Introduction #
The CelebA: Large-Scale CelebFaces Attributes Dataset comprises over 200,000 celebrity images, each annotated with 40 attributes. The dataset encompasses diverse images with significant pose variations and background clutter. CelebA boasts extensive diversities, large quantities, and rich annotations, including 10,177 identities, 202,599 face images, 5 landmark locations, and 40 binary attribute annotations per image. It serves as valuable training and test sets for various computer vision tasks, such as face attribute recognition, face recognition, face detection, landmark localization, and face editing & synthesis.
The authors of the dataset address the challenging task of predicting face attributes in diverse settings marked by complex face variations. They introduce a novel deep learning framework designed for attribute prediction in such wild conditions. The framework involves two cascaded CNNs, namely LNet and ANet, both fine-tuned jointly with attribute tags but pre-trained using different strategies. LNet undergoes pre-training with extensive general object categories for face localization, while ANet is pre-trained with numerous face identities for attribute prediction.
Summary #
CelebA: Large-Scale CelebFaces Attributes Dataset is a dataset for object detection, classification, identification, and weakly supervised learning tasks. It is applicable or relevant across various domains.
The dataset consists of 202598 images with 1215588 labeled objects belonging to 6 different classes including face, left eye, right eye, and other: nose, left mouth, and right mouth.
Images in the CelebA dataset have bounding box annotations. All images are labeled (i.e. with annotations). There are 3 splits in the dataset: train (162771 images), test (19961 images), and val (19866 images). Additionally, every person has their own id tag. Alternatively, the dataset could be split into 40 attributes: no_beard (169157 images), young (156734 images), attractive (103833 images), mouth_slightly_open (97941 images), smiling (97668 images), wearing_lipstick (95715 images), high_cheekbones (92188 images), male (84434 images), heavy_makeup (78390 images), wavy_hair (64743 images), oval_face (57567 images), pointy_nose (56209 images), arched_eyebrows (54089 images), big_lips (48785 images), black_hair (48472 images), big_nose (47516 images), straight_hair (42222 images), brown_hair (41572 images), bags_under_eyes (41445 images), wearing_earrings (38275 images), bangs (30709 images), blond_hair (29983 images), bushy_eyebrows (28803 images), wearing_necklace (24913 images), narrow_eyes (23329 images), 5_o_clock_shadow (22516 images), receding_hairline (16163 images), wearing_necktie (14732 images), rosy_cheeks (13315 images), eyeglasses (13193 images), goatee (12716 images), chubby (11663 images), sideburns (11449 images), blurry (10312 images), wearing_hat (9817 images), double_chin (9459 images), pale_skin (8701 images), gray_hair (8499 images), mustache (8417 images), and bald (4547 images). The dataset was released in 2015 by the The Chinese University of Hong Kong.
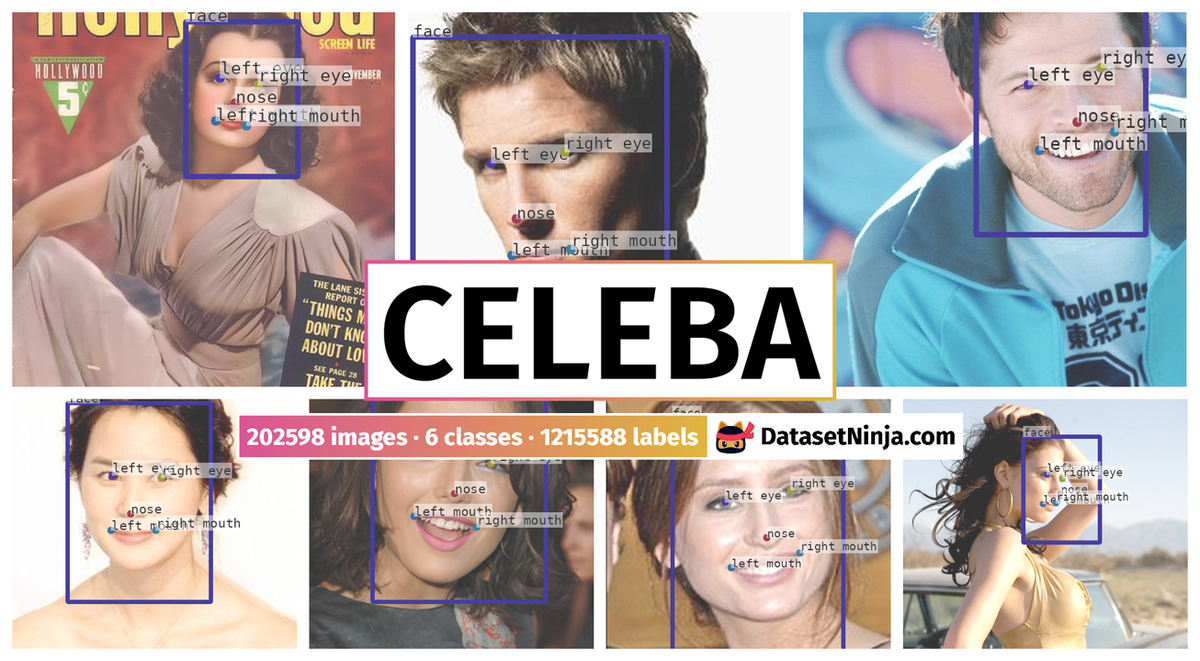
Explore #
CelebA dataset has 202598 images. Click on one of the examples below or open "Explore" tool anytime you need to view dataset images with annotations. This tool has extended visualization capabilities like zoom, translation, objects table, custom filters and more. Hover the mouse over the images to hide or show annotations.
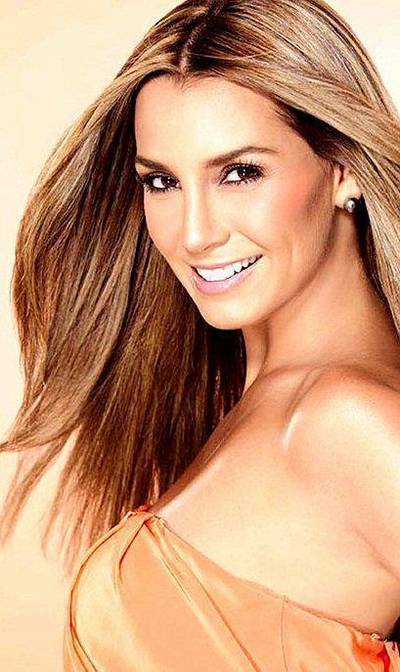
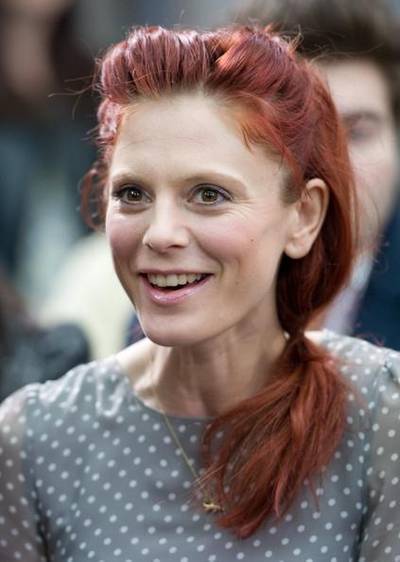
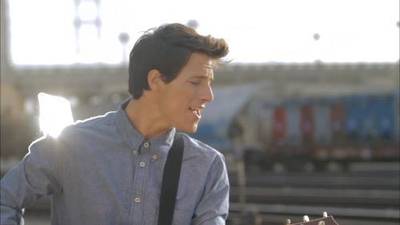
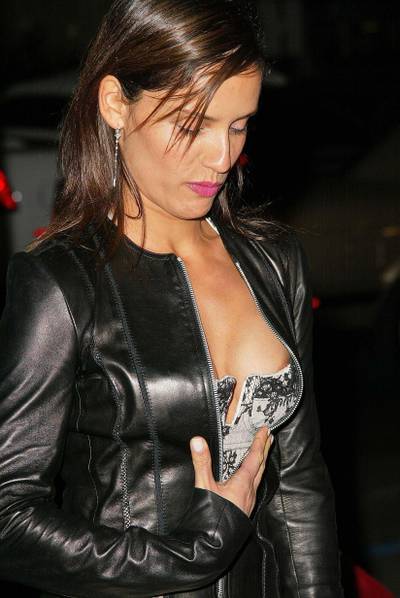
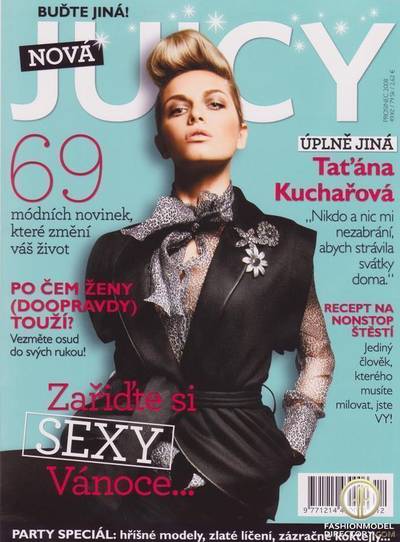
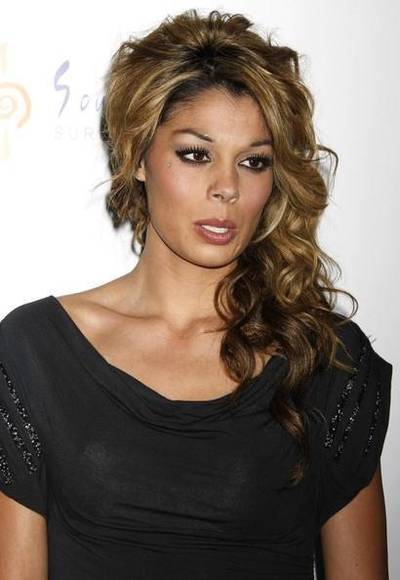
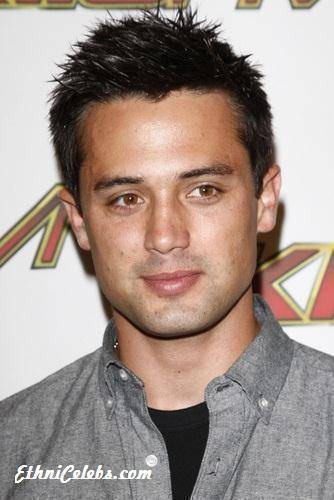
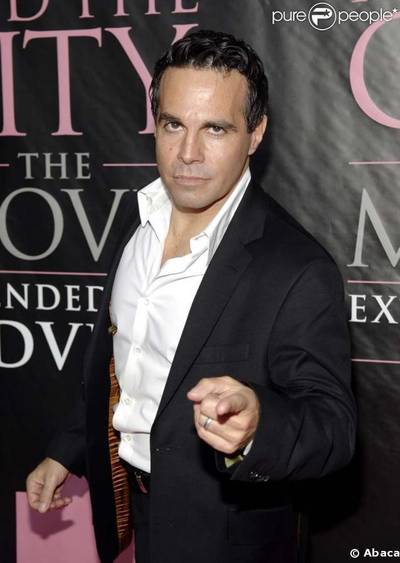
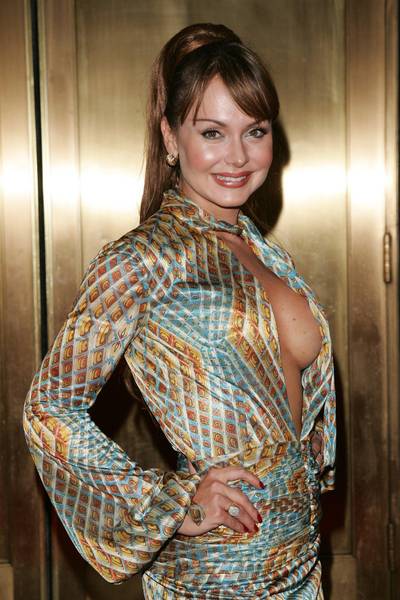
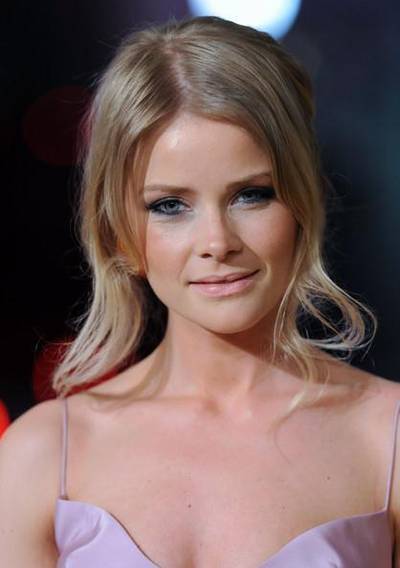
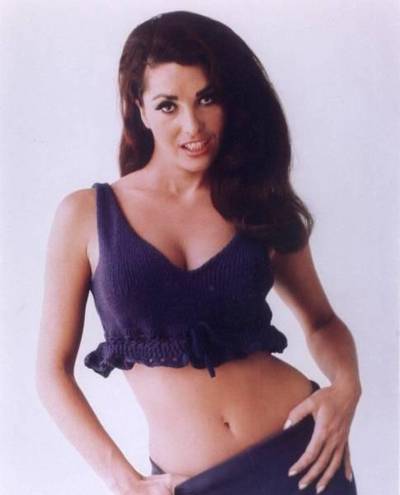
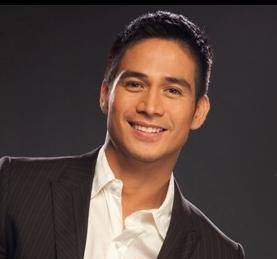
Class balance #
There are 6 annotation classes in the dataset. Find the general statistics and balances for every class in the table below. Click any row to preview images that have labels of the selected class. Sort by column to find the most rare or prevalent classes.
Class ã…¤ | Images ã…¤ | Objects ã…¤ | Count on image average | Area on image average |
---|---|---|---|---|
right mouthâž” point | 202598 | 202598 | 1 | 0% |
right eyeâž” point | 202598 | 202598 | 1 | 0% |
noseâž” point | 202598 | 202598 | 1 | 0% |
left mouthâž” point | 202598 | 202598 | 1 | 0% |
left eyeâž” point | 202598 | 202598 | 1 | 0% |
faceâž” rectangle | 202598 | 202598 | 1 | 23.21% |
Co-occurrence matrix #
Co-occurrence matrix is an extremely valuable tool that shows you the images for every pair of classes: how many images have objects of both classes at the same time. If you click any cell, you will see those images. We added the tooltip with an explanation for every cell for your convenience, just hover the mouse over a cell to preview the description.
Images #
Explore every single image in the dataset with respect to the number of annotations of each class it has. Click a row to preview selected image. Sort by any column to find anomalies and edge cases. Use horizontal scroll if the table has many columns for a large number of classes in the dataset.
Object distribution #
Interactive heatmap chart for every class with object distribution shows how many images are in the dataset with a certain number of objects of a specific class. Users can click cell and see the list of all corresponding images.
Class sizes #
The table below gives various size properties of objects for every class. Click a row to see the image with annotations of the selected class. Sort columns to find classes with the smallest or largest objects or understand the size differences between classes.
Class | Object count | Avg area | Max area | Min area | Min height | Min height | Max height | Max height | Avg height | Avg height | Min width | Min width | Max width | Max width |
---|---|---|---|---|---|---|---|---|---|---|---|---|---|---|
face rectangle | 202598 | 23.21% | 99.37% | 0% | 1px | 0.09% | 5300px | 99.84% | 270px | 48.88% | 1px | 0.05% | 3828px | 99.85% |
Spatial Heatmap #
The heatmaps below give the spatial distributions of all objects for every class. These visualizations provide insights into the most probable and rare object locations on the image. It helps analyze objects' placements in a dataset.
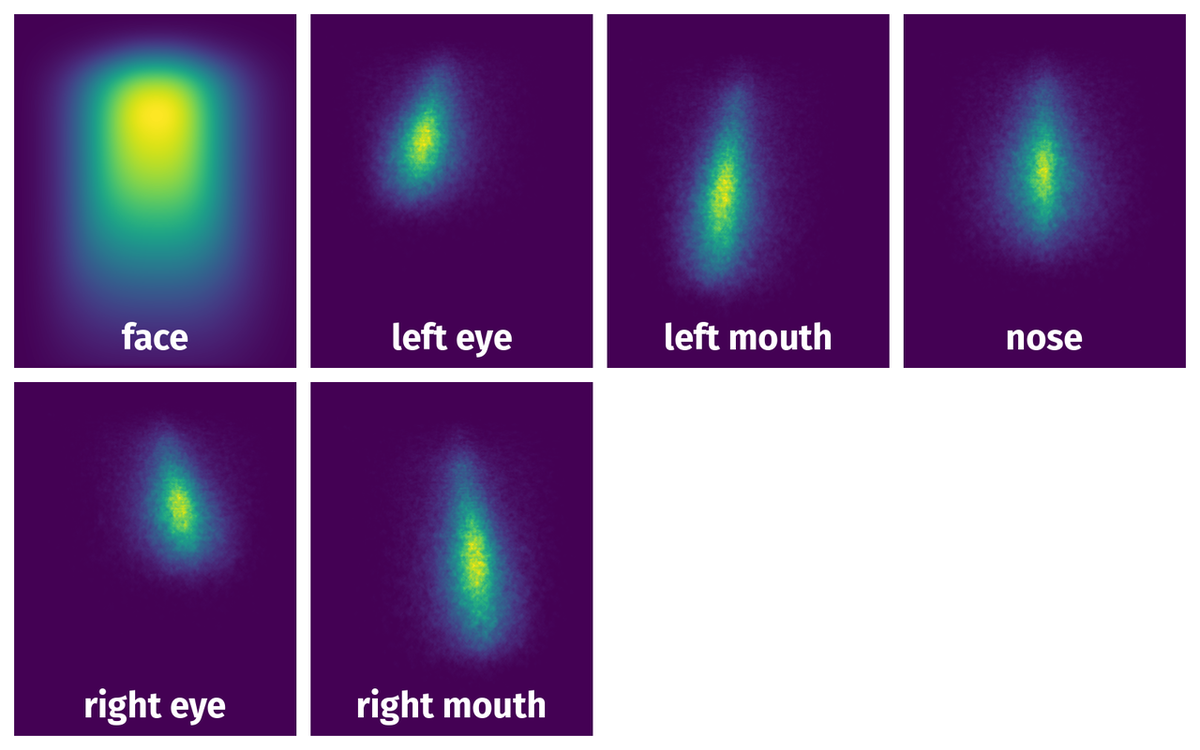
Objects #
Table contains all 16673 objects. Click a row to preview an image with annotations, and use search or pagination to navigate. Sort columns to find outliers in the dataset.
Object ID ã…¤ | Class ã…¤ | Image name click row to open | Image size height x width | Height ã…¤ | Height ã…¤ | Width ã…¤ | Width ã…¤ | Area ã…¤ |
---|---|---|---|---|---|---|---|---|
1âž” | face rectangle | 045474.jpg | 600 x 859 | 429px | 71.5% | 310px | 36.09% | 25.8% |
2âž” | face rectangle | 055700.jpg | 1131 x 700 | 285px | 25.2% | 206px | 29.43% | 7.42% |
3âž” | face rectangle | 071416.jpg | 1450 x 967 | 991px | 68.34% | 716px | 74.04% | 50.6% |
4âž” | face rectangle | 082745.jpg | 648 x 434 | 307px | 47.38% | 222px | 51.15% | 24.23% |
5âž” | face rectangle | 050229.jpg | 800 x 600 | 145px | 18.12% | 105px | 17.5% | 3.17% |
6âž” | face rectangle | 000783.jpg | 500 x 375 | 174px | 34.8% | 126px | 33.6% | 11.69% |
7âž” | face rectangle | 060527.jpg | 526 x 330 | 271px | 51.52% | 196px | 59.39% | 30.6% |
8âž” | face rectangle | 048470.jpg | 500 x 367 | 311px | 62.2% | 225px | 61.31% | 38.13% |
9âž” | face rectangle | 158864.jpg | 255 x 262 | 145px | 56.86% | 105px | 40.08% | 22.79% |
10âž” | face rectangle | 064529.jpg | 219 x 204 | 116px | 52.97% | 84px | 41.18% | 21.81% |
License #
- The CelebA dataset is available for non-commercial research purposes only.
- All images of the CelebA dataset are obtained from the Internet which are not property of MMLAB, The Chinese University of Hong Kong. The MMLAB is not responsible for the content nor the meaning of these images.
- You agree not to reproduce, duplicate, copy, sell, trade, resell or exploit for any commercial purposes, any portion of the images and any portion of derived data.
- You agree not to further copy, publish or distribute any portion of the CelebA dataset. Except, for internal use at a single site within the same organization it is allowed to make copies of the dataset.
- The MMLAB reserves the right to terminate your access to the CelebA dataset at any time.
- The face identities are released upon request for research purposes only. Please contact us for details.
Citation #
If you make use of the CelebA data, please cite the following reference:
@inproceedings{liu2015faceattributes,
title = {Deep Learning Face Attributes in the Wild},
author = {Liu, Ziwei and Luo, Ping and Wang, Xiaogang and Tang, Xiaoou},
booktitle = {Proceedings of International Conference on Computer Vision (ICCV)},
month = {December},
year = {2015}
}
If you are happy with Dataset Ninja and use provided visualizations and tools in your work, please cite us:
@misc{ visualization-tools-for-celeb-faces-attributes-dataset,
title = { Visualization Tools for CelebA Dataset },
type = { Computer Vision Tools },
author = { Dataset Ninja },
howpublished = { \url{ https://datasetninja.com/celeb-faces-attributes } },
url = { https://datasetninja.com/celeb-faces-attributes },
journal = { Dataset Ninja },
publisher = { Dataset Ninja },
year = { 2025 },
month = { jun },
note = { visited on 2025-06-25 },
}
Download #
Please visit dataset homepage to download the data.
Disclaimer #
Our gal from the legal dep told us we need to post this:
Dataset Ninja provides visualizations and statistics for some datasets that can be found online and can be downloaded by general audience. Dataset Ninja is not a dataset hosting platform and can only be used for informational purposes. The platform does not claim any rights for the original content, including images, videos, annotations and descriptions. Joint publishing is prohibited.
You take full responsibility when you use datasets presented at Dataset Ninja, as well as other information, including visualizations and statistics we provide. You are in charge of compliance with any dataset license and all other permissions. You are required to navigate datasets homepage and make sure that you can use it. In case of any questions, get in touch with us at hello@datasetninja.com.