665 | 0.98% | 17.63% | 0.03% | 11px | 0.91% | 592px | 43.34% | 93px | 8.88% | 13px | 1.46% | 662px | 53.81% |
pencil rectangle | 486 | 1.15% | 17.96% | 0.02% | 6px | 0.57% | 1272px | 86.6% | 129px | 16.07% | 4px | 0.6% | 1703px | 49.17% |
pen rectangle | 486 | 1.32% | 48.13% | 0.02% | 7px | 0.95% | 1554px | 92.78% | 118px | 10.88% | 4px | 0.45% | 1104px | 73.44% |
Spatial Heatmap #
The heatmaps below give the spatial distributions of all objects for every class. These visualizations provide insights into the most probable and rare object locations on the image. It helps analyze objects' placements in a dataset.
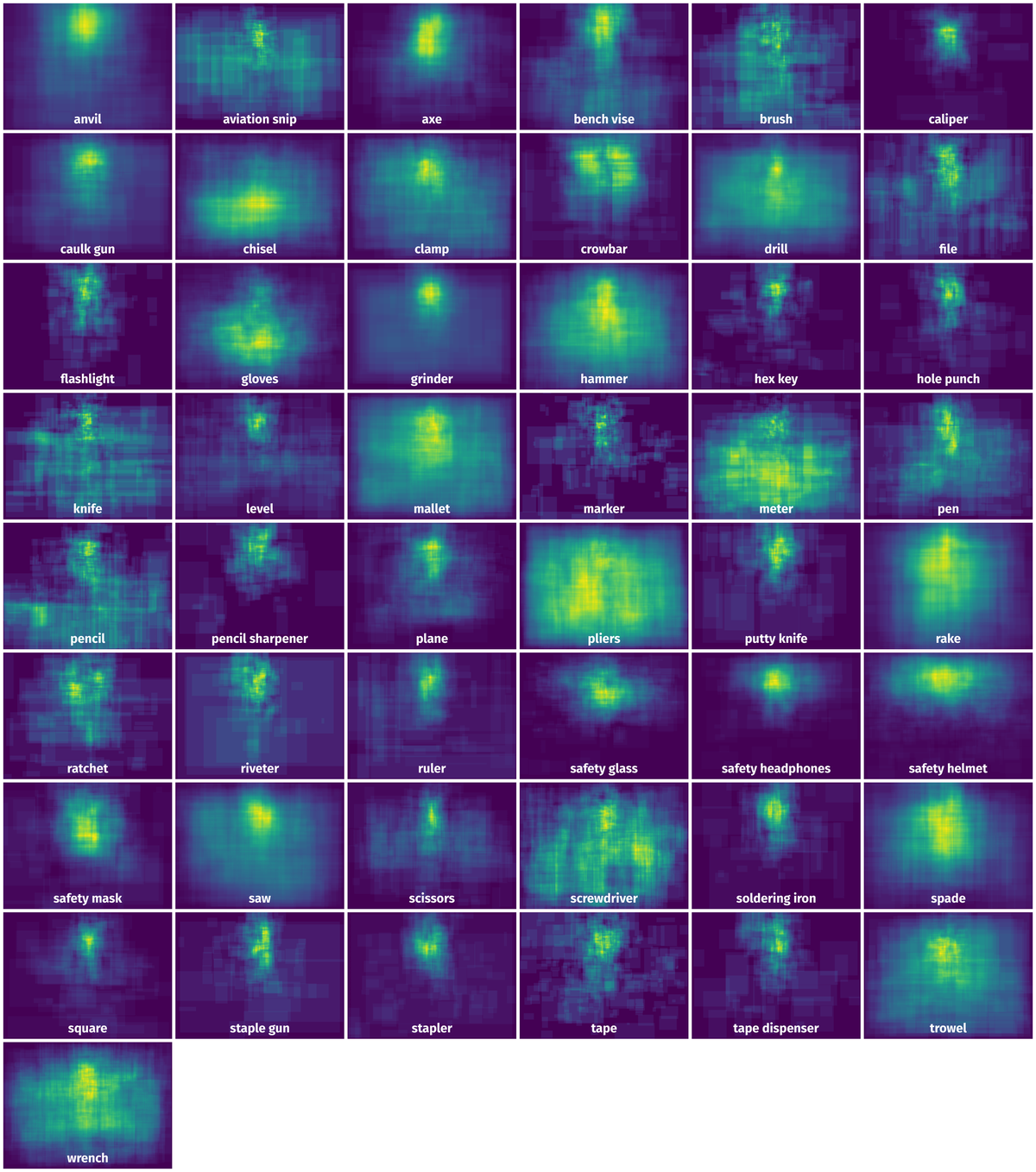
Objects #
Table contains all 22068 objects. Click a row to preview an image with annotations, and use search or pagination to navigate. Sort columns to find outliers in the dataset.
Object ID ㅤ | Class ㅤ | Image name click row to open | Image size height x width | Height ㅤ | Height ㅤ | Width ㅤ | Width ㅤ | Area ㅤ |
---|---|---|---|---|---|---|---|---|
1➔ | gloves rectangle | m_pixabay_woodworking8.jpg | 640 x 960 | 150px | 23.44% | 177px | 18.44% | 4.32% |
2➔ | safety helmet rectangle | wikimgs_construction644.jpg | 576 x 1024 | 69px | 11.98% | 70px | 6.84% | 0.82% |
3➔ | gloves rectangle | wikimgs_construction644.jpg | 576 x 1024 | 47px | 8.16% | 53px | 5.18% | 0.42% |
4➔ | gloves rectangle | wikimgs_construction644.jpg | 576 x 1024 | 46px | 7.99% | 54px | 5.27% | 0.42% |
5➔ | safety helmet rectangle | wikimgs_construction309.jpg | 775 x 1024 | 117px | 15.1% | 157px | 15.33% | 2.31% |
6➔ | safety helmet rectangle | wikimgs_construction19.jpg | 1536 x 1024 | 50px | 3.26% | 60px | 5.86% | 0.19% |
7➔ | gloves rectangle | wikimgs_construction19.jpg | 1536 x 1024 | 40px | 2.6% | 47px | 4.59% | 0.12% |
8➔ | trowel rectangle | wikimgs_construction44.jpg | 1365 x 1024 | 37px | 2.71% | 34px | 3.32% | 0.09% |
9➔ | mallet rectangle | wikimgs_construction44.jpg | 1365 x 1024 | 77px | 5.64% | 80px | 7.81% | 0.44% |
10➔ | clamp rectangle | Image15.png | 1058 x 2014 | 182px | 17.2% | 142px | 7.05% | 1.21% |
License #
The METU-ALET dataset is copyright free for research and commercial purposes provided that suitable citation is provided (see below):
- The “downloaded” images are selected from copyright free images from Creativecommons, Wikicommons, Flickr, Pexels, Unsplash, Shopify, Pixabay, Everystock.
- Additions to the images (photographed and synthesized) and object annotations are provided copyright free as well.
Citation #
If you make use of the ALET data, please cite the following reference:
@inproceedings{METU_ALET,
title={ALET (Automated Labeling of Equipment and Tools): A Dataset for Tool Detection and Human Worker Safety Detection},
author={Kurnaz, Fatih Can and Hocaog̃lu, Burak and Y{\i}lmaz, Mert Kaan and S{\"u}lo, {\.I}dil and Kalkan, Sinan},
booktitle={European Conference on Computer Vision Workshop on Assistive Computer Vision and Robotics},
pages={371--386},
year={2020},
organization={Springer}
}
If you are happy with Dataset Ninja and use provided visualizations and tools in your work, please cite us:
@misc{ visualization-tools-for-alet-dataset,
title = { Visualization Tools for METU-ALET Dataset },
type = { Computer Vision Tools },
author = { Dataset Ninja },
howpublished = { \url{ https://datasetninja.com/alet } },
url = { https://datasetninja.com/alet },
journal = { Dataset Ninja },
publisher = { Dataset Ninja },
year = { 2025 },
month = { apr },
note = { visited on 2025-04-26 },
}
Download #
Dataset METU-ALET can be downloaded in Supervisely format:
As an alternative, it can be downloaded with dataset-tools package:
pip install --upgrade dataset-tools
… using following python code:
import dataset_tools as dtools
dtools.download(dataset='METU-ALET', dst_dir='~/dataset-ninja/')
Make sure not to overlook the python code example available on the Supervisely Developer Portal. It will give you a clear idea of how to effortlessly work with the downloaded dataset.
The data in original format can be downloaded here.
Disclaimer #
Our gal from the legal dep told us we need to post this:
Dataset Ninja provides visualizations and statistics for some datasets that can be found online and can be downloaded by general audience. Dataset Ninja is not a dataset hosting platform and can only be used for informational purposes. The platform does not claim any rights for the original content, including images, videos, annotations and descriptions. Joint publishing is prohibited.
You take full responsibility when you use datasets presented at Dataset Ninja, as well as other information, including visualizations and statistics we provide. You are in charge of compliance with any dataset license and all other permissions. You are required to navigate datasets homepage and make sure that you can use it. In case of any questions, get in touch with us at hello@datasetninja.com.