Introduction #
EALPR: Egyptian Automatic License Plate Recognition (Plates) is a comprehensive dataset tailored for object detection tasks, particularly focused on the recognition and localization of diverse characters found on license plates. Comprising 2068 images, the dataset encompasses a substantial 10797 annotated objects, spread across 27 distinct classes, including characters like ١, م, أ, among others such as ٦, س, ر, and a variety of Arabic characters. The dataset showcases the versatility of characters present on license plates, especially exemplified by the Egyptian License Plate (LP), which typically incorporates Arabic digits and characters. This rich collection captures the variation in characters utilized on plates from different regions, including those that use Latin letters, such as Europe, Brazil, and the US, thereby serving as a valuable resource for training models to recognize and interpret diverse international license plate characters.
EALPR Structure
- EALPR: Vehicles (available on DatasetNinja)
- EALPR: Plates (current)

EALPR: Vehicles demo.

EALPR: Plates demo.
Proposed method
Authors use two object detection models for license plate detection and recognition. The input to authors’ proposed system is the vehicle image, whereas the output is the recognized license plate characters and digits. Tiny-YOLOV3 is the backbone architecture for both stages LP detection and recognition.

Main Stages of the Proposed EALPR Approach.
About EALPR
Authors scrap the EALPR dataset images from websites such as Instagram pages and Facebook Marketplace using web scraping Tools. It has 2,450, and 12,160 characters images including many types of vehicles such as cars, buses, trucks, microbuses, and mini-busses. The vehicle images are captured using different cameras, at varying times, lighting, and background.

Characters and Digits Statistics in the EALPR Dataset.
The Egyptian License Plate (LP) uses the Arabic digits and characters which is varying in several countries that use Latin letters such as Europe, Brazil, US, … etc. It has a dimension 16 (height) X 40 (width) with aspect ratio 1:2 approximately. its layout is divided into 3 regions: the country region contains the Egypt word in Arabic and English format, the character region includes plate characters, and the number region contains plate digits. Egyptian License Plate structure uses 10 Arabic digits and 17 Arabic characters.

Egyptian License Plate Structure.

Plate Arabic Digits and Latin Digits.

Plate Arabic Characters and Latin Characters.
After collecting EALPR dataset authors manually annotate the vehicles, characters, and digits using Ybat tool.
Summary #
EALPR: Egyptian Automatic License Plate Recognition (Plates) is a dataset for an object detection task. It is used in the surveillance industry.
The dataset consists of 2068 images with 10797 labeled objects belonging to 27 different classes including ١, م, أ, and other: ٦, س, ر, ٥, د, ٤, ع, ٧, ھ, ٢, ن, و, ٣, ل, ٩, ج, ب, ى, ٨, ق, ط, ف, ص, and ٠.
Images in the EALPR: Plates dataset have bounding box annotations. There are 90 (4% of the total) unlabeled images (i.e. without annotations). There are no pre-defined train/val/test splits in the dataset. The dataset was released in 2021 by the Fayoum University, Egypt and Minia University, Egypt.
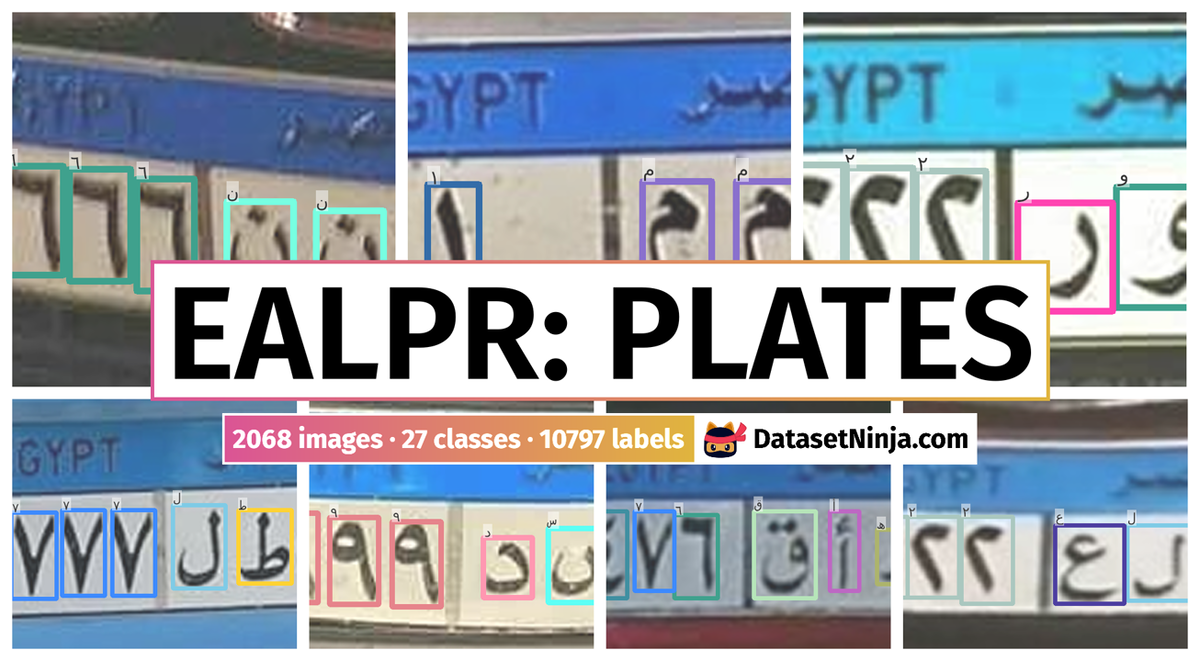
Explore #
EALPR: Plates dataset has 2068 images. Click on one of the examples below or open "Explore" tool anytime you need to view dataset images with annotations. This tool has extended visualization capabilities like zoom, translation, objects table, custom filters and more. Hover the mouse over the images to hide or show annotations.
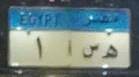
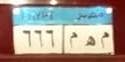
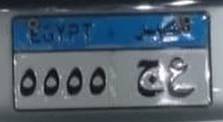
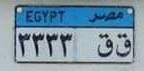
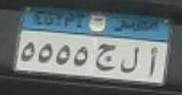
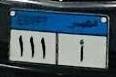



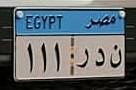

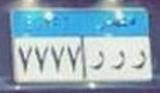
Class balance #
There are 27 annotation classes in the dataset. Find the general statistics and balances for every class in the table below. Click any row to preview images that have labels of the selected class. Sort by column to find the most rare or prevalent classes.
Class ㅤ | Images ㅤ | Objects ㅤ | Count on image average | Area on image average |
---|---|---|---|---|
١➔ rectangle | 769 | 1408 | 1.83 | 4.36% |
م➔ rectangle | 471 | 616 | 1.31 | 4.22% |
أ➔ rectangle | 397 | 465 | 1.17 | 2.8% |
٦➔ rectangle | 378 | 1058 | 2.8 | 9.52% |
س➔ rectangle | 359 | 434 | 1.21 | 4.15% |
ر➔ rectangle | 348 | 383 | 1.1 | 3.33% |
٥➔ rectangle | 310 | 644 | 2.08 | 5.03% |
د➔ rectangle | 296 | 358 | 1.21 | 2.86% |
٤➔ rectangle | 291 | 615 | 2.11 | 7.19% |
ع➔ rectangle | 287 | 323 | 1.13 | 5.21% |
Co-occurrence matrix #
Co-occurrence matrix is an extremely valuable tool that shows you the images for every pair of classes: how many images have objects of both classes at the same time. If you click any cell, you will see those images. We added the tooltip with an explanation for every cell for your convenience, just hover the mouse over a cell to preview the description.
Images #
Explore every single image in the dataset with respect to the number of annotations of each class it has. Click a row to preview selected image. Sort by any column to find anomalies and edge cases. Use horizontal scroll if the table has many columns for a large number of classes in the dataset.
Object distribution #
Interactive heatmap chart for every class with object distribution shows how many images are in the dataset with a certain number of objects of a specific class. Users can click cell and see the list of all corresponding images.
Class sizes #
The table below gives various size properties of objects for every class. Click a row to see the image with annotations of the selected class. Sort columns to find classes with the smallest or largest objects or understand the size differences between classes.
Class | Object count | Avg area | Max area | Min area | Min height | Min height | Max height | Max height | Avg height | Avg height | Min width | Min width | Max width | Max width |
---|---|---|---|---|---|---|---|---|---|---|---|---|---|---|
١ rectangle | 1408 | 2.38% | 5% | 1.11% | 11px | 23.17% | 162px | 51.97% | 34px | 35.33% | 4px | 4.07% | 74px | 13.48% |
٦ rectangle | 1058 | 3.43% | 5.59% | 1.95% | 11px | 25.45% | 108px | 47.14% | 33px | 35.02% | 6px | 6.57% | 54px | 13.35% |
٥ rectangle | 644 | 2.44% | 4.12% | 1.31% | 9px | 17.39% | 64px | 36.08% | 25px | 25.53% | 5px | 6.1% | 49px | 14.46% |
م rectangle | 616 | 3.22% | 5.34% | 1.96% | 11px | 21.95% | 156px | 44.02% | 34px | 33.33% | 6px | 6.4% | 93px | 14% |
٤ rectangle | 615 | 3.42% | 5.45% | 2.01% | 13px | 26.09% | 152px | 52.36% | 38px | 35.53% | 7px | 6.98% | 76px | 13.11% |
٧ rectangle | 550 | 3.53% | 7.47% | 1.95% | 10px | 20.73% | 187px | 48.18% | 34px | 33.63% | 5px | 6.19% | 96px | 15.89% |
أ rectangle | 465 | 2.39% | 4.77% | 1.35% | 14px | 25.45% | 140px | 50.19% | 36px | 36.03% | 5px | 3.66% | 54px | 12.4% |
٢ rectangle | 435 | 3.34% | 6.48% | 1.92% | 11px | 21.25% | 156px | 48.86% | 34px | 35.05% | 7px | 6.86% | 73px | 16.95% |
س rectangle | 434 | 3.44% | 6.02% | 2.01% | 8px | 17.07% | 88px | 41.89% | 24px | 24.67% | 9px | 11.39% | 81px | 19.48% |
٩ rectangle | 403 | 3.37% | 5.8% | 1.82% | 11px | 26.67% | 147px | 51.18% | 37px | 35.24% | 7px | 6.19% | 71px | 13.25% |
Spatial Heatmap #
The heatmaps below give the spatial distributions of all objects for every class. These visualizations provide insights into the most probable and rare object locations on the image. It helps analyze objects' placements in a dataset.
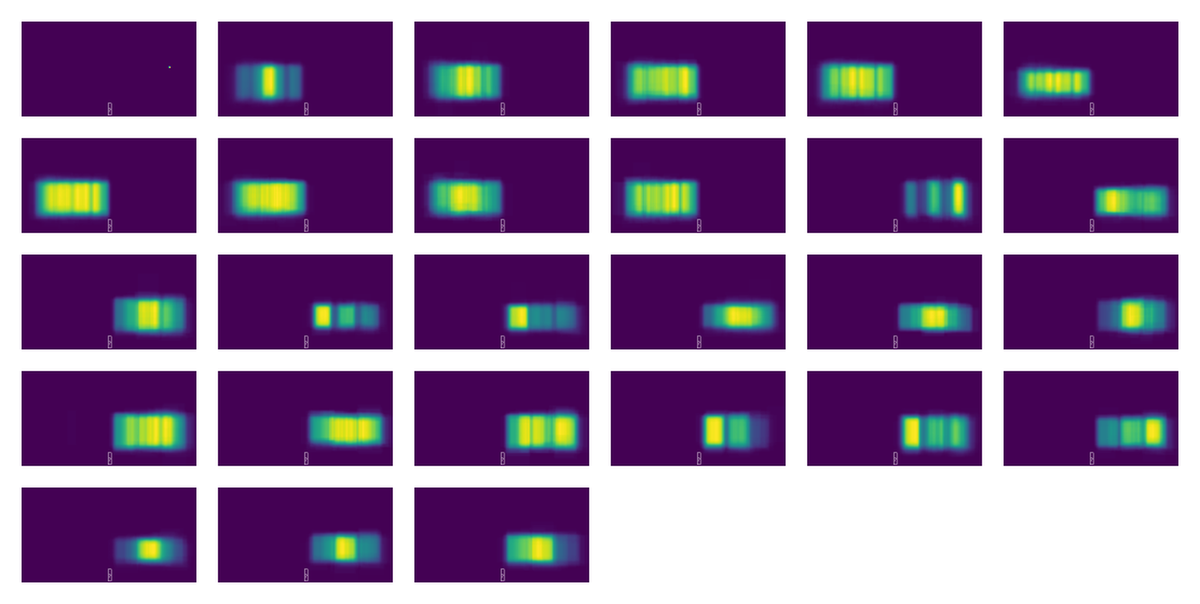
Objects #
Table contains all 10797 objects. Click a row to preview an image with annotations, and use search or pagination to navigate. Sort columns to find outliers in the dataset.
Object ID ㅤ | Class ㅤ | Image name click row to open | Image size height x width | Height ㅤ | Height ㅤ | Width ㅤ | Width ㅤ | Area ㅤ |
---|---|---|---|---|---|---|---|---|
1➔ | ٩ rectangle | 1087_license_plate_1.png | 95 x 164 | 31px | 32.63% | 13px | 7.93% | 2.59% |
2➔ | م rectangle | 1087_license_plate_1.png | 95 x 164 | 33px | 34.74% | 15px | 9.15% | 3.18% |
3➔ | م rectangle | 1087_license_plate_1.png | 95 x 164 | 31px | 32.63% | 13px | 7.93% | 2.59% |
4➔ | ١ rectangle | 1571_license_plate_1.png | 75 x 131 | 27px | 36% | 11px | 8.4% | 3.02% |
5➔ | ١ rectangle | 1571_license_plate_1.png | 75 x 131 | 25px | 33.33% | 13px | 9.92% | 3.31% |
6➔ | ١ rectangle | 1571_license_plate_1.png | 75 x 131 | 27px | 36% | 11px | 8.4% | 3.02% |
7➔ | ١ rectangle | 1571_license_plate_1.png | 75 x 131 | 29px | 38.67% | 11px | 8.4% | 3.25% |
8➔ | م rectangle | 1571_license_plate_1.png | 75 x 131 | 29px | 38.67% | 13px | 9.92% | 3.84% |
9➔ | ر rectangle | 1571_license_plate_1.png | 75 x 131 | 21px | 28% | 17px | 12.98% | 3.63% |
10➔ | أ rectangle | 1571_license_plate_1.png | 75 x 131 | 28px | 37.33% | 11px | 8.4% | 3.13% |
License #
License is unknown for the Egyptian Automatic License Plate Recognition: Plates dataset.
Citation #
If you make use of the EALPR: Plates data, please cite the following reference:
@INPROCEEDINGS{9845514,
author={Youssef, Ahmed Ramadan and Sayed, Fawzya Ramadan and Ali, Abdelmgeid Ameen},
booktitle={2022 7th Asia-Pacific Conference on Intelligent Robot Systems (ACIRS)},
title={A New Benchmark Dataset for Egyptian License Plate Detection and Recognition},
year={2022},
volume={},
number={},
pages={106-111},
doi={10.1109/ACIRS55390.2022.9845514}
}
If you are happy with Dataset Ninja and use provided visualizations and tools in your work, please cite us:
@misc{ visualization-tools-for-ealpr-plates-dataset,
title = { Visualization Tools for EALPR: Plates Dataset },
type = { Computer Vision Tools },
author = { Dataset Ninja },
howpublished = { \url{ https://datasetninja.com/ealpr-plates } },
url = { https://datasetninja.com/ealpr-plates },
journal = { Dataset Ninja },
publisher = { Dataset Ninja },
year = { 2025 },
month = { may },
note = { visited on 2025-05-18 },
}
Download #
Please visit dataset homepage to download the data.
Disclaimer #
Our gal from the legal dep told us we need to post this:
Dataset Ninja provides visualizations and statistics for some datasets that can be found online and can be downloaded by general audience. Dataset Ninja is not a dataset hosting platform and can only be used for informational purposes. The platform does not claim any rights for the original content, including images, videos, annotations and descriptions. Joint publishing is prohibited.
You take full responsibility when you use datasets presented at Dataset Ninja, as well as other information, including visualizations and statistics we provide. You are in charge of compliance with any dataset license and all other permissions. You are required to navigate datasets homepage and make sure that you can use it. In case of any questions, get in touch with us at hello@datasetninja.com.