Introduction #
Authors introduce DUTS, a significant contribution to the field of saliency detection, which originally relied on unsupervised computational models with heuristic priors but has recently seen remarkable progress with deep neural networks (DNNs). DUTS is a large-scale dataset comprising 10,553 train images and 5,019 test images. The training images are sourced from the ImageNet DET training/val sets, while the test images are drawn from the ImageNet DET test set and the SUN dataset, encompassing challenging scenarios for salient_object detection. What sets DUTS apart is its meticulously annotated pixel-level ground truths by 50 subjects and the explicit training/test evaluation protocol, making it the largest saliency detection benchmark to date, enabling fair and consistent comparisons in future research endeavors, with the training set serving as an ideal resource for DNN learning and the test set for evaluation purposes.

Image-level tags (left panel) provide informative cues of dominant objects, which tend to be the salient foreground. Authors propose to use image-level tags as weak supervision to learn to predict pixel-level saliency maps (right panel).
Authors provide a new paradigm for learning saliency detectors with weak supervision, which requires less annotation efforts and allows the usage of existing large scale data set with only imagelevel tags (e.g., ImageNet). Secondly, authors propose two novel network designs, i.e., global smooth pooling layer and foreground inference network, which enable the deep model to infer saliency maps by leveraging image-level tags and better generalize to previously unseen categories at test time. Thirdly, authors propose a new CRF algorithm, which provides accurate refinement of the estimated ground truth, giving rise to more effective network training. The trained DNN does not require any post-processing step, and yields comparable or even higher accuracy than fully supervised counterparts at a substantially accelerated speed.

Overview of the network architecture. In the first stage, authors jointly train FCN and FIN (b-e) for image categorization (f). In the second stage, the FIN (b,d) is trained for saliency prediction (g).
Summary #
DUTS is a dataset for semantic segmentation and weakly supervised learning tasks. It is applicable or relevant across various domains.
The dataset consists of 15572 images with 15572 labeled objects belonging to 1 single class (salient_object).
Images in the DUTS dataset have pixel-level semantic segmentation annotations. All images are labeled (i.e. with annotations). There are 2 splits in the dataset: train (10553 images) and test (5019 images). The dataset was released in 2018 by the Tiwaki Co., Ltd and Dalian University of Technology, China.
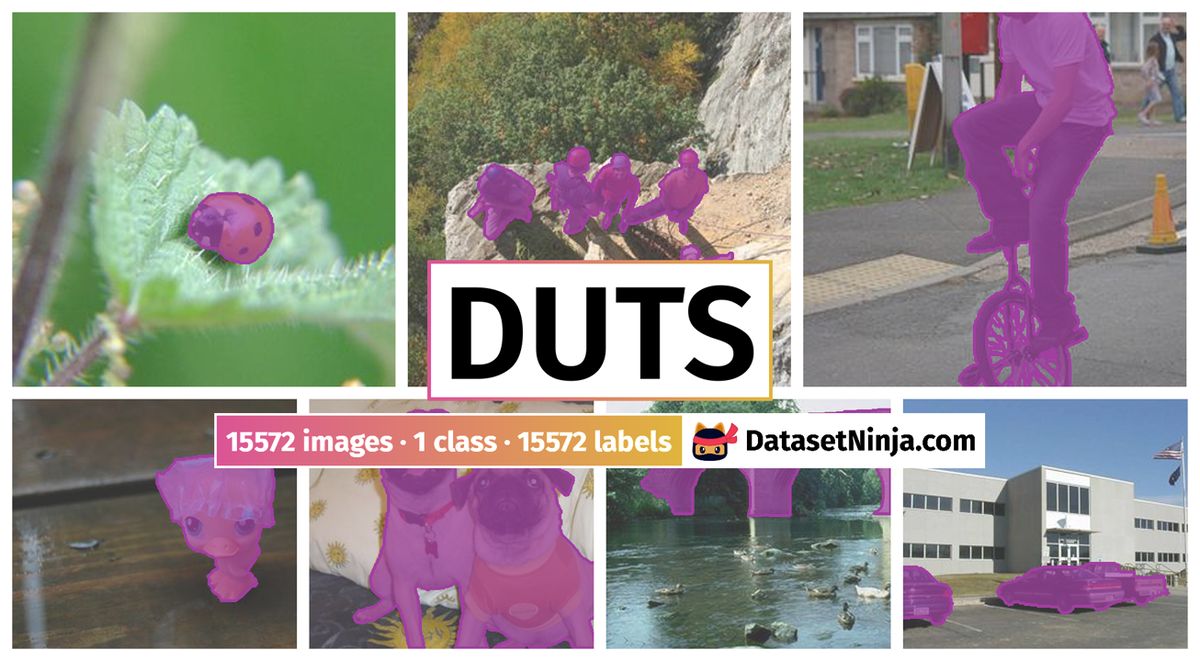
Explore #
DUTS dataset has 15572 images. Click on one of the examples below or open "Explore" tool anytime you need to view dataset images with annotations. This tool has extended visualization capabilities like zoom, translation, objects table, custom filters and more. Hover the mouse over the images to hide or show annotations.
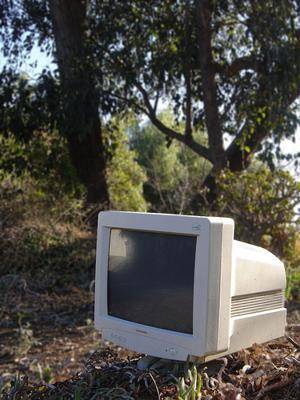
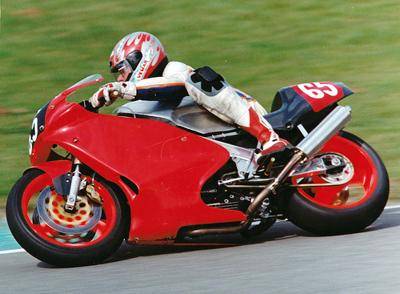
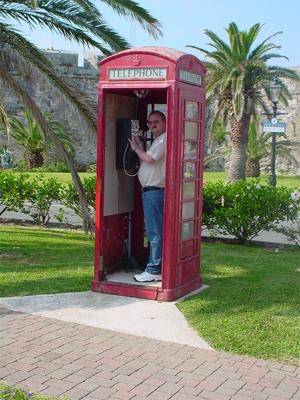
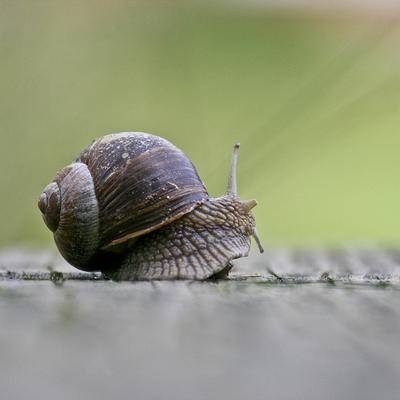
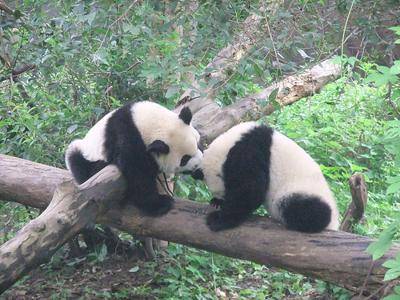
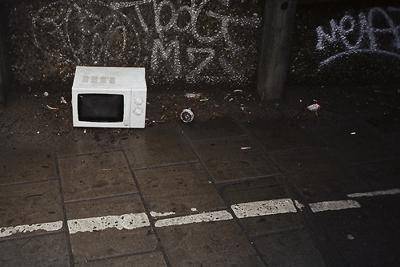
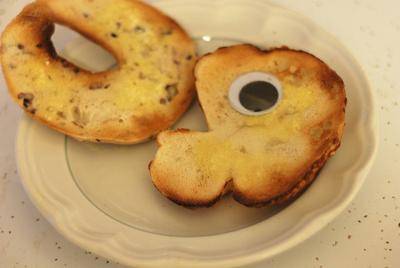
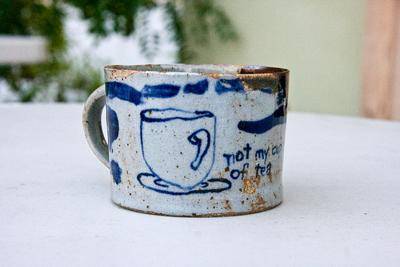
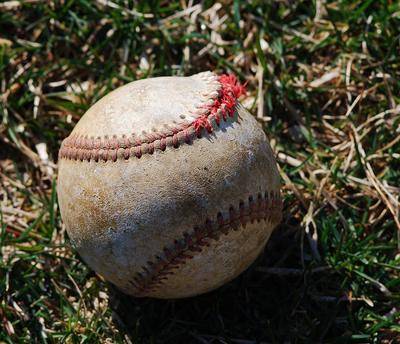
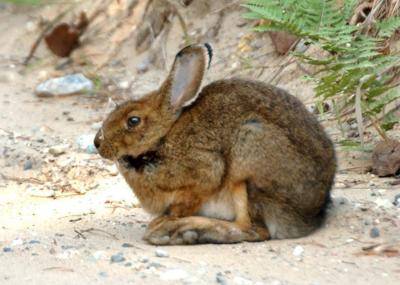
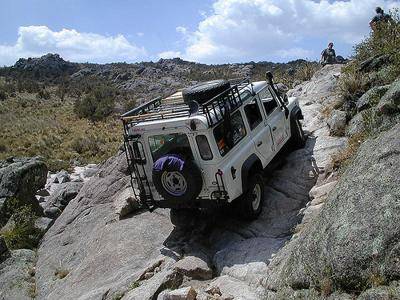
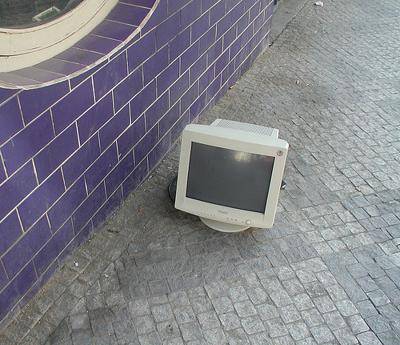
Class balance #
There are 1 annotation classes in the dataset. Find the general statistics and balances for every class in the table below. Click any row to preview images that have labels of the selected class. Sort by column to find the most rare or prevalent classes.
Class ã…¤ | Images ã…¤ | Objects ã…¤ | Count on image average | Area on image average |
---|---|---|---|---|
salient_objectâž” mask | 15572 | 15572 | 1 | 23.17% |
Images #
Explore every single image in the dataset with respect to the number of annotations of each class it has. Click a row to preview selected image. Sort by any column to find anomalies and edge cases. Use horizontal scroll if the table has many columns for a large number of classes in the dataset.
Object distribution #
Interactive heatmap chart for every class with object distribution shows how many images are in the dataset with a certain number of objects of a specific class. Users can click cell and see the list of all corresponding images.
Class sizes #
The table below gives various size properties of objects for every class. Click a row to see the image with annotations of the selected class. Sort columns to find classes with the smallest or largest objects or understand the size differences between classes.
Class | Object count | Avg area | Max area | Min area | Min height | Min height | Max height | Max height | Avg height | Avg height | Min width | Min width | Max width | Max width |
---|---|---|---|---|---|---|---|---|---|---|---|---|---|---|
salient_object mask | 15572 | 23.17% | 84.59% | 0.02% | 7px | 2.33% | 400px | 100% | 205px | 63.42% | 5px | 1.25% | 400px | 100% |
Spatial Heatmap #
The heatmaps below give the spatial distributions of all objects for every class. These visualizations provide insights into the most probable and rare object locations on the image. It helps analyze objects' placements in a dataset.
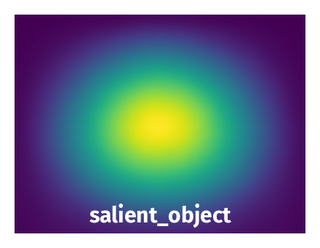
Objects #
Table contains all 15572 objects. Click a row to preview an image with annotations, and use search or pagination to navigate. Sort columns to find outliers in the dataset.
Object ID ã…¤ | Class ã…¤ | Image name click row to open | Image size height x width | Height ã…¤ | Height ã…¤ | Width ã…¤ | Width ã…¤ | Area ã…¤ |
---|---|---|---|---|---|---|---|---|
1âž” | salient_object mask | n03710721_2131.jpg | 271 x 400 | 271px | 100% | 202px | 50.5% | 26.75% |
2âž” | salient_object mask | n03770439_9574.jpg | 400 x 300 | 312px | 78% | 114px | 38% | 16.69% |
3âž” | salient_object mask | n04263257_7125.jpg | 300 x 400 | 260px | 86.67% | 335px | 83.75% | 40.2% |
4âž” | salient_object mask | ILSVRC2012_test_00075096.jpg | 300 x 400 | 95px | 31.67% | 119px | 29.75% | 4.73% |
5âž” | salient_object mask | ILSVRC2013_test_00001641.jpg | 248 x 400 | 236px | 95.16% | 171px | 42.75% | 27.46% |
6âž” | salient_object mask | n03764736_20550.jpg | 400 x 234 | 320px | 80% | 145px | 61.97% | 40.56% |
7âž” | salient_object mask | n04263257_1361.jpg | 400 x 400 | 266px | 66.5% | 330px | 82.5% | 30.12% |
8âž” | salient_object mask | n07753275_5610.jpg | 400 x 300 | 188px | 47% | 151px | 50.33% | 13.77% |
9âž” | salient_object mask | ILSVRC2012_test_00041200.jpg | 307 x 400 | 183px | 59.61% | 213px | 53.25% | 13.46% |
10âž” | salient_object mask | n03676483_14654.jpg | 300 x 400 | 235px | 78.33% | 352px | 88% | 26.93% |
License #
License is unknown for the DUTS dataset.
Citation #
If you make use of the DUTS data, please cite the following reference:
@inproceedings{wang2017,
title={Learning to Detect Salient Objects with Image-level Supervision},
author={Wang, Lijun and Lu, Huchuan and Wang, Yifan and Feng, Mengyang and Wang, Dong, and Yin, Baocai and Ruan, Xiang},
booktitle={CVPR},
year={2017}
}
If you are happy with Dataset Ninja and use provided visualizations and tools in your work, please cite us:
@misc{ visualization-tools-for-duts-dataset,
title = { Visualization Tools for DUTS Dataset },
type = { Computer Vision Tools },
author = { Dataset Ninja },
howpublished = { \url{ https://datasetninja.com/duts } },
url = { https://datasetninja.com/duts },
journal = { Dataset Ninja },
publisher = { Dataset Ninja },
year = { 2025 },
month = { apr },
note = { visited on 2025-04-03 },
}
Download #
Please visit dataset homepage to download the data.
Disclaimer #
Our gal from the legal dep told us we need to post this:
Dataset Ninja provides visualizations and statistics for some datasets that can be found online and can be downloaded by general audience. Dataset Ninja is not a dataset hosting platform and can only be used for informational purposes. The platform does not claim any rights for the original content, including images, videos, annotations and descriptions. Joint publishing is prohibited.
You take full responsibility when you use datasets presented at Dataset Ninja, as well as other information, including visualizations and statistics we provide. You are in charge of compliance with any dataset license and all other permissions. You are required to navigate datasets homepage and make sure that you can use it. In case of any questions, get in touch with us at hello@datasetninja.com.