Introduction #
The authors of the BSData: Industrial Machine Tool Element Surface Defect Dataset have compiled a collection of 1,104 channel-3 images accompanied by 394 image annotations, all related to the pitting type of surface damage. They address the problem of the manual inspection of machine tool components and the labor-intensive end-of-line product checks in industrial settings. In order to facilitate the automation of classification processes and the development of robust machine learning-based classification and wear prognostics models, the present dataset is provided.
The Ball Screw Drives (BSD) represent fundamental components in the realm of machine tools, serving the purpose of converting rotary motion into linear motion to enable the movement of machine tool axes. BSDs, as a subset of machine tool components, are notably prone to wear and stand as a primary contributor to the unplanned breakdown of machine tools. These components find extensive application not only within the machine tool industry but also in other sectors, including power generation, medicine, automation, and robotics. Given the tribological nature of BSDs (Sommer, Heinz, Schöfer 2018), wear constitutes a critical tribological aspect. Drawing from the classical bathtub curve (Heise 2002) and insights from (Haberkern 1998), (Schopp 2009), and (Spohrer 2019), BSD failures can be attributed to one of three distinct failure modes:
The dataset is generated through experiments conducted on a test bench at the Institute of Production Science at the Karlsruhe Institute of Technology. Here is a visual representation of the test bench alongside the integrated camera systems:
The design of the test bench accommodates the simultaneous wear testing of up to five spindles. These spindles are arranged in a configuration analogous to the arrangement of dots on a standard six-sided die, with the central spindle being the primary spindle connected to the motor. The remaining four spindles are actuated by a chain drive mechanism linked to the central spindle, ensuring uniform operation across all spindles. The spindles employed are standard 32mm diameter spindles, without any specialized treatment or prestress. Each spindle is preloaded with 70% of the manufacturer-specified 𝐶𝑎 (axial load capacity), where the manufacturer’s stipulated value of 𝐶𝑎 ensures safe operation for 106 revolutions. In this case, the selected 𝐶𝑎 is 12kN. The camera system is programmed to capture an image every four hours in this experimental setup. Following each image capture, the spindle is rotated by an additional 22.5°, and an area measuring 150x150 pixels is automatically cropped from the larger image.
The annotations of the dataset have been created using the annotation tool labelme and are available in JSON format, thus allowing for conversion to VOC and COCO formats. All of the images in this dataset originate from two distinct types of BSDs and 27 wear developments wear_dev. One of the two BSD types referenced in this dataset is represented by 69 images with 55 different image sizes, encompassing clean and soiled conditions. The other BSD type is depicted in 325 images, and these images come in two distinct sizes. Importantly, these images depict the evolution of soiling over time since they have been captured continuously. Additionally, the dataset includes the aforementioned 27 pitting development sequences.
Summary #
BSData: Industrial Machine Tool Element Surface Defect Dataset is a dataset for instance segmentation, semantic segmentation, and object detection tasks. It is used in the surface defect detection domain.
The dataset consists of 394 images with 485 labeled objects belonging to 1 single class (pitting).
Images in the BSData dataset have pixel-level instance segmentation annotations. Due to the nature of the instance segmentation task, it can be automatically transformed into a semantic segmentation (only one mask for every class) or object detection (bounding boxes for every object) tasks. All images are labeled (i.e. with annotations). There are 2 splits in the dataset: train (324 images) and test (70 images). Alternatively, the dataset could be split into 2 occurring BSD types: type_2 (325 images) and type_1 (69 images). Also, it could be categorized by the 27 wear developments (wear_dev). The dataset was released in 2021 by the Karlsruhe Institute of Technology, Germany.
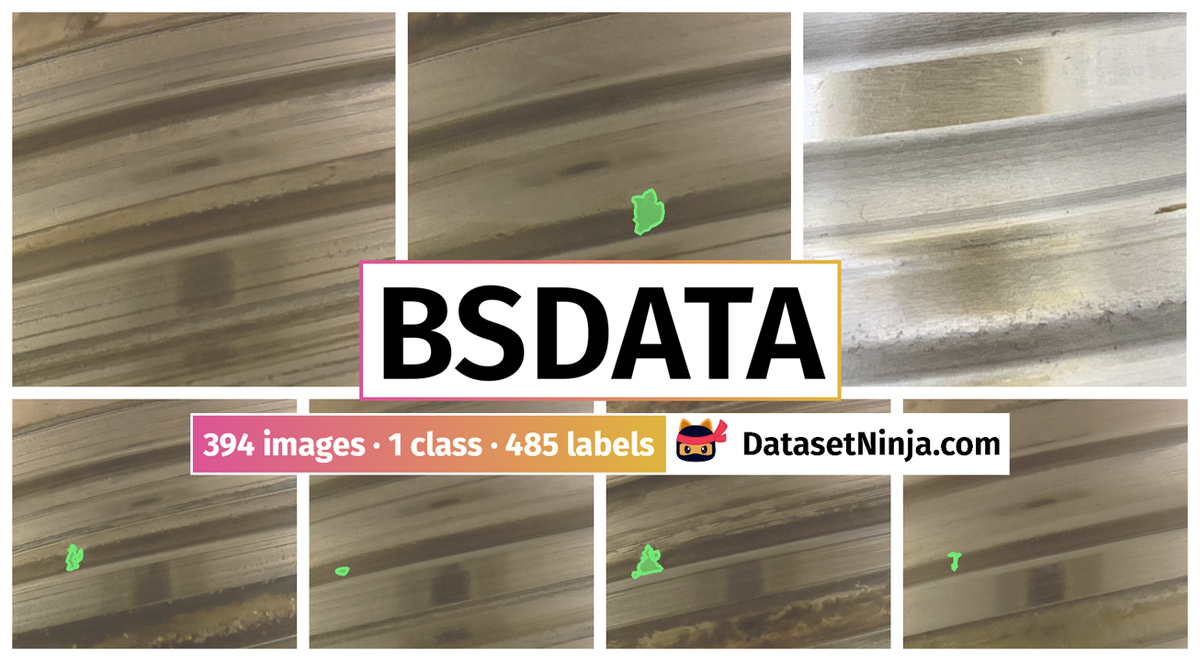
Explore #
BSData dataset has 394 images. Click on one of the examples below or open "Explore" tool anytime you need to view dataset images with annotations. This tool has extended visualization capabilities like zoom, translation, objects table, custom filters and more. Hover the mouse over the images to hide or show annotations.

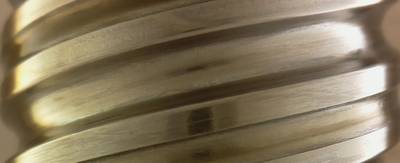
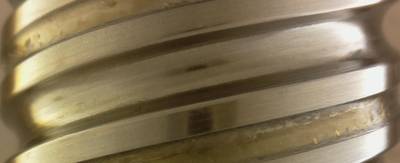
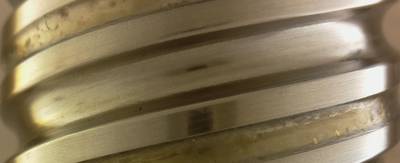
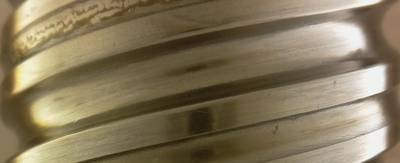
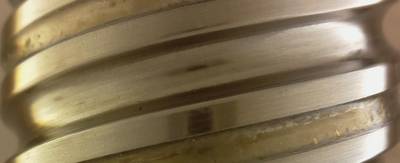
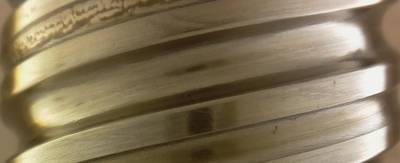
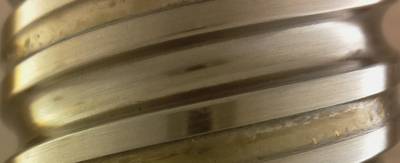

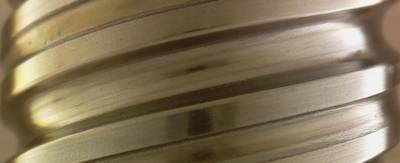
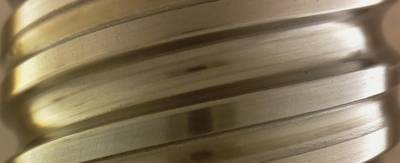
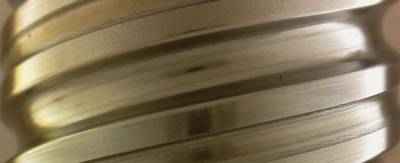
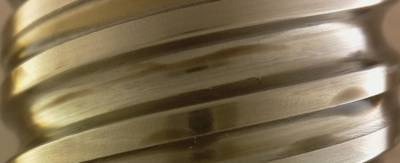
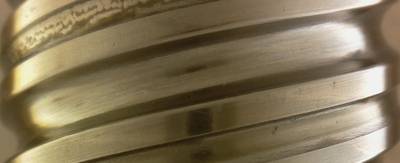
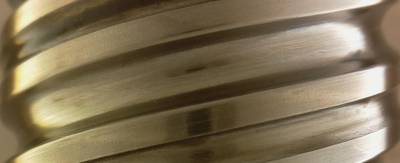
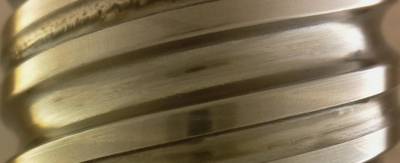

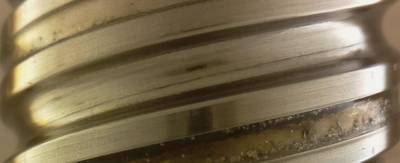
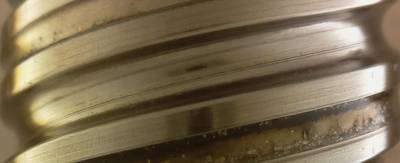
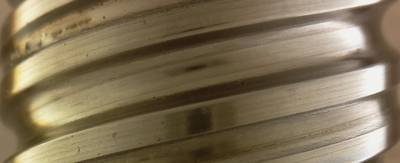
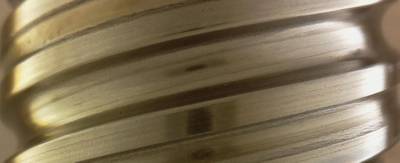
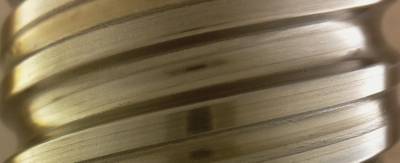

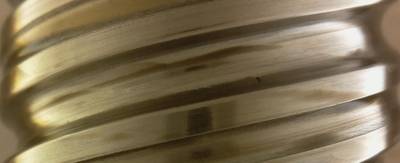

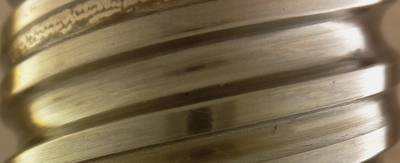
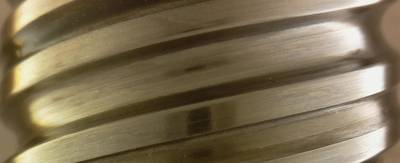
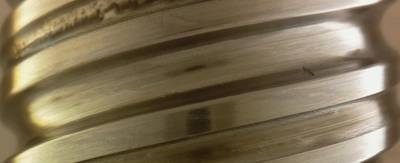
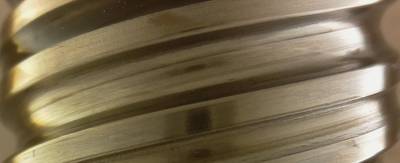

Class balance #
There are 1 annotation classes in the dataset. Find the general statistics and balances for every class in the table below. Click any row to preview images that have labels of the selected class. Sort by column to find the most rare or prevalent classes.
Class ㅤ | Images ㅤ | Objects ㅤ | Count on image average | Area on image average |
---|---|---|---|---|
pitting➔ polygon | 394 | 485 | 1.23 | 0.35% |
Images #
Explore every single image in the dataset with respect to the number of annotations of each class it has. Click a row to preview selected image. Sort by any column to find anomalies and edge cases. Use horizontal scroll if the table has many columns for a large number of classes in the dataset.
Object distribution #
Interactive heatmap chart for every class with object distribution shows how many images are in the dataset with a certain number of objects of a specific class. Users can click cell and see the list of all corresponding images.
Class sizes #
The table below gives various size properties of objects for every class. Click a row to see the image with annotations of the selected class. Sort columns to find classes with the smallest or largest objects or understand the size differences between classes.
Class | Object count | Avg area | Max area | Min area | Min height | Min height | Max height | Max height | Avg height | Avg height | Min width | Min width | Max width | Max width |
---|---|---|---|---|---|---|---|---|---|---|---|---|---|---|
pitting polygon | 485 | 0.27% | 1.09% | 0% | 6px | 1.04% | 108px | 16.74% | 56px | 9.11% | 4px | 0.22% | 198px | 12.86% |
Spatial Heatmap #
The heatmaps below give the spatial distributions of all objects for every class. These visualizations provide insights into the most probable and rare object locations on the image. It helps analyze objects' placements in a dataset.
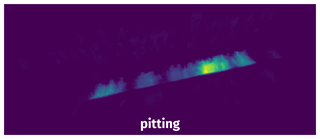
Objects #
Table contains all 485 objects. Click a row to preview an image with annotations, and use search or pagination to navigate. Sort columns to find outliers in the dataset.
Object ID ㅤ | Class ㅤ | Image name click row to open | Image size height x width | Height ㅤ | Height ㅤ | Width ㅤ | Width ㅤ | Area ㅤ |
---|---|---|---|---|---|---|---|---|
1➔ | pitting polygon | 01_200907165530_332500_225_crop_2.jpg | 460 x 1130 | 56px | 12.17% | 52px | 4.6% | 0.31% |
2➔ | pitting polygon | 01_200908111639_277500_315_crop_1.jpg | 645 x 1540 | 78px | 12.09% | 71px | 4.61% | 0.3% |
3➔ | pitting polygon | KGT1-S-131-A04_crop_0.jpg | 909 x 2642 | 47px | 5.17% | 52px | 1.97% | 0.04% |
4➔ | pitting polygon | 01_200907033550_585000_090_crop_2.jpg | 460 x 1130 | 54px | 11.74% | 91px | 8.05% | 0.56% |
5➔ | pitting polygon | 01_200906073637_635000_270_crop_2.jpg | 460 x 1130 | 28px | 6.09% | 35px | 3.1% | 0.06% |
6➔ | pitting polygon | 01_200908072550_635000_270_crop_1.jpg | 645 x 1540 | 96px | 14.88% | 128px | 8.31% | 0.65% |
7➔ | pitting polygon | 01_200908072550_635000_270_crop_1.jpg | 645 x 1540 | 90px | 13.95% | 121px | 7.86% | 0.57% |
8➔ | pitting polygon | 01_200908072550_635000_270_crop_1.jpg | 645 x 1540 | 41px | 6.36% | 71px | 4.61% | 0.09% |
9➔ | pitting polygon | 01_200907073132_397500_315_crop_2.jpg | 460 x 1130 | 54px | 11.74% | 69px | 6.11% | 0.38% |
10➔ | pitting polygon | 01_200907073551_585000_090_crop_2.jpg | 460 x 1130 | 57px | 12.39% | 102px | 9.03% | 0.59% |
License #
BSData: Dataset for Instance Segmentation and Industrial Wear Forecasting is under CC BY-SA 4.0 license.
Citation #
If you make use of the BSData data, please cite the following reference:
@dataset{BSData,
author={Tobias Schlagenhauf and Magnus Landwehr and Jürgen Fleischer},
title={BSData: Dataset for Instance Segmentation and Industrial Wear Forecasting},
year={2021},
url={https://github.com/2Obe/BSData}
}
If you are happy with Dataset Ninja and use provided visualizations and tools in your work, please cite us:
@misc{ visualization-tools-for-bsdata-dataset,
title = { Visualization Tools for BSData Dataset },
type = { Computer Vision Tools },
author = { Dataset Ninja },
howpublished = { \url{ https://datasetninja.com/bsdata } },
url = { https://datasetninja.com/bsdata },
journal = { Dataset Ninja },
publisher = { Dataset Ninja },
year = { 2025 },
month = { jul },
note = { visited on 2025-07-12 },
}
Download #
Dataset BSData can be downloaded in Supervisely format:
As an alternative, it can be downloaded with dataset-tools package:
pip install --upgrade dataset-tools
… using following python code:
import dataset_tools as dtools
dtools.download(dataset='BSData', dst_dir='~/dataset-ninja/')
Make sure not to overlook the python code example available on the Supervisely Developer Portal. It will give you a clear idea of how to effortlessly work with the downloaded dataset.
The data in original format can be downloaded here.
Disclaimer #
Our gal from the legal dep told us we need to post this:
Dataset Ninja provides visualizations and statistics for some datasets that can be found online and can be downloaded by general audience. Dataset Ninja is not a dataset hosting platform and can only be used for informational purposes. The platform does not claim any rights for the original content, including images, videos, annotations and descriptions. Joint publishing is prohibited.
You take full responsibility when you use datasets presented at Dataset Ninja, as well as other information, including visualizations and statistics we provide. You are in charge of compliance with any dataset license and all other permissions. You are required to navigate datasets homepage and make sure that you can use it. In case of any questions, get in touch with us at hello@datasetninja.com.