Introduction #
The authors of the Food Recognition 2022 dataset emphasize the significance of image-based food recognition, which holds utility across various applications. Particularly, this recognition capability offers a streamlined method for individuals to monitor their dietary intake by capturing images of consumed food. Such tracking has both personal and medical implications, as it assists in studies focusing on participants’ food intake, a task that has previously relied on imprecise food frequency questionnaires.
The advancement of deep learning has significantly propelled image-based food recognition in recent years. However, the complex nature of food recognition persists due to numerous challenges. This benchmark, now in its third consecutive year on AIcrowd, continues to build upon the achievements of the previous Food Recognition Challenges held in 2019, 2020, and 2021.
The primary objective of this benchmark is to train models capable of examining images of food items and identifying the individual components within them. To facilitate this, a novel dataset of food images has been curated using the MyFoodRepo app. This app involves Swiss volunteers who contribute images of their daily food consumption as part of the digital cohort named Food & You.
This evolving dataset has been meticulously annotated, or its automatic annotations have been validated, with respect to segmentation, classification (mapping individual food items to a Swiss Food ontology), and estimations of weight/volume. As the dataset continues to expand, additional data will be periodically released.
Locating annotated food images poses challenges, as most existing databases possess limited annotations. Notably, the majority of food images available online are visually appealing but often diverge from real-world representations. Algorithms require real-world images, and proper annotations are generally lacking. Ideally, food images should be accompanied by accurate segmentation, classification, and volume/weight estimates.
Summary #
Food Recognition 2022 v2.0 is a dataset for a semantic segmentation task. Possible applications of the dataset could be in the food and medical industries.
The dataset consists of 43962 images with 95009 labeled objects belonging to 498 different classes including water, salad-leaf-salad-green, bread-white, and other: tomato-raw, butter, carrot-raw, bread-wholemeal, coffee-with-caffeine, rice, egg, apple, mixed-vegetables, jam, cucumber, wine-red, cheese, banana, potatoes-steamed, bell-pepper-red-raw, espresso-with-caffeine, hard-cheese, bread-whole-wheat, tea, mixed-salad-chopped-without-sauce, avocado, white-coffee-with-caffeine, tomato-sauce, wine-white, and 470 more.
Images in the Food Recognition 2022 dataset have pixel-level semantic segmentation annotations. There are 3001 (7% of the total) unlabeled images (i.e. without annotations). There are 3 splits in the dataset: training (39962 images), test (3000 images), and validation (1000 images). The dataset was released in 2022 by the AIcrowd, Switzerland.
Here is a visualized example for randomly selected sample classes:
Explore #
Food Recognition 2022 dataset has 43962 images. Click on one of the examples below or open "Explore" tool anytime you need to view dataset images with annotations. This tool has extended visualization capabilities like zoom, translation, objects table, custom filters and more. Hover the mouse over the images to hide or show annotations.
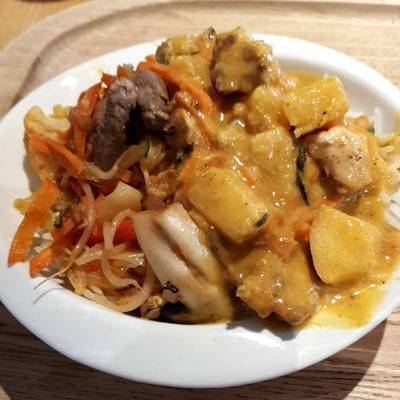
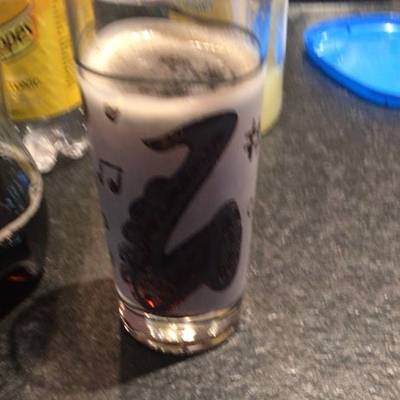
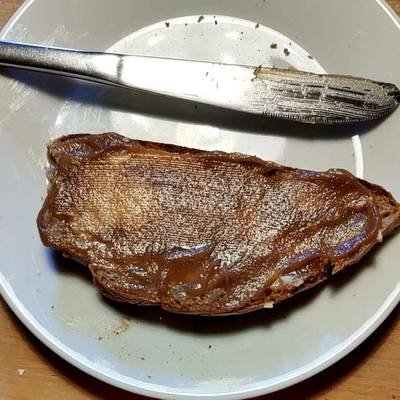
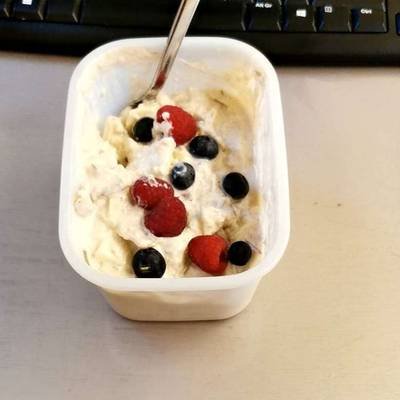
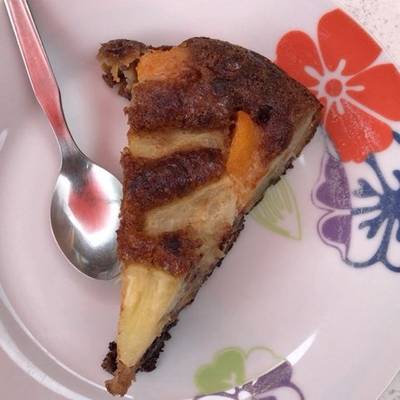
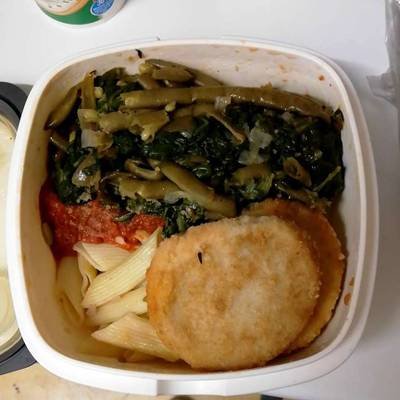


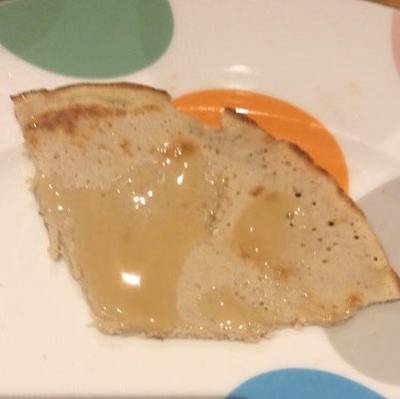
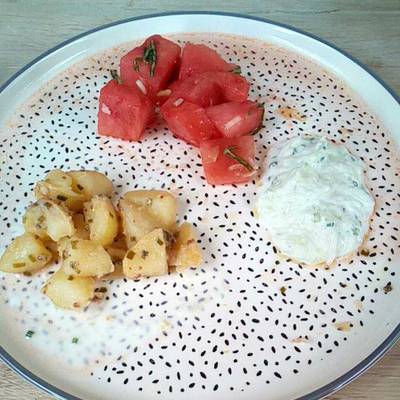
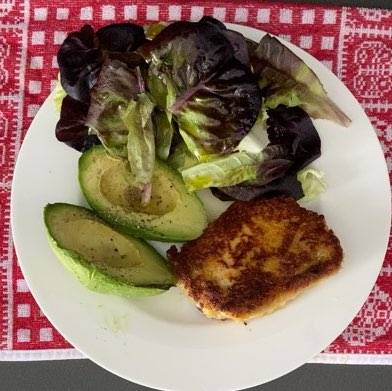
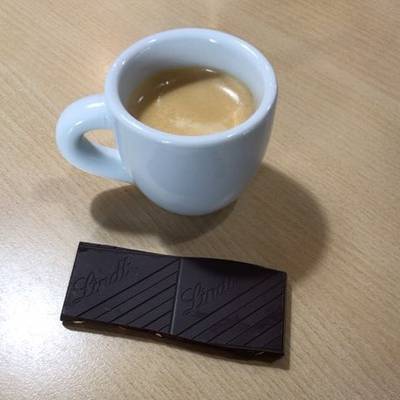


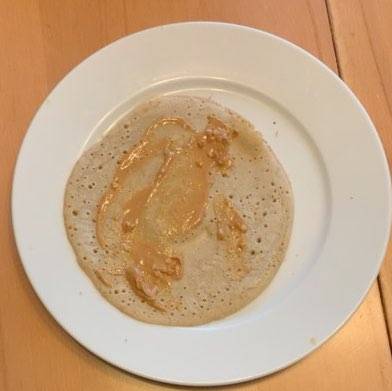

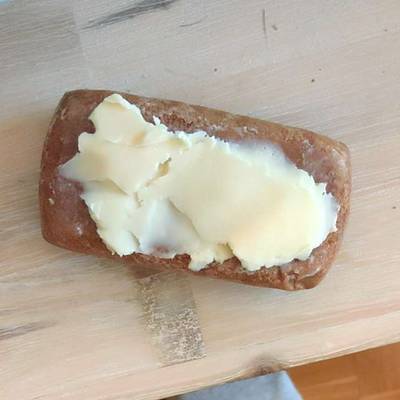


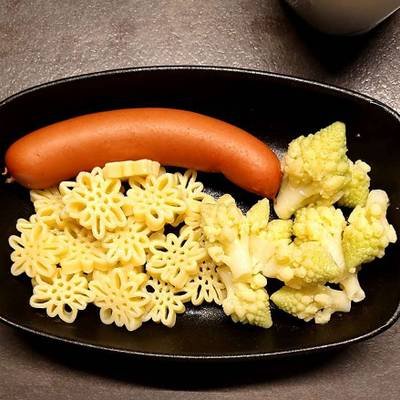

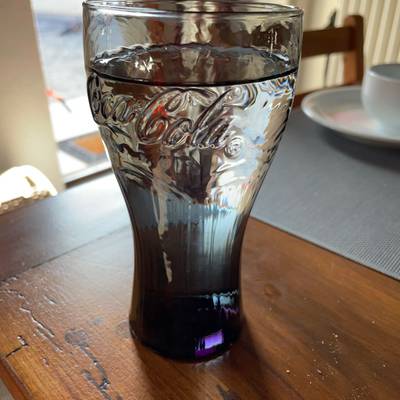
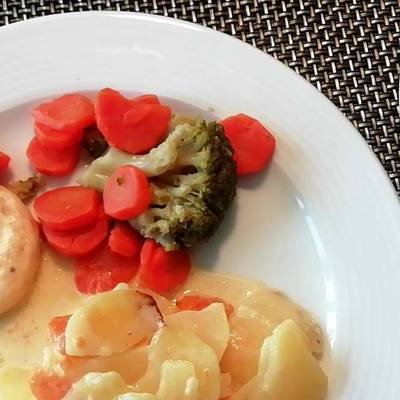
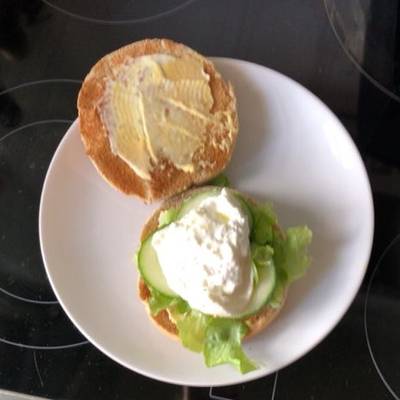

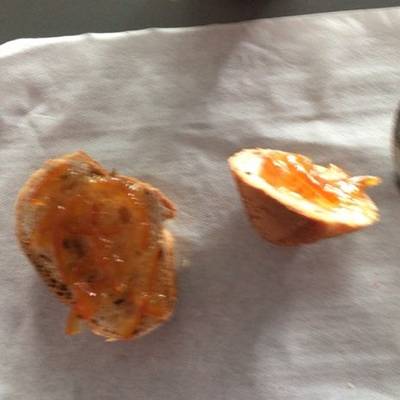

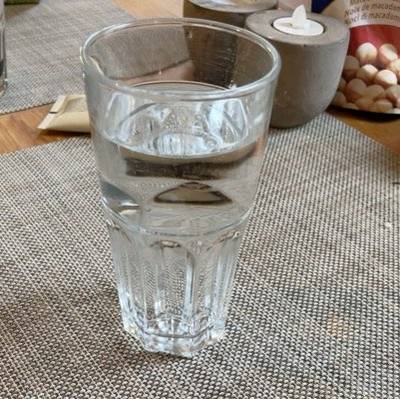
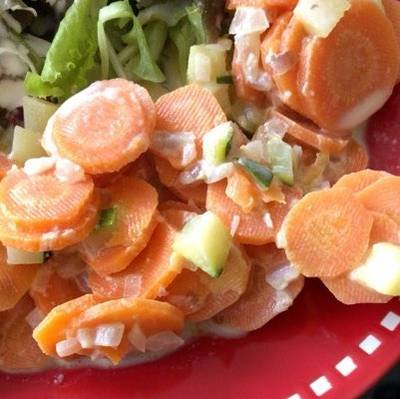
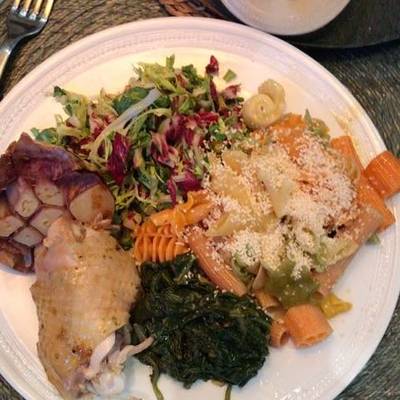
Class balance #
There are 498 annotation classes in the dataset. Find the general statistics and balances for every class in the table below. Click any row to preview images that have labels of the selected class. Sort by column to find the most rare or prevalent classes.
Class ã…¤ | Images ã…¤ | Objects ã…¤ | Count on image average | Area on image average |
---|---|---|---|---|
waterâž” polygon | 3002 | 5414 | 1.8 | 23.66% |
salad-leaf-salad-greenâž” polygon | 2064 | 2385 | 1.16 | 32.92% |
bread-whiteâž” polygon | 1930 | 2265 | 1.17 | 30.27% |
tomato-rawâž” polygon | 1925 | 3352 | 1.74 | 11.28% |
butterâž” polygon | 1642 | 2441 | 1.49 | 11.61% |
carrot-rawâž” polygon | 1509 | 2220 | 1.47 | 13.28% |
bread-wholemealâž” polygon | 1488 | 1708 | 1.15 | 27.04% |
coffee-with-caffeineâž” polygon | 1433 | 2035 | 1.42 | 13.46% |
riceâž” polygon | 1048 | 1204 | 1.15 | 21.03% |
eggâž” polygon | 1035 | 1279 | 1.24 | 17.31% |
Images #
Explore every single image in the dataset with respect to the number of annotations of each class it has. Click a row to preview selected image. Sort by any column to find anomalies and edge cases. Use horizontal scroll if the table has many columns for a large number of classes in the dataset.
Class sizes #
The table below gives various size properties of objects for every class. Click a row to see the image with annotations of the selected class. Sort columns to find classes with the smallest or largest objects or understand the size differences between classes.
Class | Object count | Avg area | Max area | Min area | Min height | Min height | Max height | Max height | Avg height | Avg height | Min width | Min width | Max width | Max width |
---|---|---|---|---|---|---|---|---|---|---|---|---|---|---|
water polygon | 5414 | 23.49% | 81.81% | 0% | 2px | 0.35% | 1896px | 98.54% | 363px | 56.73% | 2px | 0.42% | 2717px | 98.75% |
tomato-raw polygon | 3352 | 6.63% | 81.06% | 0% | 2px | 0.21% | 1997px | 99.34% | 173px | 26.7% | 3px | 0.32% | 1896px | 99.78% |
butter polygon | 2441 | 8.03% | 67.56% | 0% | 3px | 0.35% | 2189px | 99.38% | 202px | 31.81% | 3px | 0.47% | 2803px | 98.5% |
salad-leaf-salad-green polygon | 2385 | 28.71% | 92.08% | 0% | 2px | 0.42% | 3186px | 99.75% | 382px | 59.12% | 3px | 0.48% | 2979px | 100% |
bread-white polygon | 2265 | 27.01% | 82.62% | 0% | 5px | 0.64% | 3509px | 99.17% | 365px | 56.89% | 4px | 0.43% | 2976px | 99.73% |
carrot-raw polygon | 2220 | 9.01% | 86.9% | 0% | 2px | 0.42% | 3136px | 98.77% | 222px | 34.08% | 2px | 0.42% | 2934px | 98.97% |
coffee-with-caffeine polygon | 2035 | 13.64% | 87.39% | 0% | 2px | 0.42% | 1467px | 98% | 205px | 33.1% | 2px | 0.32% | 1967px | 98% |
bread-wholemeal polygon | 1708 | 24.2% | 88.22% | 0% | 4px | 0.39% | 1442px | 99.3% | 359px | 53.74% | 4px | 0.39% | 1513px | 99.58% |
egg polygon | 1279 | 14.6% | 80.46% | 0.01% | 9px | 1.14% | 1599px | 99% | 256px | 40.88% | 8px | 0.9% | 1271px | 99% |
rice polygon | 1204 | 18.37% | 87.37% | 0% | 5px | 0.88% | 1966px | 99.38% | 313px | 47.79% | 6px | 0.59% | 2208px | 99.25% |
Spatial Heatmap #
The heatmaps below give the spatial distributions of all objects for every class. These visualizations provide insights into the most probable and rare object locations on the image. It helps analyze objects' placements in a dataset.

Objects #
Table contains all 95009 objects. Click a row to preview an image with annotations, and use search or pagination to navigate. Sort columns to find outliers in the dataset.
Object ID ã…¤ | Class ã…¤ | Image name click row to open | Image size height x width | Height ã…¤ | Height ã…¤ | Width ã…¤ | Width ã…¤ | Area ã…¤ |
---|---|---|---|---|---|---|---|---|
1âž” | bread polygon | 145958.jpg | 972 x 973 | 683px | 70.27% | 898px | 92.29% | 34% |
2âž” | tomato-raw polygon | 145958.jpg | 972 x 973 | 634px | 65.23% | 806px | 82.84% | 28.1% |
3âž” | hummus polygon | 145958.jpg | 972 x 973 | 257px | 26.44% | 385px | 39.57% | 3.5% |
4âž” | hummus polygon | 145958.jpg | 972 x 973 | 256px | 26.34% | 242px | 24.87% | 2.64% |
5âž” | onion polygon | 145958.jpg | 972 x 973 | 341px | 35.08% | 483px | 49.64% | 9.65% |
6âž” | onion polygon | 145958.jpg | 972 x 973 | 325px | 33.44% | 420px | 43.17% | 5.64% |
7âž” | onion polygon | 145958.jpg | 972 x 973 | 239px | 24.59% | 218px | 22.4% | 4.1% |
8âž” | fish-fingers-breaded polygon | 126651.jpg | 464 x 464 | 452px | 97.41% | 90px | 19.4% | 15.38% |
9âž” | salmon polygon | 131323.jpg | 972 x 973 | 949px | 97.63% | 966px | 99.28% | 69.65% |
10âž” | risotto-without-cheese-cooked polygon | 177552.jpg | 840 x 840 | 500px | 59.52% | 470px | 55.95% | 20.93% |
License #
Citation #
If you make use of the Food Recognition 2022 data, please cite the following reference:
@dataset{Food Recognition 2022,
author={AIcrowd},
title={Food Recognition 2022},
year={2022},
url={https://www.kaggle.com/datasets/awsaf49/food-recognition-2022-dataset}
}
If you are happy with Dataset Ninja and use provided visualizations and tools in your work, please cite us:
@misc{ visualization-tools-for-food-recognition-dataset,
title = { Visualization Tools for Food Recognition 2022 Dataset },
type = { Computer Vision Tools },
author = { Dataset Ninja },
howpublished = { \url{ https://datasetninja.com/food-recognition } },
url = { https://datasetninja.com/food-recognition },
journal = { Dataset Ninja },
publisher = { Dataset Ninja },
year = { 2025 },
month = { apr },
note = { visited on 2025-04-26 },
}
Download #
Dataset Food Recognition 2022 can be downloaded in Supervisely format:
As an alternative, it can be downloaded with dataset-tools package:
pip install --upgrade dataset-tools
… using following python code:
import dataset_tools as dtools
dtools.download(dataset='Food Recognition 2022', dst_dir='~/dataset-ninja/')
Make sure not to overlook the python code example available on the Supervisely Developer Portal. It will give you a clear idea of how to effortlessly work with the downloaded dataset.
The data in original format can be downloaded here.
Disclaimer #
Our gal from the legal dep told us we need to post this:
Dataset Ninja provides visualizations and statistics for some datasets that can be found online and can be downloaded by general audience. Dataset Ninja is not a dataset hosting platform and can only be used for informational purposes. The platform does not claim any rights for the original content, including images, videos, annotations and descriptions. Joint publishing is prohibited.
You take full responsibility when you use datasets presented at Dataset Ninja, as well as other information, including visualizations and statistics we provide. You are in charge of compliance with any dataset license and all other permissions. You are required to navigate datasets homepage and make sure that you can use it. In case of any questions, get in touch with us at hello@datasetninja.com.