Introduction #
The creators of the Cracks and Potholes in Road Images Dataset emphasize the critical influence of subpar road conditions on traffic safety. They highlight the practice of using vehicles for road data collection in different nations, where images are taken to guide highway maintenance and intervention decisions. However, these images frequently demand manual examination by skilled technicians to identify issues on the road.
They propose the use of image processing and machine learning techniques to automatically identify defects like cracks and potholes. However, to advance research in this domain, a substantial dataset is essential for algorithm training and recognition tests. To facilitate this, the authors have compiled a dataset featuring images of asphalt road defects in Brazil. This dataset serves as a foundation for studying crack and pothole detection using texture descriptors and machine learning algorithms such as Support Vector Machine, K-Nearest Neighbors, and Multi-Layer Perceptron Neural Network.
The authors sourced images from the Brazilian National Department of Transport Infrastructure (NDTI). These images pertain to highways in EspĂrito Santo, Rio Grande do Sul, and the Federal District. They manually selected 2235 images, applying criteria to ensure the absence of vehicles, people, and image defects. Each image in the dataset consists of three masks outlining the vehicleâs path, as well as crack and pothole defects.
The authors emphasize the value of this dataset, asserting its utility in training classifiers or neural networks to identify lanes, cracks, or potholes. They propose that various classifiers could be developed to suit specific problem types, contributing to road maintenance through defect recognition. They also highlight the potential of automating the detection and classification of defects in road images, which could expedite the monitoring process and reduce costs.
The authors underscore the significance of vision-based methods, explaining that they offer a cost-effective solution for defect detection using common cameras. The datasetâs focus on identifying cracks and potholes is pivotal for maintaining road safety and quality, informing intervention and maintenance strategies.
Detailing the dataset, the authors explain that it comprises images extracted from videos captured by NDTIâs Highway Diagnostic Vehicle (HDV). This vehicle is equipped with high-resolution cameras, including a top-mounted camera facing the front and two video cameras. The HDV captures images with minimum resolutions of 4 megapixels every 5 meters and records videos at 30 Frames Per Second (FPS) with a resolution of at least 1280x729.
The images were provided by the NDTI on a hard disk, and with the following characteristics:
- The images were captured between 2014 and 2017; and
- They are images from highways of EspĂrito Santo state (BR 101, 259, 262, 393, 447, 482 and 484), Rio Grande do Sul state (BR 101, 290 and 386) and Federal District (BR 010, 020, 060, 070, 080 and 251).
The dataset was developed using only the images provided by NDTI. A total of 2235 images were selected manually, considering the following criteria:
- To count as an image with damaged asphalt, present crack(s) and/or pothole(s);
- Do not contain vehicles in images;
- Do not contain people in images; and
- No problems due to capture, such as defects in colors (colors that do not correspond to the rest of the image) and defects in the image (such as missing parts).
In essence, the authorsâ dataset addresses the need for comprehensive road defect detection using advanced image processing and machine learning techniques, offering valuable resources for further research and practical applications in road maintenance.
Summary #
Cracks and Potholes in Road Images is a dataset for semantic segmentation and instance segmentation tasks. It is used in the utilities and safety industries.
The dataset consists of 2235 images with 4720 labeled objects belonging to 3 different classes including road, cracks, and pothole.
Images in the Cracks and Potholes in Road dataset have pixel-level semantic segmentation annotations. All images are labeled (i.e. with annotations). There are no pre-defined train/val/test splits in the dataset. The dataset was released in 2020 by the Universidade do Vale do Itajai, Brazil and DNIT, Brazil.
Here is the visualized example grid with animated annotations:
Explore #
Cracks and Potholes in Road dataset has 2235 images. Click on one of the examples below or open "Explore" tool anytime you need to view dataset images with annotations. This tool has extended visualization capabilities like zoom, translation, objects table, custom filters and more. Hover the mouse over the images to hide or show annotations.
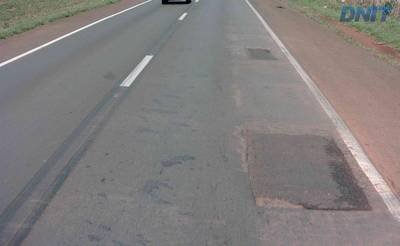
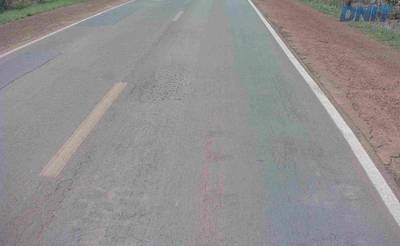
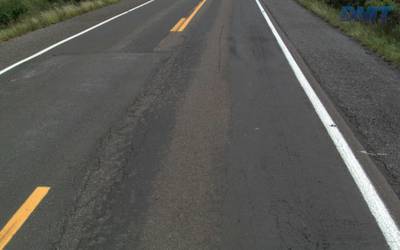
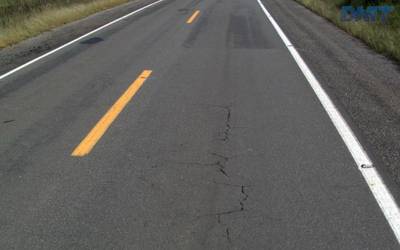
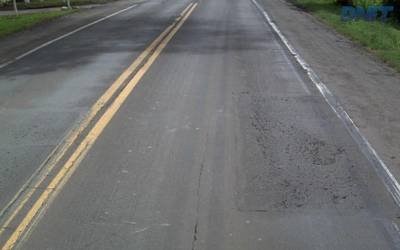
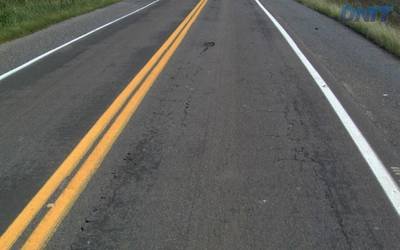
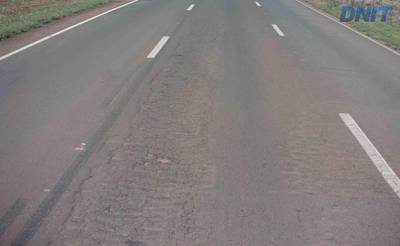
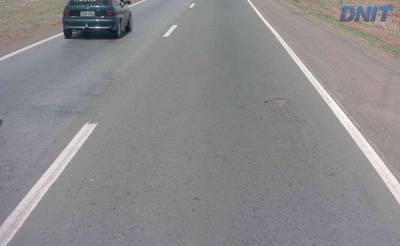

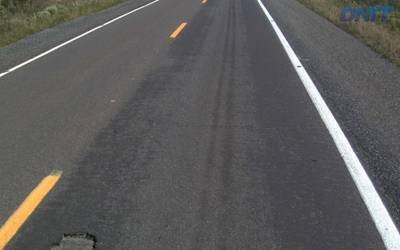
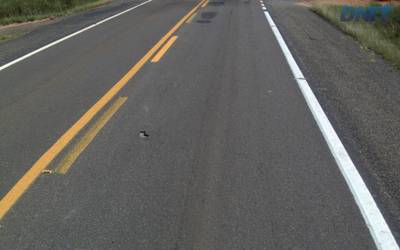

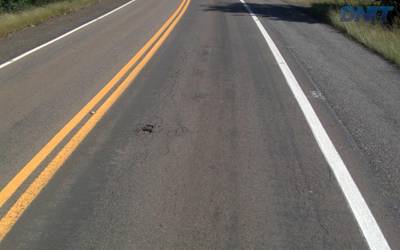
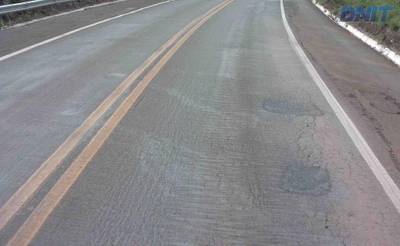
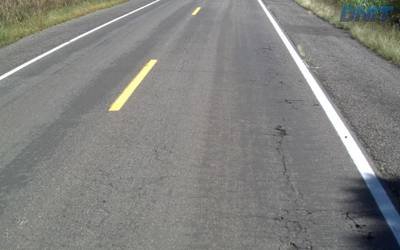
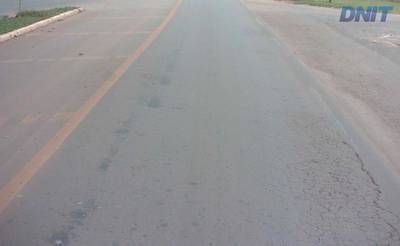
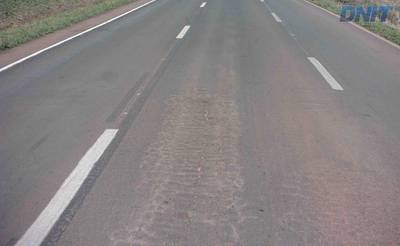
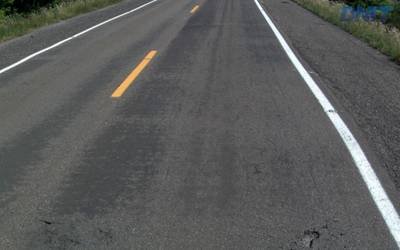
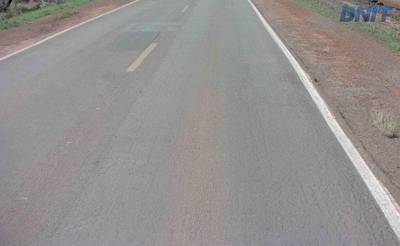
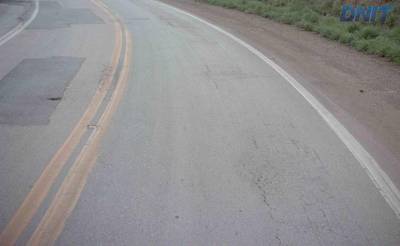
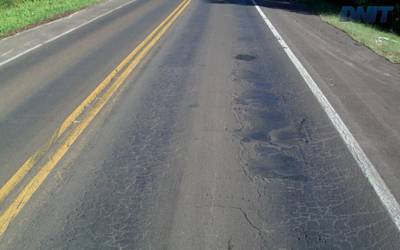
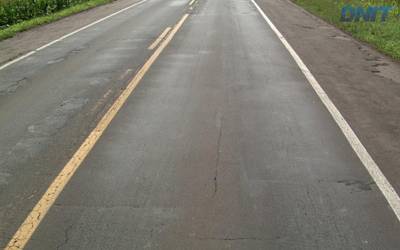
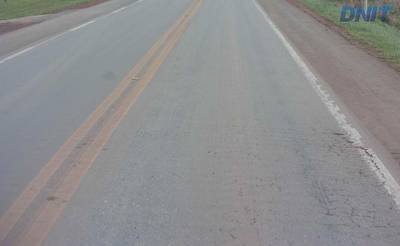
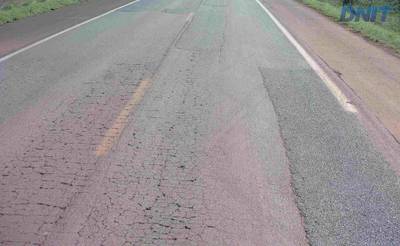
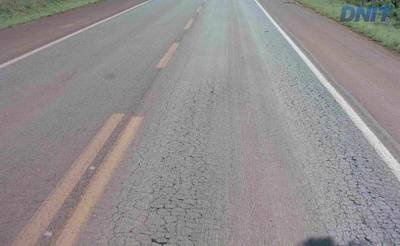
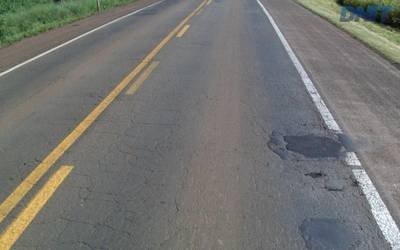
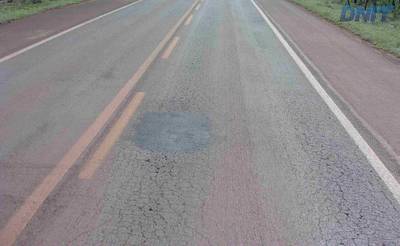
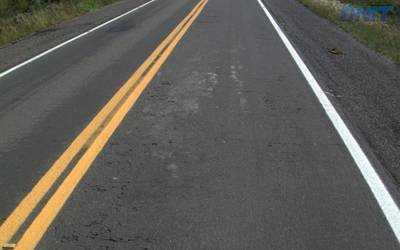
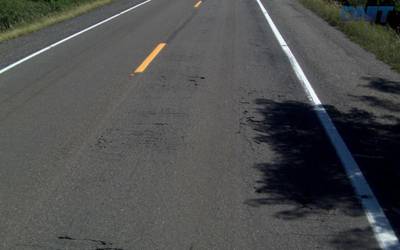
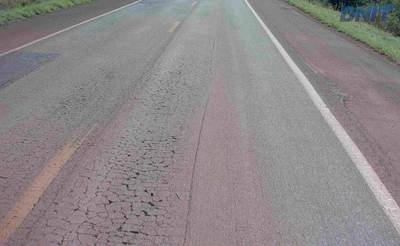
Class balance #
There are 3 annotation classes in the dataset. Find the general statistics and balances for every class in the table below. Click any row to preview images that have labels of the selected class. Sort by column to find the most rare or prevalent classes.
Class ă
€ | Images ă
€ | Objects ă
€ | Count on image average | Area on image average |
---|---|---|---|---|
roadâ mask | 2235 | 2235 | 1 | 55.68% |
cracksâ mask | 1921 | 1921 | 1 | 1.1% |
potholeâ mask | 564 | 564 | 1 | 1.62% |
Co-occurrence matrix #
Co-occurrence matrix is an extremely valuable tool that shows you the images for every pair of classes: how many images have objects of both classes at the same time. If you click any cell, you will see those images. We added the tooltip with an explanation for every cell for your convenience, just hover the mouse over a cell to preview the description.
Images #
Explore every single image in the dataset with respect to the number of annotations of each class it has. Click a row to preview selected image. Sort by any column to find anomalies and edge cases. Use horizontal scroll if the table has many columns for a large number of classes in the dataset.
Object distribution #
Interactive heatmap chart for every class with object distribution shows how many images are in the dataset with a certain number of objects of a specific class. Users can click cell and see the list of all corresponding images.
Class sizes #
The table below gives various size properties of objects for every class. Click a row to see the image with annotations of the selected class. Sort columns to find classes with the smallest or largest objects or understand the size differences between classes.
Class | Object count | Avg area | Max area | Min area | Min height | Min height | Max height | Max height | Avg height | Avg height | Min width | Min width | Max width | Max width |
---|---|---|---|---|---|---|---|---|---|---|---|---|---|---|
road mask | 2235 | 55.68% | 72.74% | 46.45% | 603px | 95.71% | 639px | 99.84% | 634px | 99.83% | 696px | 67.97% | 1023px | 99.9% |
cracks mask | 1921 | 1.1% | 8.63% | 0.01% | 11px | 1.72% | 623px | 97.34% | 351px | 55.23% | 13px | 1.27% | 998px | 97.46% |
pothole mask | 564 | 1.62% | 18.93% | 0.01% | 8px | 1.27% | 639px | 99.84% | 163px | 25.49% | 14px | 1.37% | 775px | 75.68% |
Spatial Heatmap #
The heatmaps below give the spatial distributions of all objects for every class. These visualizations provide insights into the most probable and rare object locations on the image. It helps analyze objects' placements in a dataset.
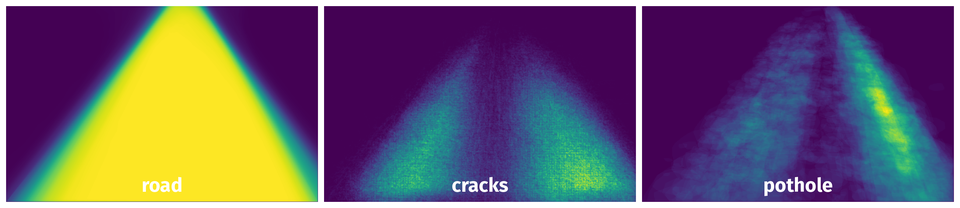
Objects #
Table contains all 4720 objects. Click a row to preview an image with annotations, and use search or pagination to navigate. Sort columns to find outliers in the dataset.
Object ID ă
€ | Class ă
€ | Image name click row to open | Image size height x width | Height ă
€ | Height ă
€ | Width ă
€ | Width ă
€ | Area ă
€ |
---|---|---|---|---|---|---|---|---|
1â | road mask | 1092965_DF_251_251BDF0052_00883_RAW.jpg | 630 x 1024 | 629px | 99.84% | 975px | 95.21% | 57.95% |
2â | cracks mask | 1092965_DF_251_251BDF0052_00883_RAW.jpg | 630 x 1024 | 301px | 47.78% | 274px | 26.76% | 1.2% |
3â | road mask | 1084307_DF_080_080BDF0090_01435_RAW.jpg | 630 x 1024 | 629px | 99.84% | 898px | 87.7% | 53.15% |
4â | cracks mask | 1084307_DF_080_080BDF0090_01435_RAW.jpg | 630 x 1024 | 173px | 27.46% | 856px | 83.59% | 0.38% |
5â | road mask | 1081495_DF_080_080BDF0070_00305_RAW.jpg | 630 x 1024 | 629px | 99.84% | 872px | 85.16% | 51.79% |
6â | cracks mask | 1081495_DF_080_080BDF0070_00305_RAW.jpg | 630 x 1024 | 428px | 67.94% | 832px | 81.25% | 2.1% |
7â | road mask | 941638_RS_290_290RS070691_01095_RAW.jpg | 640 x 1024 | 638px | 99.69% | 960px | 93.75% | 53.68% |
8â | pothole mask | 941638_RS_290_290RS070691_01095_RAW.jpg | 640 x 1024 | 118px | 18.44% | 102px | 9.96% | 0.8% |
9â | road mask | 1025898_RS_386_386RS169832_09230_RAW.jpg | 640 x 1024 | 639px | 99.84% | 1023px | 99.9% | 61.73% |
10â | cracks mask | 1025898_RS_386_386RS169832_09230_RAW.jpg | 640 x 1024 | 182px | 28.44% | 120px | 11.72% | 0.25% |
License #
Citation #
If you make use of the Cracks and Potholes in Road data, please cite the following reference:
Passos, Bianka T.; Cassaniga, Mateus J.; Fernandes, Anita M. R. ; Medeiros, KĂĄtya B. ; Comunello, Eros (2020), âCracks and Potholes in Road Imagesâ, Mendeley Data, V4, doi: 10.17632/t576ydh9v8.4
If you are happy with Dataset Ninja and use provided visualizations and tools in your work, please cite us:
@misc{ visualization-tools-for-cracks-and-potholes-in-road-dataset,
title = { Visualization Tools for Cracks and Potholes in Road Dataset },
type = { Computer Vision Tools },
author = { Dataset Ninja },
howpublished = { \url{ https://datasetninja.com/cracks-and-potholes-in-road } },
url = { https://datasetninja.com/cracks-and-potholes-in-road },
journal = { Dataset Ninja },
publisher = { Dataset Ninja },
year = { 2025 },
month = { jan },
note = { visited on 2025-01-22 },
}
Download #
Dataset Cracks and Potholes in Road can be downloaded in Supervisely format:
As an alternative, it can be downloaded with dataset-tools package:
pip install --upgrade dataset-tools
⊠using following python code:
import dataset_tools as dtools
dtools.download(dataset='Cracks and Potholes in Road', dst_dir='~/dataset-ninja/')
Make sure not to overlook the python code example available on the Supervisely Developer Portal. It will give you a clear idea of how to effortlessly work with the downloaded dataset.
The data in original format can be downloaded here.
Disclaimer #
Our gal from the legal dep told us we need to post this:
Dataset Ninja provides visualizations and statistics for some datasets that can be found online and can be downloaded by general audience. Dataset Ninja is not a dataset hosting platform and can only be used for informational purposes. The platform does not claim any rights for the original content, including images, videos, annotations and descriptions. Joint publishing is prohibited.
You take full responsibility when you use datasets presented at Dataset Ninja, as well as other information, including visualizations and statistics we provide. You are in charge of compliance with any dataset license and all other permissions. You are required to navigate datasets homepage and make sure that you can use it. In case of any questions, get in touch with us at hello@datasetninja.com.