Introduction #
The LogoDet-3K: A Large-Scale Image Dataset for Logo Detection is a significant contribution to the logo detection, with broad applications in multimedia such as copyright infringement detection, brand visibility monitoring, and product brand management on social media. It features 3,000 logo categories, approximately 200,000 manually annotated logo objects, and 158,652 images, sets a new standard for logo detection benchmarks. The authors detail the dataset construction process, including logo image collection, filtering, and object annotation. The dataset is divided into nine super-classes based on daily life needs and common enterprise positioning.
For the construction of LogoDet-3K, the authors followed a meticulous three-step process, involving logo image collection, logo image filtering, and logo object annotation. Each image was thoroughly examined and reviewed to ensure the dataset’s quality after filtering and annotation.
Logo image collection
During the phase, it was compiled a comprehensive logo list based on various reputable sources, resulting in a vocabulary of 3,000 logo names spanning nine super-classes: sports, leisure, transportation, food, electronic, necessities, clothes, medical, others. Images were then retrieved from search engines using these names as queries. The dataset creation process involved manual cleaning and filtering to ensure data quality, including the removal of images with unsuitable sizes or extreme aspect ratios and those without logos.
Root Category | Sub-Category | Images | Objects |
---|---|---|---|
Food | 932 | 53,350 | 64,276 |
Clothes | 604 | 31,266 | 37,601 |
Necessities | 432 | 24,822 | 30,643 |
Others | 371 | 15,513 | 20,016 |
Electronic | 224 | 9,675 | 12,139 |
Transportation | 213 | 10,445 | 12,791 |
Leisure | 111 | 5,685 | 6,573 |
Sports | 66 | 3,945 | 5,041 |
Medical | 47 | 3,945 | 5,185 |
Total | 3,000 | 158,652 | 194,261 |
Logo object annotation
During the process a considerable amount of time was required. The resulting LogoDet-3K dataset consists of 3,000 logo classes, 158,652 images, and 194,261 logo objects. The dataset exhibits imbalanced distributions across different logo categories, presenting a challenge for effective logo detection, particularly for categories with fewer samples. The statistics at the superclass and category levels illustrate this imbalance.
Multiple logo categories for some brands, where a distinction between these logo categories via adding the suffix ‘-1’, ‘-2’.
Additionally, the authors provide insights into the distribution of images and categories in LogoDet-3K, highlighting the challenges posed by imbalances across different logo objects and images. The dataset is characterized by a large percentage of small and medium logo objects, creating additional challenges for logo detection, as smaller logos are inherently harder to detect.
Sorted distribution of images for each logo in LogoDet-3K.
The dataset’s statistics further detail the distribution of logo categories, images, and logo objects across nine super-classes, revealing variations in sizes and numbers. Notably, the Food, Clothes, and Necessities classes exhibit larger numbers of objects and images compared to other classes in the dataset.
The detailed statistics of LogoDet-3K about Image and object distribution in per category, the number of objects in per image and object size in per image.
Distributions of categories, images and objects from LogoDet-3K on super-classes.
Summary #
LogoDet-3K is a dataset for an object detection task. It is used in the retail industry.
The dataset consists of 158654 images with 194261 labeled objects belonging to 3000 different classes including lexus-1, avery_dennison-2, alpinestars-2, and other: new_balance-1, 76, maybach-1, mclaren, lexus-2, kichesippi-2, zendium, yuyue-2, nongfu_spring-2, longmu-1, longmu-2, square_one_organic, steeden, neil_pryde-2, new_balance-2, tiffany, kichesippi-1, yuyue-1, auxx-2, chow_tai_seng-1, thomapyrin, kleenex, jus-rol, paralen, music_man-2, and 2972 more.
Images in the LogoDet-3K dataset have bounding box annotations. There are 4 (0% of the total) unlabeled images (i.e. without annotations). There are no pre-defined train/val/test splits in the dataset. Alternatively, the dataset could be split into 9 root-categories: food (53350 images), clothes (31266 images), necessities (24822 images), others (15513 images), transportation (10445 images), electronic (9675 images), leisure (5685 images), sports (3953 images), and medical (3945 images). The dataset was released in 2020 by the Shandong Normal University, China and Chinese Academy of Sciences.
Here is a visualized example for randomly selected sample classes:
Explore #
LogoDet-3K dataset has 158654 images. Click on one of the examples below or open "Explore" tool anytime you need to view dataset images with annotations. This tool has extended visualization capabilities like zoom, translation, objects table, custom filters and more. Hover the mouse over the images to hide or show annotations.
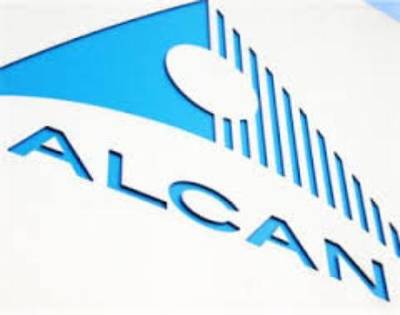
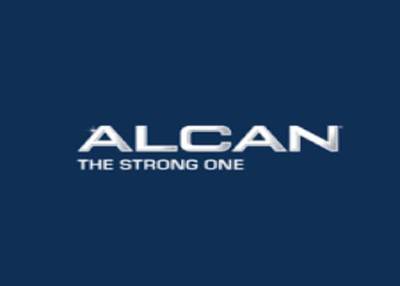
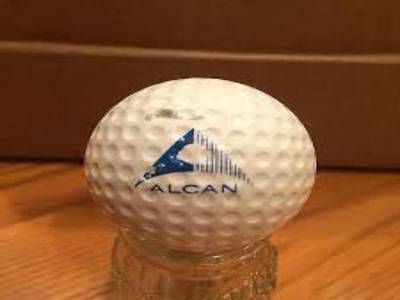
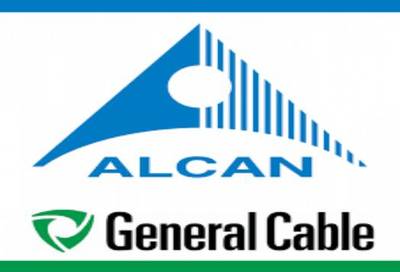
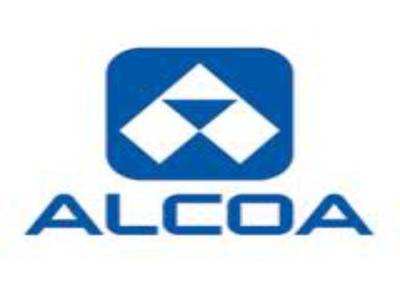
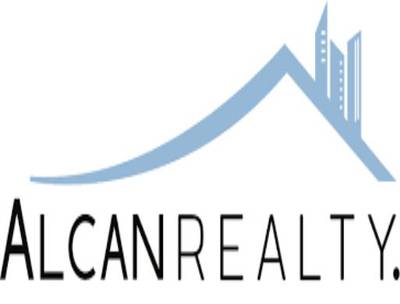
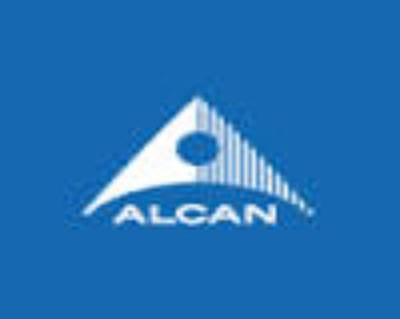
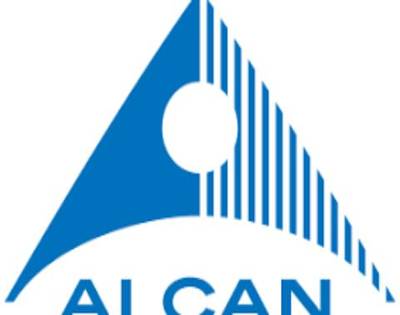

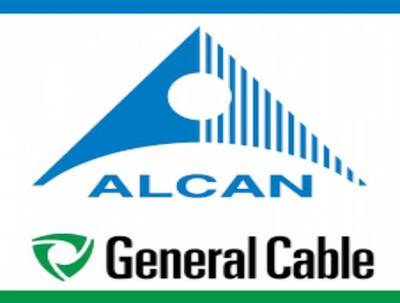
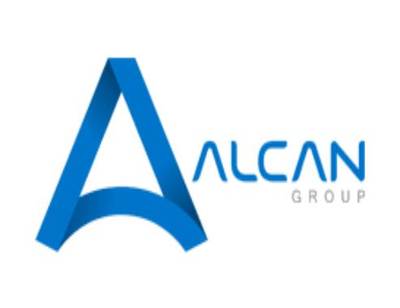
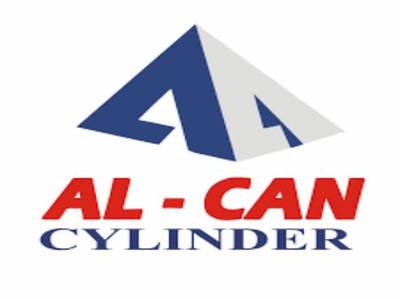
Class balance #
There are 3000 annotation classes in the dataset. Find the general statistics and balances for every class in the table below. Click any row to preview images that have labels of the selected class. Sort by column to find the most rare or prevalent classes.
Class ã…¤ | Images ã…¤ | Objects ã…¤ | Count on image average | Area on image average |
---|---|---|---|---|
lexus-1âž” rectangle | 426 | 466 | 1.09 | 28.49% |
avery_dennison-2âž” rectangle | 352 | 532 | 1.51 | 17.94% |
alpinestars-2âž” rectangle | 328 | 508 | 1.55 | 34.77% |
new_balance-1âž” rectangle | 308 | 328 | 1.06 | 30.82% |
76âž” rectangle | 302 | 302 | 1 | 15.68% |
maybach-1âž” rectangle | 294 | 310 | 1.05 | 32.92% |
mclarenâž” rectangle | 252 | 258 | 1.02 | 22.95% |
kichesippi-2âž” rectangle | 250 | 260 | 1.04 | 10% |
lexus-2âž” rectangle | 250 | 270 | 1.08 | 18.27% |
zendiumâž” rectangle | 249 | 312 | 1.25 | 11.55% |
Images #
Explore every single image in the dataset with respect to the number of annotations of each class it has. Click a row to preview selected image. Sort by any column to find anomalies and edge cases. Use horizontal scroll if the table has many columns for a large number of classes in the dataset.
Class sizes #
The table below gives various size properties of objects for every class. Click a row to see the image with annotations of the selected class. Sort columns to find classes with the smallest or largest objects or understand the size differences between classes.
Class | Object count | Avg area | Max area | Min area | Min height | Min height | Max height | Max height | Avg height | Avg height | Min width | Min width | Max width | Max width |
---|---|---|---|---|---|---|---|---|---|---|---|---|---|---|
avery_dennison-2 rectangle | 532 | 11.88% | 44.36% | 1.17% | 31px | 7.93% | 390px | 99.74% | 119px | 31.53% | 46px | 9.02% | 385px | 76.85% |
alpinestars-2 rectangle | 508 | 23.29% | 71.28% | 2.93% | 30px | 7.53% | 393px | 98.95% | 121px | 31.75% | 105px | 27.48% | 520px | 99.81% |
lexus-1 rectangle | 466 | 26.04% | 88.39% | 0.71% | 38px | 10.03% | 384px | 98.97% | 180px | 47.21% | 32px | 6.56% | 512px | 99.71% |
violet_crumble rectangle | 414 | 12.17% | 79.47% | 0.72% | 16px | 4.21% | 315px | 88.73% | 90px | 21.85% | 26px | 5.3% | 517px | 99.81% |
new_balance-1 rectangle | 328 | 28.94% | 99.55% | 1.57% | 27px | 7.18% | 395px | 99.75% | 176px | 46.22% | 81px | 16.14% | 510px | 99.8% |
auxx-2 rectangle | 327 | 6.92% | 52.51% | 0.43% | 18px | 4.31% | 349px | 99.71% | 72px | 18.89% | 46px | 9% | 475px | 97.74% |
biggby_coffee rectangle | 320 | 7.64% | 88.34% | 0.25% | 16px | 3.52% | 377px | 98.43% | 101px | 26.01% | 16px | 3.07% | 464px | 89.75% |
zendium rectangle | 312 | 9.23% | 64.68% | 1.13% | 26px | 5.5% | 308px | 69.25% | 99px | 25.32% | 22px | 4.35% | 509px | 99.8% |
bacardi rectangle | 312 | 18.81% | 98.95% | 1.01% | 29px | 7.27% | 424px | 99.74% | 138px | 36.09% | 55px | 13.92% | 504px | 99.8% |
maybach-1 rectangle | 310 | 31.22% | 85.7% | 2.26% | 69px | 17.6% | 376px | 96.63% | 201px | 52.22% | 59px | 11.73% | 507px | 98.64% |
Spatial Heatmap #
The heatmaps below give the spatial distributions of all objects for every class. These visualizations provide insights into the most probable and rare object locations on the image. It helps analyze objects' placements in a dataset.

Objects #
Table contains all 100205 objects. Click a row to preview an image with annotations, and use search or pagination to navigate. Sort columns to find outliers in the dataset.
Object ID ã…¤ | Class ã…¤ | Image name click row to open | Image size height x width | Height ã…¤ | Height ã…¤ | Width ã…¤ | Width ã…¤ | Area ã…¤ |
---|---|---|---|---|---|---|---|---|
1âž” | embraer rectangle | Embraer_46.jpg | 350 x 495 | 174px | 49.71% | 317px | 64.04% | 31.84% |
2âž” | cafe_du_monde rectangle | Cafe du Monde_122.jpg | 373 x 489 | 63px | 16.89% | 195px | 39.88% | 6.74% |
3âž” | ermenegildo_zegna rectangle | Ermenegildo Zegna_42.jpg | 378 x 484 | 97px | 25.66% | 462px | 95.45% | 24.49% |
4âž” | taixiang rectangle | taixiang_23.jpg | 397 x 521 | 83px | 20.91% | 136px | 26.1% | 5.46% |
5âž” | pakistan_state rectangle | pakistan state_28.jpg | 362 x 489 | 249px | 68.78% | 230px | 47.03% | 32.35% |
6âž” | pakistan_state rectangle | pakistan state_28.jpg | 362 x 489 | 74px | 20.44% | 271px | 55.42% | 11.33% |
7âž” | timex rectangle | timex_142.jpg | 389 x 517 | 186px | 47.81% | 286px | 55.32% | 26.45% |
8âž” | count_chocula rectangle | Count Chocula_83.jpg | 524 x 361 | 124px | 23.66% | 184px | 50.97% | 12.06% |
9âž” | laura_secord_chocolates rectangle | laura secord chocolates_67.jpg | 378 x 519 | 52px | 13.76% | 202px | 38.92% | 5.35% |
10âž” | gigo rectangle | gigo_46.jpg | 380 x 487 | 55px | 14.47% | 72px | 14.78% | 2.14% |
License #
License is unknown for the LogoDet-3K dataset.
Citation #
If you make use of the LogoDet-3K data, please cite the following reference:
@dataset{LogoDet-3K,
author={Jing Wang and Weiqing Min and Sujuan Hou and Shengnan Ma and Yuanjie Zheng and Shuqiang Jiang},
title={LogoDet-3K},
year={2020},
url={https://github.com/Wangjing1551/LogoDet-3K-Dataset}
}
If you are happy with Dataset Ninja and use provided visualizations and tools in your work, please cite us:
@misc{ visualization-tools-for-logodet-3k-dataset,
title = { Visualization Tools for LogoDet-3K Dataset },
type = { Computer Vision Tools },
author = { Dataset Ninja },
howpublished = { \url{ https://datasetninja.com/logodet-3k } },
url = { https://datasetninja.com/logodet-3k },
journal = { Dataset Ninja },
publisher = { Dataset Ninja },
year = { 2025 },
month = { apr },
note = { visited on 2025-04-26 },
}
Download #
Please visit dataset homepage to download the data.
Disclaimer #
Our gal from the legal dep told us we need to post this:
Dataset Ninja provides visualizations and statistics for some datasets that can be found online and can be downloaded by general audience. Dataset Ninja is not a dataset hosting platform and can only be used for informational purposes. The platform does not claim any rights for the original content, including images, videos, annotations and descriptions. Joint publishing is prohibited.
You take full responsibility when you use datasets presented at Dataset Ninja, as well as other information, including visualizations and statistics we provide. You are in charge of compliance with any dataset license and all other permissions. You are required to navigate datasets homepage and make sure that you can use it. In case of any questions, get in touch with us at hello@datasetninja.com.