Introduction #
The MFVT Dataset for Real-time Masked Facial Detection dataset is a groundbreaking resource designed to rectify the limitations of existing datasets in the realm of Real-time Masked Facial Detection. Comprising 13,507 meticulously annotated images, it integrates authors’ unique mask-wearing conventions to classify individuals into three distinct categories: ok, none, and wrong, offering a comprehensive and invaluable tool for advancing the field.
Although there have been a number of Masked Facial Detection datasets published, they all have their own limitations, from having different mask-wearing conventions to careless labelling and classes imbalanced. Therefore, a new dataset to overcome those problems, with correct, clear conventions on wearing masks and carefully annotated for research purposes is very essential. That motivates authors to build the MFVT dataset.
The MFVT dataset built from two datasets PWMFD and FMLD, along with some images collected from the Internet.
The reason authors chose those two datasets is that their images have been carefully selected by the authors from previous datasets such as MAFA and Wider Face. However, the mask-wearing conventions of those two datasets were different and they also differed from authors’, so they re-annotated the entire dataset.
Authors follow WHO’s advice on how to wear masks and the types of masks that can be worn during the COVID-19 epidemic to annotate the MFVT dataset. All the faces, or objects, in authors’ dataset belong to one of three classes: properly wearing (ok), incorrectly wearing (wrong) and not wearing mask (none). Details on these classes are given below:
- Class ok: Firstly, about the type of mask that can be worn, authors consider cases that not only be medical masks but also can be something like fabric masks, scarves, buffs, or hijabs. Authors will call them “masks”. According to WHO’s advice, authors should wear a mask that could cover nose, mouth and chin. Therefore, if a person uses masks with “proper thickness” and wears them in that way, authors will annotate them as OK. For image data, they consider a mask with proper thickness when they can not see the part of the face occluded by that mask.

Example images for class ok. (a) - (d) Faces with medical masks. (e) - (f) Using scarves to cover nose, mouth and chin. (g) - (j) Wearing fabric masks properly. (k) - (l) Illustrations for hijabs.
- Class wrong: Authors regard that a presented mask that does not cover the nose, mouth, and chin is the wrong way to wear a face mask. In addition, a mask worn without being pulled high enough is marked as an improperly wearing way (also, in a perspective, these cases are difficult for models to learn because it is easy to confuse between wrong and ok). Especially, masks with small holes (such as wool masks) or wearing the mask on one side do not provide good protection for health during the pandemic, so authors also consider these as incorrect.

Example images for class wrong. (a) - © Not intending to use masks to protect from the virus. (d) - (f) The nose, mouth or chin is not covered. (g) - (h) The masks are not pulled high enough. (i) - (j) Small holes on wool masks. (k) - (l) The masks are worn just in one side.
- Class none: In the COVID-19 pandemic, wearing too thin masks (such as mesh face veils, or satin face veils) or using hands, arms to cover the face are quite useless in preventing the virus from spreading. Therefore, these cases will be considered as not wearing masks. Authors also suppose that people using a mask to conceal the face but not wearing it on, or using kinds of stuff such as phones, papers, cups, books, placards, etc. to hide their face are not acceptable and will be annotated as none. Specially, when people use a scarf but do not intend to pull it up to cover the face (the scarf does not hide the mouth), authors assume that they do not intend to use it as a way to protect from viruses. Furthermore, authors attempt to consider the scene that people fake the face mask by pulling up a collar to cover the face. These cases are deemed as not wearing masks.

Example for class none. (a) Normal face. (b) - © Using masks to conceal the face but not wearing them on. (d) - (h) Using kinds of stuff such as phones, papers, cups, books, placards to hide the face. (i) Pulling up a collar to cover the face. (j) Wearing too thin masks. (k) - (l) Using hands or arms to cover the face.
The PWMFD dataset primarily focuses on medical masks, and the authors collected numerous medical mask-related images from this dataset. Additionally, they included some images of fabric masks or scarves in their selection. From the original 6340 training images and 1633 test images in the PWMFD dataset, the authors chose 2473 images for the training set and 500 images for the test set of the MFVT dataset.
Regarding the FMLD dataset, it contains a wide variety of images covering different cases. While the FMLD dataset includes many images labeled as “NONE,” which were derived from the Wider Face dataset, the authors decided that these “NONE” images didn’t significantly contribute to the Masked Facial Detection problem. Therefore, they selected only a few of these “NONE” images for the MFVT dataset. The cases mentioned in the mask conventions align with the FMLD dataset, albeit with different annotations. This makes the FMLD dataset a reliable source of images. From the original 34,784 training images in the FMLD dataset, the authors selected 6,182 images, and from the 7,152 test images, they selected 850 images for the MFVT dataset.
It’s important to note that during the process of image selection from the two datasets, the authors may come across images that are present in both datasets. In some cases, an image in the training set of one dataset may appear in the testing set of the other dataset. The authors carefully reviewed and removed such duplicate images.
With two training sets selected from the PWMFD and FMLD datasets, the authors randomly selected a small portion from each set to create two validation sets, which they then merged to obtain the overall validation set for the MFVT dataset. The training and testing sets for the authors’ dataset are also composed of merged selections from the two respective sets. Furthermore, the authors augmented the training set by adding background images (images without objects) to enhance the robustness of the models trained on the MFVT dataset. They also included a few “Extra” images representing unusual cases in both the training and testing sets.
Summary #
MFVT Dataset for Real-time Masked Facial Detection is a dataset for an object detection task. It is used in the surveillance industry.
The dataset consists of 13507 images with 26201 labeled objects belonging to 3 different classes including ok, none, and wrong.
Images in the MFVT dataset have bounding box annotations. There are 220 (2% of the total) unlabeled images (i.e. without annotations). There are 3 splits in the dataset: train (10445 images), valid (1706 images), and test (1356 images). The dataset was released in 2022 by the University of Science - VNUHCM, Vietnam and Vietnam National University.
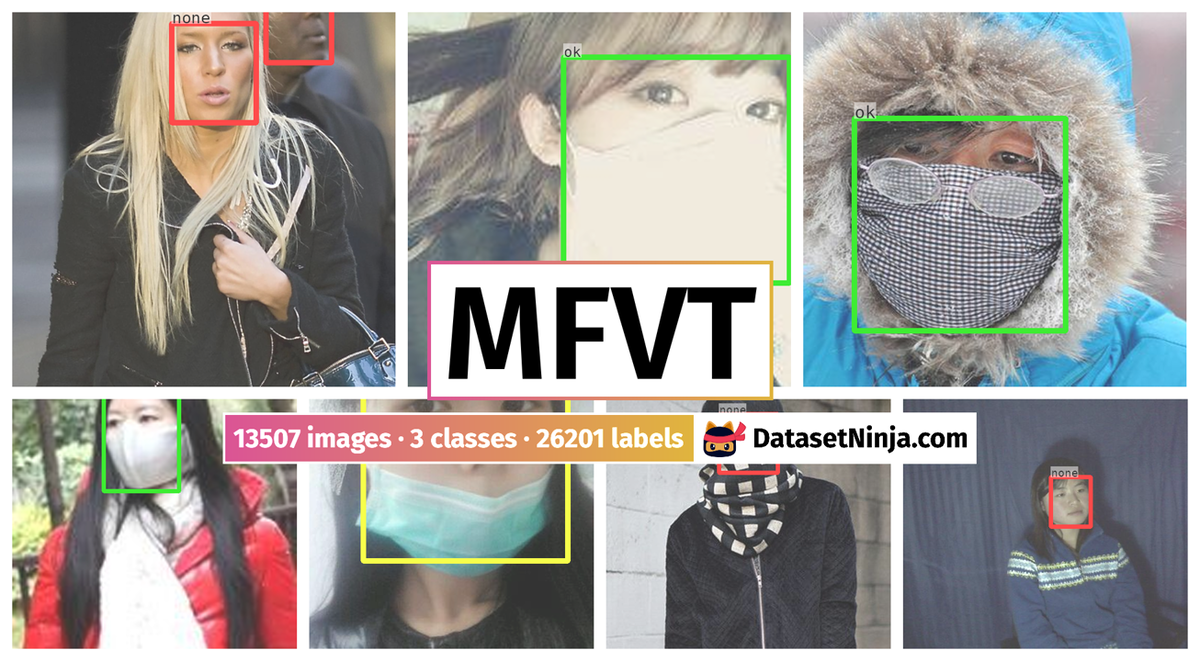
Explore #
MFVT dataset has 13507 images. Click on one of the examples below or open "Explore" tool anytime you need to view dataset images with annotations. This tool has extended visualization capabilities like zoom, translation, objects table, custom filters and more. Hover the mouse over the images to hide or show annotations.
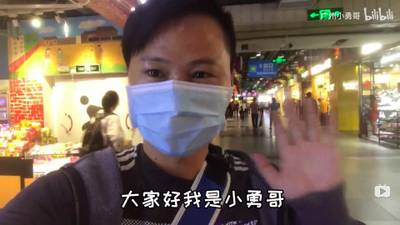
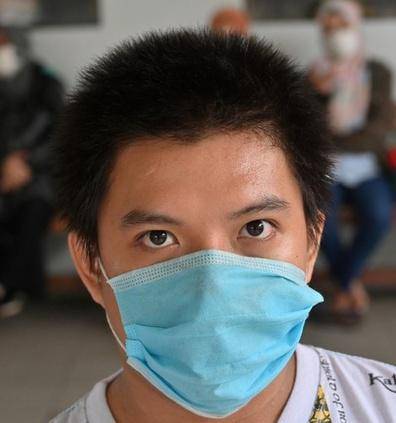
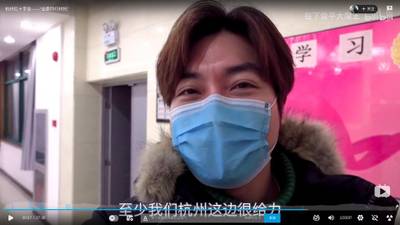
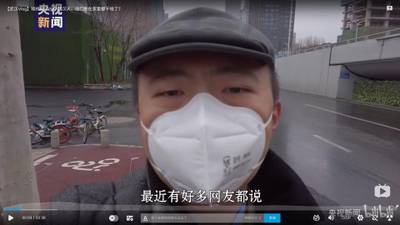
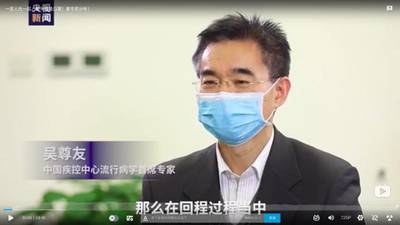
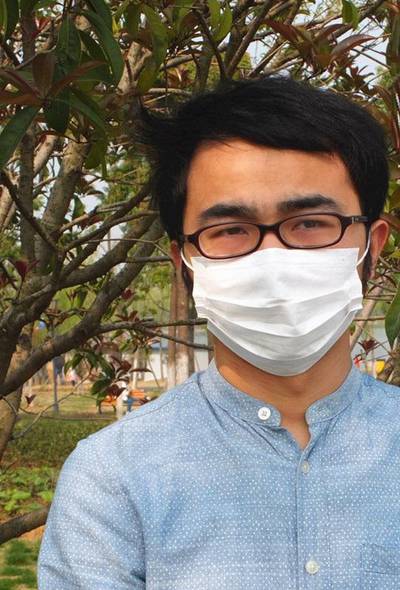
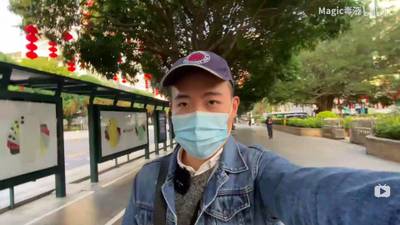
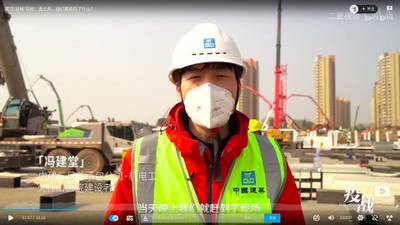
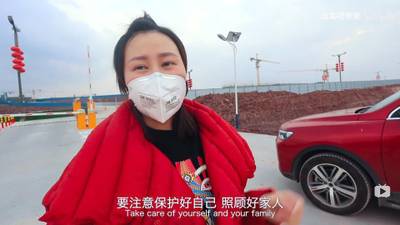
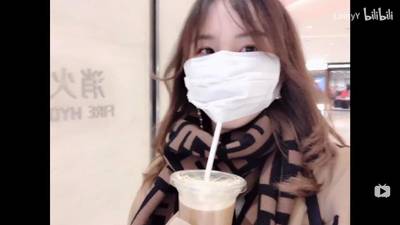
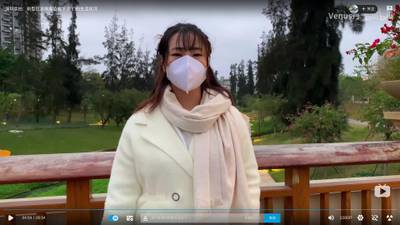
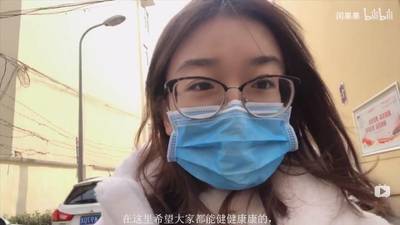
Class balance #
There are 3 annotation classes in the dataset. Find the general statistics and balances for every class in the table below. Click any row to preview images that have labels of the selected class. Sort by column to find the most rare or prevalent classes.
Class ㅤ | Images ㅤ | Objects ㅤ | Count on image average | Area on image average |
---|---|---|---|---|
ok➔ rectangle | 6610 | 12180 | 1.84 | 13.11% |
none➔ rectangle | 4982 | 8521 | 1.71 | 9.33% |
wrong➔ rectangle | 4803 | 5500 | 1.15 | 13.58% |
Co-occurrence matrix #
Co-occurrence matrix is an extremely valuable tool that shows you the images for every pair of classes: how many images have objects of both classes at the same time. If you click any cell, you will see those images. We added the tooltip with an explanation for every cell for your convenience, just hover the mouse over a cell to preview the description.
Images #
Explore every single image in the dataset with respect to the number of annotations of each class it has. Click a row to preview selected image. Sort by any column to find anomalies and edge cases. Use horizontal scroll if the table has many columns for a large number of classes in the dataset.
Object distribution #
Interactive heatmap chart for every class with object distribution shows how many images are in the dataset with a certain number of objects of a specific class. Users can click cell and see the list of all corresponding images.
Class sizes #
The table below gives various size properties of objects for every class. Click a row to see the image with annotations of the selected class. Sort columns to find classes with the smallest or largest objects or understand the size differences between classes.
Class | Object count | Avg area | Max area | Min area | Min height | Min height | Max height | Max height | Avg height | Avg height | Min width | Min width | Max width | Max width |
---|---|---|---|---|---|---|---|---|---|---|---|---|---|---|
ok rectangle | 12180 | 7.13% | 100% | 0.02% | 2px | 0.89% | 2179px | 100% | 115px | 23.66% | 5px | 0.88% | 1954px | 100% |
none rectangle | 8521 | 5.46% | 87.29% | 0.01% | 3px | 1.17% | 1821px | 100% | 107px | 20.57% | 2px | 0.36% | 1821px | 99.44% |
wrong rectangle | 5500 | 11.86% | 98.89% | 0.05% | 9px | 2.3% | 2554px | 99.44% | 177px | 31.02% | 6px | 1.6% | 1743px | 100% |
Spatial Heatmap #
The heatmaps below give the spatial distributions of all objects for every class. These visualizations provide insights into the most probable and rare object locations on the image. It helps analyze objects' placements in a dataset.
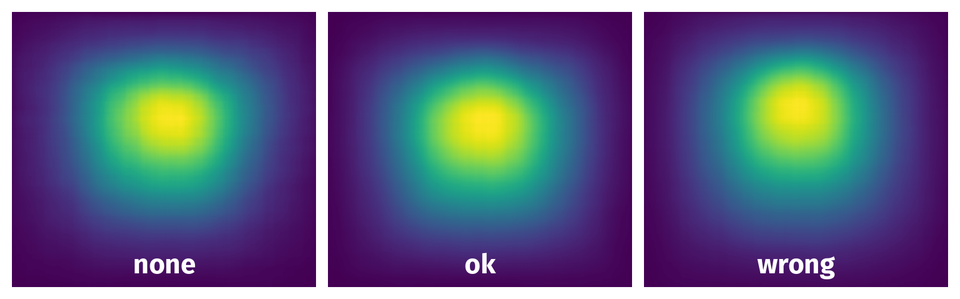
Objects #
Table contains all 26201 objects. Click a row to preview an image with annotations, and use search or pagination to navigate. Sort columns to find outliers in the dataset.
Object ID ㅤ | Class ㅤ | Image name click row to open | Image size height x width | Height ㅤ | Height ㅤ | Width ㅤ | Width ㅤ | Area ㅤ |
---|---|---|---|---|---|---|---|---|
1➔ | ok rectangle | vt_test_00048.jpg | 567 x 480 | 316px | 55.73% | 260px | 54.17% | 30.19% |
2➔ | none rectangle | vt_test_00628.jpg | 461 x 333 | 83px | 18% | 83px | 24.92% | 4.49% |
3➔ | ok rectangle | vt_test_00980.jpg | 176 x 200 | 68px | 38.64% | 69px | 34.5% | 13.33% |
4➔ | none rectangle | vt_test_00713.jpg | 310 x 310 | 100px | 32.26% | 83px | 26.77% | 8.64% |
5➔ | none rectangle | vt_test_00817.jpg | 253 x 550 | 57px | 22.53% | 52px | 9.45% | 2.13% |
6➔ | ok rectangle | vt_test_00136.jpg | 220 x 220 | 115px | 52.27% | 100px | 45.45% | 23.76% |
7➔ | ok rectangle | vt_test_01335.jpg | 326 x 580 | 125px | 38.34% | 125px | 21.55% | 8.26% |
8➔ | none rectangle | vt_test_00594.jpg | 826 x 690 | 207px | 25.06% | 161px | 23.33% | 5.85% |
9➔ | none rectangle | vt_test_00386.jpg | 268 x 202 | 121px | 45.15% | 84px | 41.58% | 18.77% |
10➔ | ok rectangle | vt_test_01161.jpg | 800 x 800 | 297px | 37.12% | 296px | 37% | 13.74% |
License #
License is unknown for the MFVT Dataset for Real-time Masked Facial Detection dataset.
Citation #
If you make use of the MFVT data, please cite the following reference:
@dataset{MFVT,
author={Trong-Vu Hoang and Huu-Thien Tran and Trong-Le Do},
title={MFVT Dataset for Real-time Masked Facial Detection},
year={2022},
url={https://www.kaggle.com/datasets/trngvhong/mfvt-dataset}
}
If you are happy with Dataset Ninja and use provided visualizations and tools in your work, please cite us:
@misc{ visualization-tools-for-mfvt-dataset,
title = { Visualization Tools for MFVT Dataset },
type = { Computer Vision Tools },
author = { Dataset Ninja },
howpublished = { \url{ https://datasetninja.com/mfvt } },
url = { https://datasetninja.com/mfvt },
journal = { Dataset Ninja },
publisher = { Dataset Ninja },
year = { 2025 },
month = { apr },
note = { visited on 2025-04-03 },
}
Download #
Please visit dataset homepage to download the data.
Disclaimer #
Our gal from the legal dep told us we need to post this:
Dataset Ninja provides visualizations and statistics for some datasets that can be found online and can be downloaded by general audience. Dataset Ninja is not a dataset hosting platform and can only be used for informational purposes. The platform does not claim any rights for the original content, including images, videos, annotations and descriptions. Joint publishing is prohibited.
You take full responsibility when you use datasets presented at Dataset Ninja, as well as other information, including visualizations and statistics we provide. You are in charge of compliance with any dataset license and all other permissions. You are required to navigate datasets homepage and make sure that you can use it. In case of any questions, get in touch with us at hello@datasetninja.com.