Introduction #
The GlaS@MICCAI’2015: Gland Segmentation dataset used in the GlaS@MICCAI’2015 challenge consists of 165 images derived from 16 H&E stained histological sections of stage T3 or T4 colorectal adenocarcinoma. The T in TNM cancer staging refers to the spread of the primary tumour). In colorectal cancer, stage T3 means the tumour has grown into the outer lining of the bowel wall, whereas stage T4 means the tumour has grown through the outer lining of the bowel wall. The cancer stage is different from the tumour histologic grade, as the latter indicates the aggressiveness of the tumour. Each section belongs to a different patient, and sections were processed in the laboratory on different occasions. Thus, the dataset exhibits high inter-subject variability in both stain distribution and tissue architecture. The digitization of these histological sections into whole-slide images (WSIs) was accomplished using a Zeiss MIRAX MIDI Slide Scanner with a pixel resolution of 0.465µm. The WSIs were subsequently rescaled to a pixel resolution of 0.620µm (equivalent to 20× objective magnification).
A total of 52 visual fields from both malignant and benign areas across the entire set of WSIs were selected in order to cover as wide a variety of tissue architectures as possible. An expert pathologist (DRJS) then graded each visual field as either benign or malignant, according to the overall glandular architecture. The pathologist also delineated the boundary of each individual glandular object on that visual field. Authors used this manual annotation as ground truth for automatic segmentation. Note that different glandular objects in an image may be part of the same gland. This is because a gland is a 3-dimensional structure that can appear as separate objects on a single tissue section. The visual fields were further separated into smaller, non-overlapping images, whose histologic grades (i.e. benign or malignant) were assigned the same value as the larger visual field. This dataset was also previously used in the gland segmentation study by Sirinukunwattana et al.
In the challenge, the dataset was separated into train, test_a, and test_b. Note that the data were stratified according to the histologic grade and the visual field before splitting. This was done to ensure that none of the images from the same visual field appeared in different parts of the dataset (i.e. train, test_a, or test_b). However, since the data were not stratified based on patients, different visual fields from the same slide can appear in different parts of the dataset. The ground truth as well as the histologic grade which reflects the morphology of glandular structures were provided for every image in the Training Part at the time of release. The authors used test_a and test_b as off-site and on-site test datasets respectively. Furthermore, to ensure blindness of evaluation, the ground truth and histologic grade of each image in the test parts were not released to the participants.
Summary #
GlaS@MICCAI’2015: Gland Segmentation is a dataset for instance segmentation, semantic segmentation, and object detection tasks. It is used in the medical industry, and in the medical research.
The dataset consists of 165 images with 1530 labeled objects belonging to 1 single class (gland).
Images in the GlaS@MICCAI’2015: Gland Segmentation dataset have pixel-level instance segmentation annotations. Due to the nature of the instance segmentation task, it can be automatically transformed into a semantic segmentation (only one mask for every class) or object detection (bounding boxes for every object) tasks. All images are labeled (i.e. with annotations). There are 3 splits in the dataset: train (85 images), test_a (60 images), and test_b (20 images). Also, the dataset contains patient_id, grade_sirinukunwattana and malignant/benign tags. The dataset was released in 2015 by the University of Warwick, Eindhoven University of Technology, The Chinese University of Hong Kong, University of Central Lancashire, University of Freiburg, ExB Research and Development, Biomedical Imaging Laboratory (LIB), Medical University of Graz, University of Zurich and ETH Zurich, Graz University of Technology, Ludwig Boltzmann Institute for Clinical Forensic Imaging, and University Hospitals Coventry and Warwickshire.
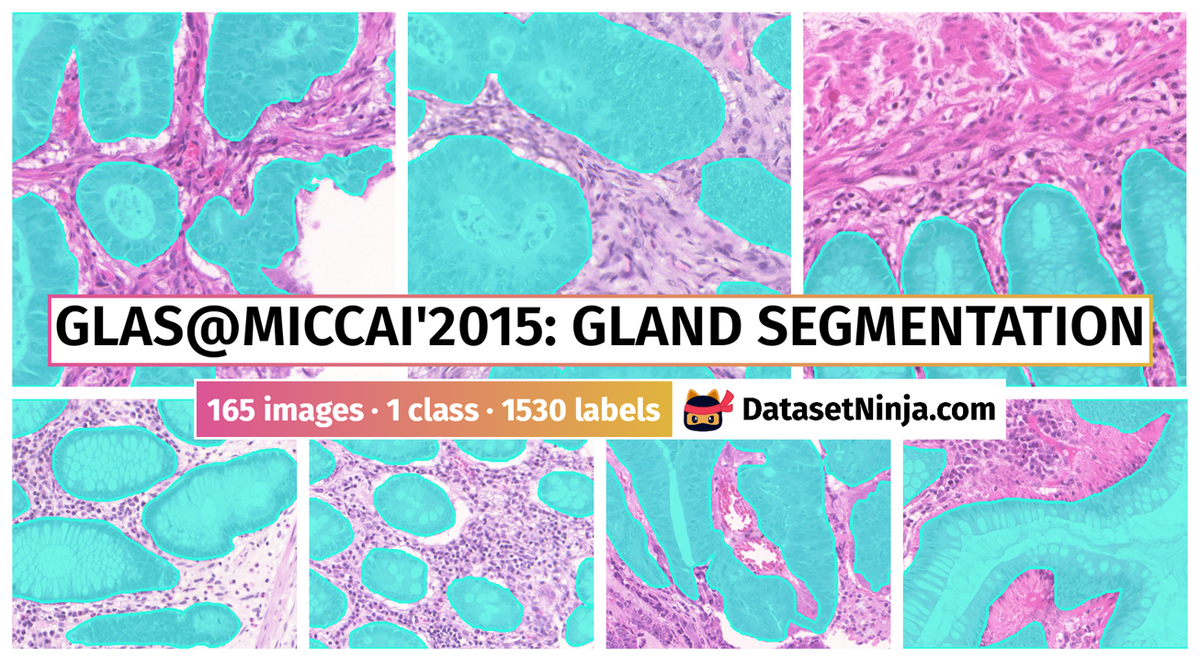
Explore #
GlaS@MICCAI'2015: Gland Segmentation dataset has 165 images. Click on one of the examples below or open "Explore" tool anytime you need to view dataset images with annotations. This tool has extended visualization capabilities like zoom, translation, objects table, custom filters and more. Hover the mouse over the images to hide or show annotations.
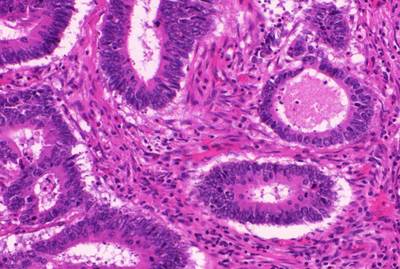
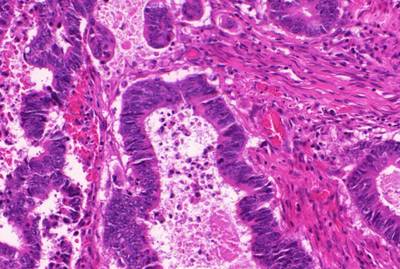
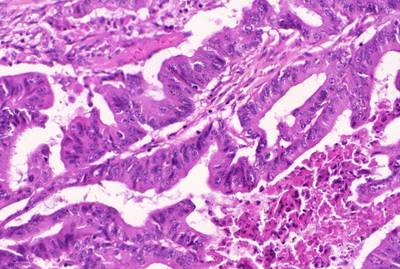

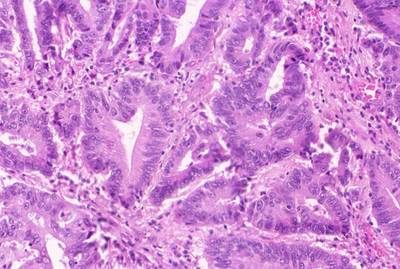
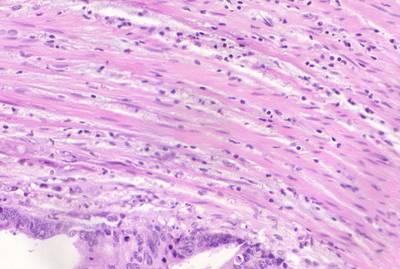
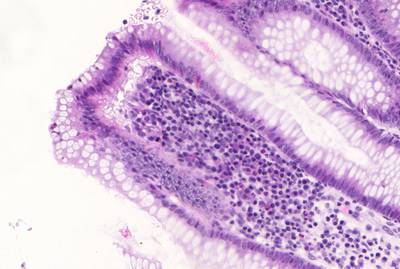
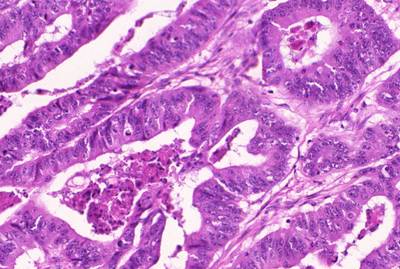
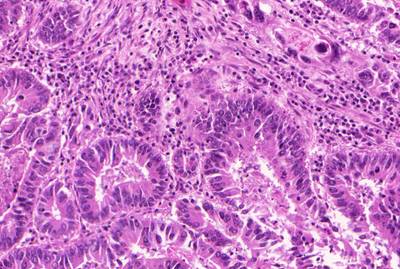
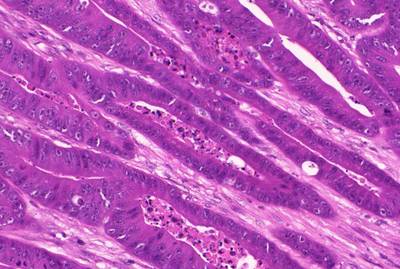
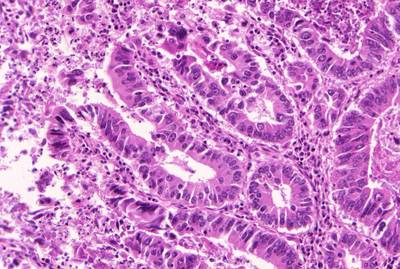
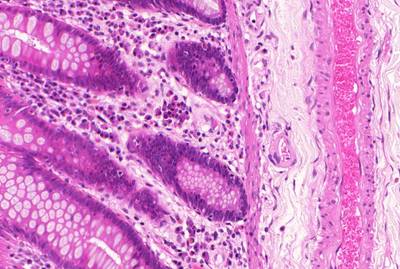
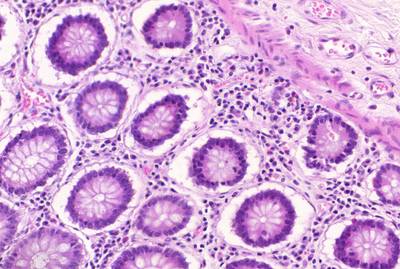
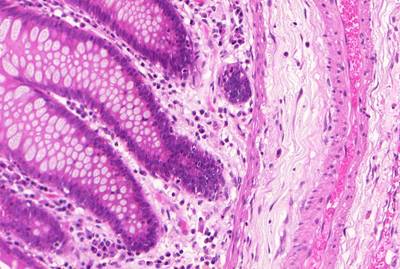
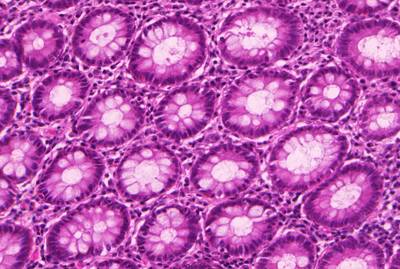
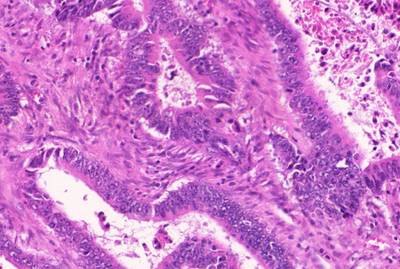
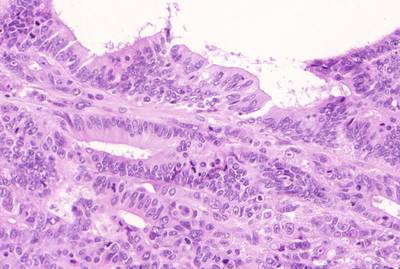
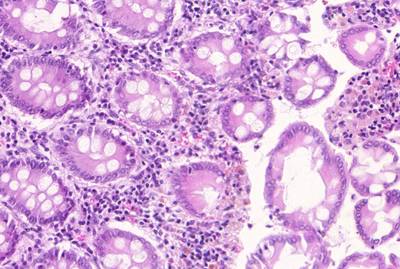
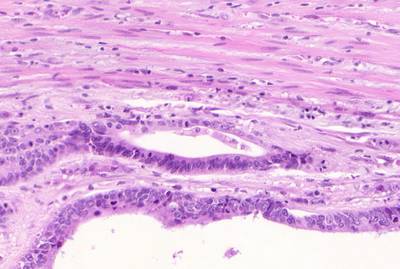
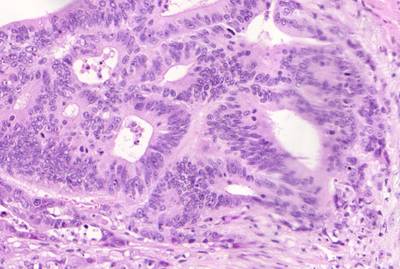
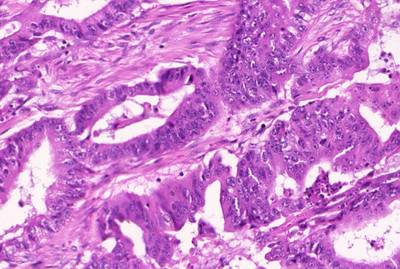
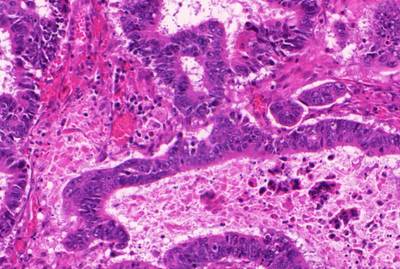
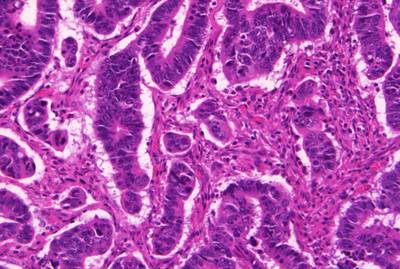
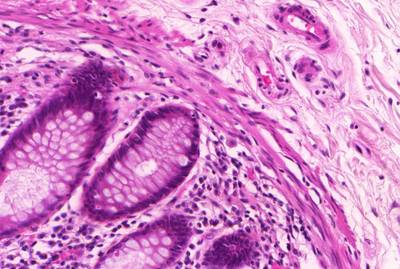
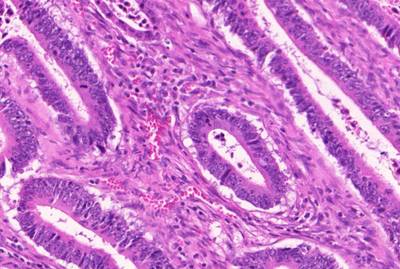
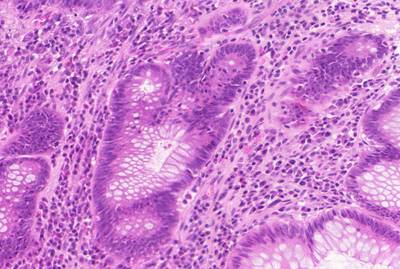
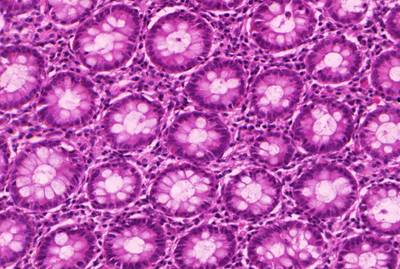

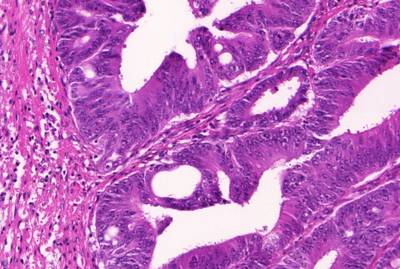

Class balance #
There are 1 annotation classes in the dataset. Find the general statistics and balances for every class in the table below. Click any row to preview images that have labels of the selected class. Sort by column to find the most rare or prevalent classes.
Class ㅤ | Images ㅤ | Objects ㅤ | Count on image average | Area on image average |
---|---|---|---|---|
gland➔ mask | 165 | 1530 | 9.27 | 50.71% |
Images #
Explore every single image in the dataset with respect to the number of annotations of each class it has. Click a row to preview selected image. Sort by any column to find anomalies and edge cases. Use horizontal scroll if the table has many columns for a large number of classes in the dataset.
Object distribution #
Interactive heatmap chart for every class with object distribution shows how many images are in the dataset with a certain number of objects of a specific class. Users can click cell and see the list of all corresponding images.
Class sizes #
The table below gives various size properties of objects for every class. Click a row to see the image with annotations of the selected class. Sort columns to find classes with the smallest or largest objects or understand the size differences between classes.
Class | Object count | Avg area | Max area | Min area | Min height | Min height | Max height | Max height | Avg height | Avg height | Min width | Min width | Max width | Max width |
---|---|---|---|---|---|---|---|---|---|---|---|---|---|---|
gland mask | 1530 | 5.47% | 88.52% | 0% | 1px | 0.19% | 522px | 100% | 152px | 29.55% | 1px | 0.13% | 775px | 100% |
Spatial Heatmap #
The heatmaps below give the spatial distributions of all objects for every class. These visualizations provide insights into the most probable and rare object locations on the image. It helps analyze objects' placements in a dataset.
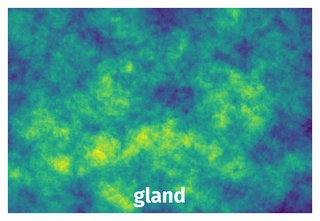
Objects #
Table contains all 1530 objects. Click a row to preview an image with annotations, and use search or pagination to navigate. Sort columns to find outliers in the dataset.
Object ID ㅤ | Class ㅤ | Image name click row to open | Image size height x width | Height ㅤ | Height ㅤ | Width ㅤ | Width ㅤ | Area ㅤ |
---|---|---|---|---|---|---|---|---|
1➔ | gland mask | train_62.bmp | 522 x 775 | 120px | 22.99% | 114px | 14.71% | 2.44% |
2➔ | gland mask | train_62.bmp | 522 x 775 | 179px | 34.29% | 486px | 62.71% | 10.99% |
3➔ | gland mask | train_62.bmp | 522 x 775 | 121px | 23.18% | 267px | 34.45% | 3.21% |
4➔ | gland mask | train_62.bmp | 522 x 775 | 116px | 22.22% | 445px | 57.42% | 10.12% |
5➔ | gland mask | train_62.bmp | 522 x 775 | 53px | 10.15% | 151px | 19.48% | 1.42% |
6➔ | gland mask | train_62.bmp | 522 x 775 | 1px | 0.19% | 1px | 0.13% | 0% |
7➔ | gland mask | train_62.bmp | 522 x 775 | 157px | 30.08% | 292px | 37.68% | 7.16% |
8➔ | gland mask | train_62.bmp | 522 x 775 | 116px | 22.22% | 98px | 12.65% | 2.02% |
9➔ | gland mask | train_53.bmp | 522 x 775 | 233px | 44.64% | 321px | 41.42% | 11.47% |
10➔ | gland mask | train_53.bmp | 522 x 775 | 93px | 17.82% | 36px | 4.65% | 0.57% |
License #
Data Usage
The dataset used in this competition is provided for research purposes only. Commercial uses are not allowed.
If you intend to publish research work that uses this dataset, you must cite our review paper to be published after the competition
K. Sirinukunwattana, J. P. W. Pluim, H. Chen, X Qi, P. Heng, Y. Guo, L. Wang, B. J. Matuszewski, E. Bruni, U. Sanchez, A. Böhm, O. Ronneberger, B. Ben Cheikh, D. Racoceanu, P. Kainz, M. Pfeiffer, M. Urschler, D. R. J. Snead, N. M. Rajpoot
"Gland Segmentation in Colon Histology Images: The GlaS Challenge Contest"
http://arxiv.org/abs/1603.00275 [Preprint]
The details of the journal version will be available soon.
AND the following paper, wherein the same dataset was first used:
K. Sirinukunwattana, D.R.J. Snead, N.M. Rajpoot,
"A Stochastic Polygons Model for Glandular Structures in Colon Histology Images," in IEEE Transactions on Medical Imaging, 2015
doi: 10.1109/TMI.2015.2433900
Citation #
If you make use of the GlaS@MICCAI’2015: Gland Segmentation data, please cite the following reference:
@misc{sirinukunwattana2016gland,
title={Gland Segmentation in Colon Histology Images: The GlaS Challenge Contest},
author={Korsuk Sirinukunwattana and Josien P. W. Pluim and Hao Chen and Xiaojuan Qi and Pheng-Ann Heng and Yun Bo Guo and Li Yang Wang and Bogdan J. Matuszewski and Elia Bruni and Urko Sanchez and Anton Böhm and Olaf Ronneberger and Bassem Ben Cheikh and Daniel Racoceanu and Philipp Kainz and Michael Pfeiffer and Martin Urschler and David R. J. Snead and Nasir M. Rajpoot},
year={2016},
eprint={1603.00275},
archivePrefix={arXiv},
primaryClass={cs.CV}
}
If you are happy with Dataset Ninja and use provided visualizations and tools in your work, please cite us:
@misc{ visualization-tools-for-gland-segmentation-dataset,
title = { Visualization Tools for GlaS@MICCAI'2015: Gland Segmentation Dataset },
type = { Computer Vision Tools },
author = { Dataset Ninja },
howpublished = { \url{ https://datasetninja.com/gland-segmentation } },
url = { https://datasetninja.com/gland-segmentation },
journal = { Dataset Ninja },
publisher = { Dataset Ninja },
year = { 2025 },
month = { apr },
note = { visited on 2025-04-02 },
}
Download #
Dataset GlaS@MICCAI’2015: Gland Segmentation can be downloaded in Supervisely format:
As an alternative, it can be downloaded with dataset-tools package:
pip install --upgrade dataset-tools
… using following python code:
import dataset_tools as dtools
dtools.download(dataset='GlaS@MICCAI'2015: Gland Segmentation', dst_dir='~/dataset-ninja/')
Make sure not to overlook the python code example available on the Supervisely Developer Portal. It will give you a clear idea of how to effortlessly work with the downloaded dataset.
The data in original format can be downloaded here.
Disclaimer #
Our gal from the legal dep told us we need to post this:
Dataset Ninja provides visualizations and statistics for some datasets that can be found online and can be downloaded by general audience. Dataset Ninja is not a dataset hosting platform and can only be used for informational purposes. The platform does not claim any rights for the original content, including images, videos, annotations and descriptions. Joint publishing is prohibited.
You take full responsibility when you use datasets presented at Dataset Ninja, as well as other information, including visualizations and statistics we provide. You are in charge of compliance with any dataset license and all other permissions. You are required to navigate datasets homepage and make sure that you can use it. In case of any questions, get in touch with us at hello@datasetninja.com.