Introduction #
The authors of the NPU-BOLT: A Dataset for Bolt Object Detection in Natural Scene Images delve into the realm of engineering structures, focusing on bolt joints, which are commonplace and pivotal. These joints are susceptible to loosening or disengagement due to extreme service environments and load factors, posing a critical challenge for structural safety and longevity. The authors acknowledge the urgent need for real-time or timely detection of such issues in practical engineering applications.
Recent years have seen the emergence of various bolt loosening detection methods utilizing deep learning and machine learning techniques. However, most of these methods rely on laboratory-captured bolt images, characterized by controlled lighting, distance, and viewing angles. Real-world bolt images, on the other hand, often exhibit complexities such as blurred edges, oblique perspectives, partial occlusion, and variable colors, rendering models trained in controlled lab conditions less accurate or ineffective.
There are three sources of image-sets: natural environment photos, photos from the internet, and the CAD renders of a simulation.
For the images captured by the authors in natural environment, the following practical conditions, different light intensity, shadow shielding, large area corrosion, bolt shedding, cracks, rain and snow weather are considered. The real images from natural scenes are captured by a Cannon 200D hand-hold camera, an Honor X10 mobile phone and a DJI AIR 2plus drone. The images of real bolt connections in sunny, cloudy, night and indoor (building) environments were collected. A variety of bolted structures are captured to cover as many as possible bolted joint types, which include bolted flange joints, pipe connections and different bolted structures from bridge steel frame, vehicle hub, construction machinery, guardrails, iron cable fixings and anchor bolts.
The reason for using the downloaded pictures from the internet is that if the images in the dataset are all from the same photographers, the photographers’ habits may reduce the diversity of the dataset. The images on the internet are generally captured by different photographers and covers many engineering objects. To include the images from internet can improve the application scope and diversity of the dataset. However, generally the pixel quality of these pictures is slightly lower than the pictures captured by the authors. And, most of the image sizes are between 500x500 and 1500x1500.
The reason to add 3D CAD images is that they usually have high resolutions and clear bolt features without background information. Beside, the view angle can be easily adjusted in the CAD model. With adding a few number of 3D CAD simulation images in the dataset, it is expected to enhance the bolt object detection accuracy.
The dataset comprises 337 samples of bolt joint images primarily obtained from the natural environment. These images encompass a wide range of data sizes, spanning from 400x400 to 6000x4000 pixels, with a total of around 1275 annotated bolt targets. The dataset categorizes the bolt targets into four classes: blur bolt (loss of camera focus), bolt head (head of the bolt), bolt nut (nut of the bolt) and bolt side (side of the bolt).
Summary #
NPU-BOLT: A Dataset for Bolt Object Detection in Natural Scene Images is a dataset for an object detection task. It is used in the construction industry, and in the engineering domain.
The dataset consists of 337 images with 1275 labeled objects belonging to 4 different classes including bolt nut, bolt head, blur bolt, and other: bolt side.
Images in the NPU-BOLT dataset have bounding box annotations. All images are labeled (i.e. with annotations). There are no pre-defined train/val/test splits in the dataset. Alternatively, the dataset could be split into 3 image-sets: captured by authors (204 images), from internet (116 images), and 3D CAD simulation (17 images). The dataset was released in 2022 by the Northwestern Polytechnical University, China.
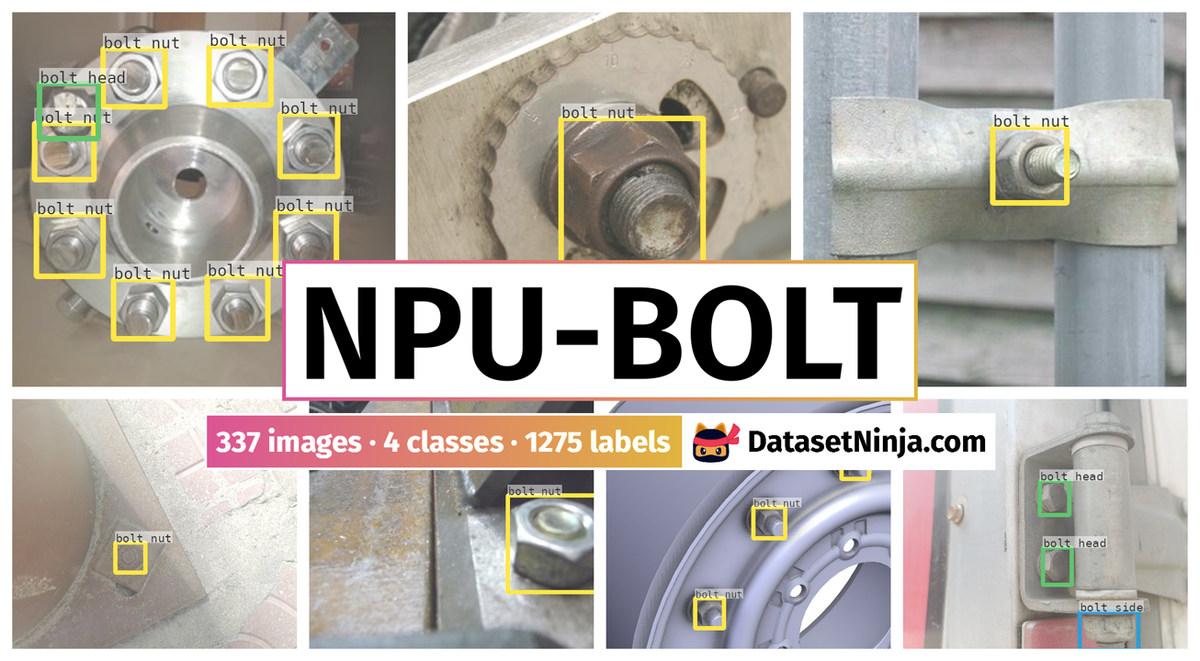
Explore #
NPU-BOLT dataset has 337 images. Click on one of the examples below or open "Explore" tool anytime you need to view dataset images with annotations. This tool has extended visualization capabilities like zoom, translation, objects table, custom filters and more. Hover the mouse over the images to hide or show annotations.
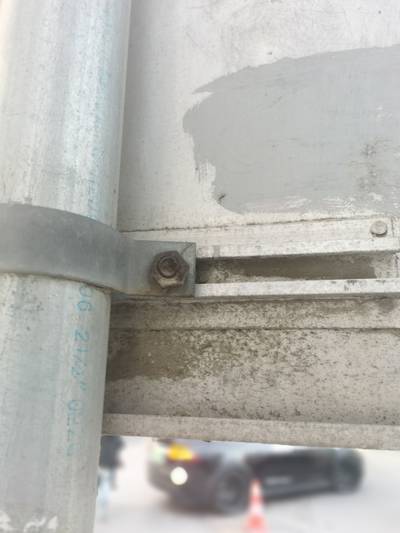
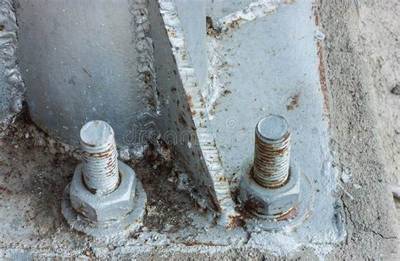
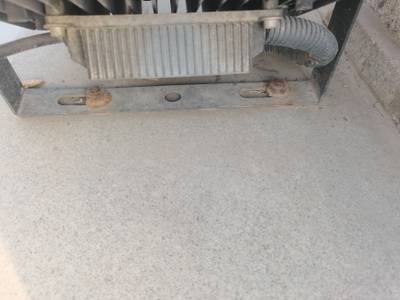
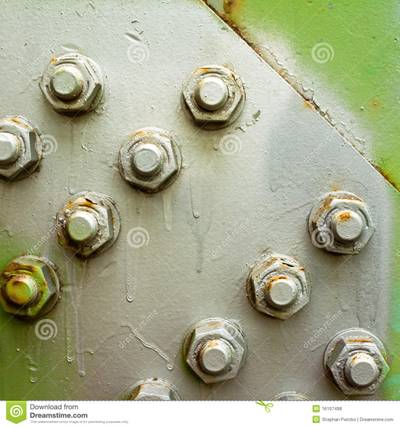
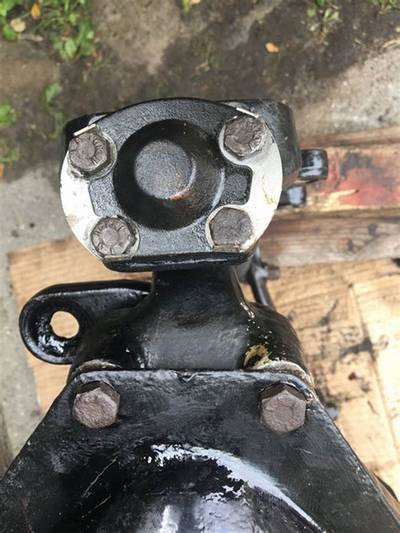
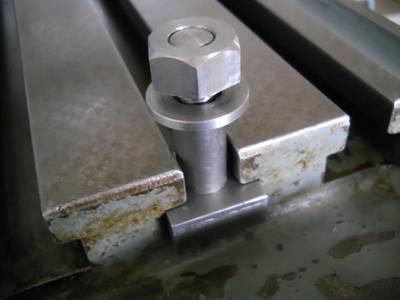
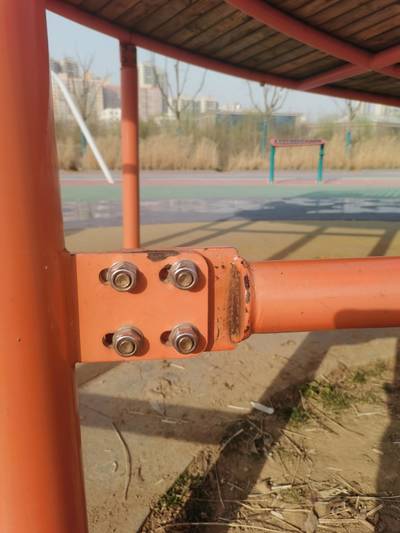

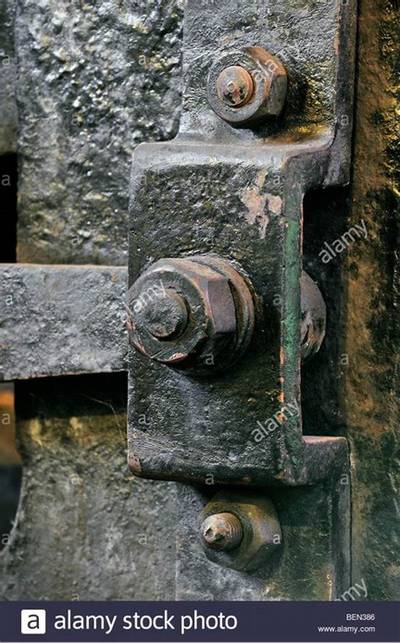
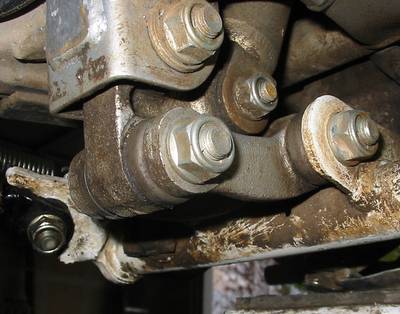
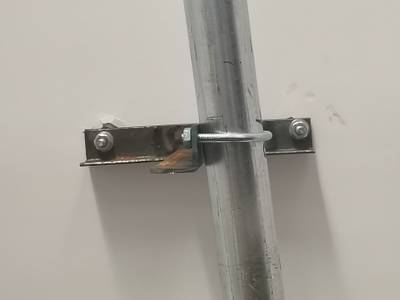
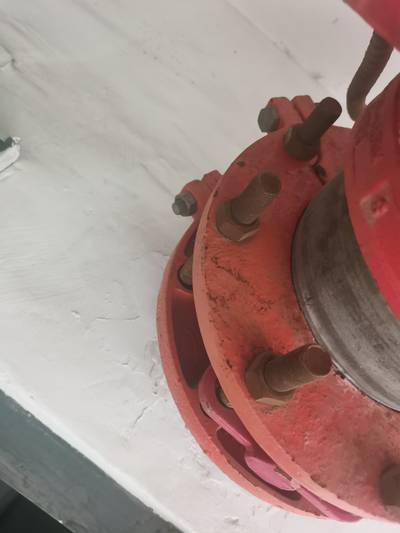
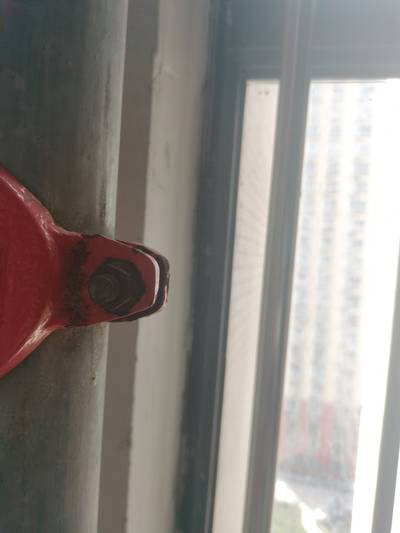
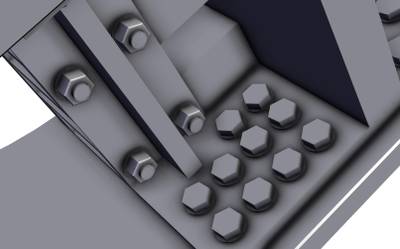
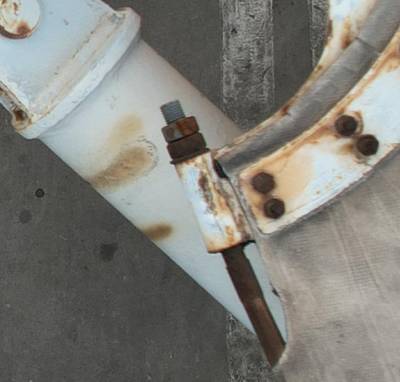
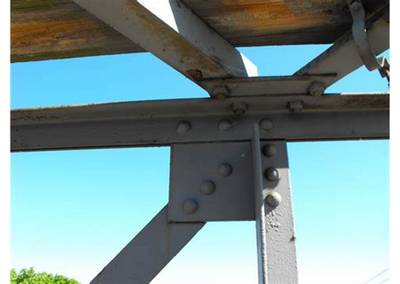
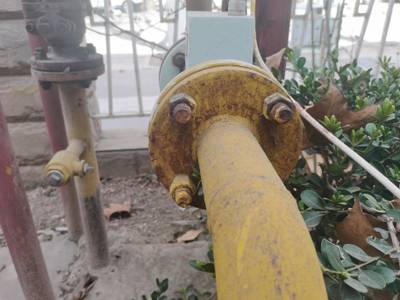
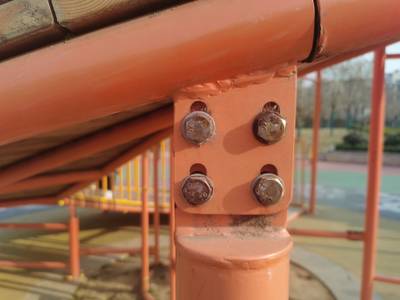
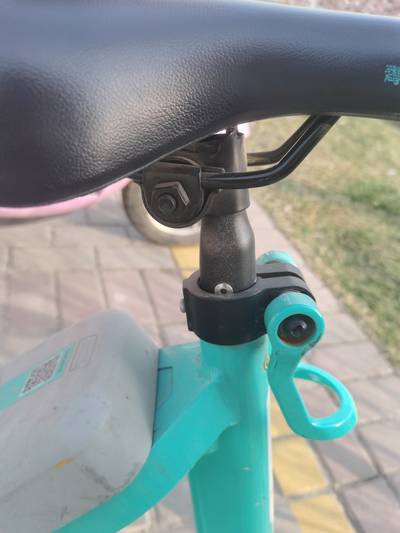
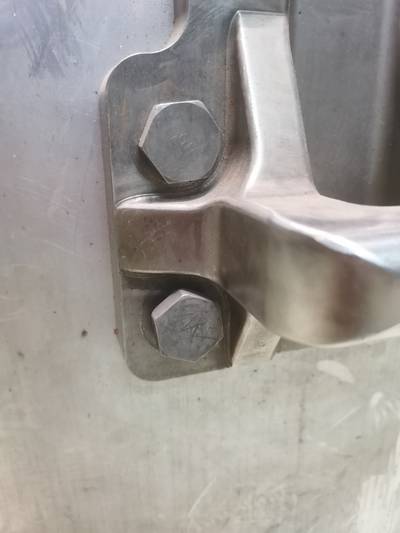
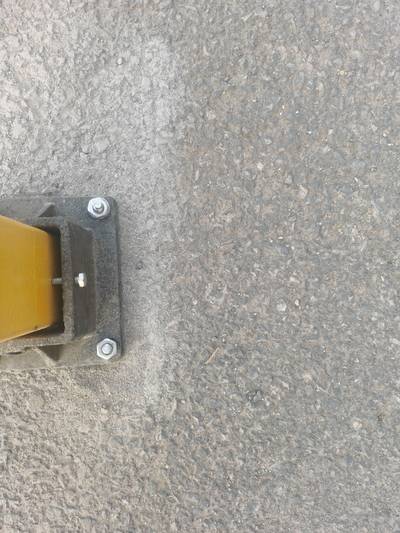
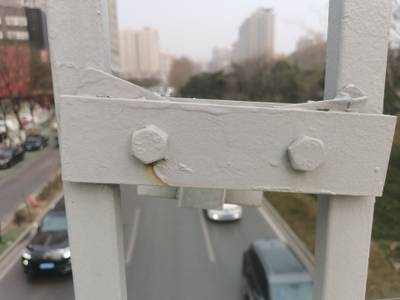
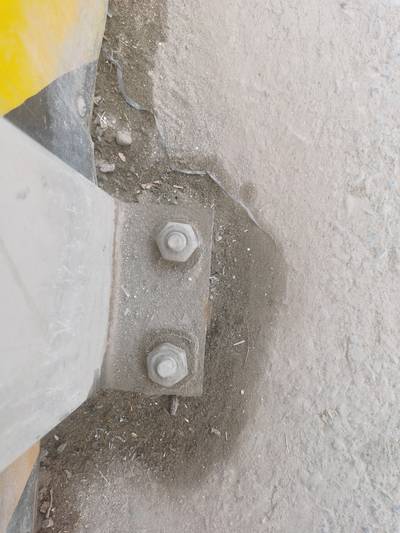

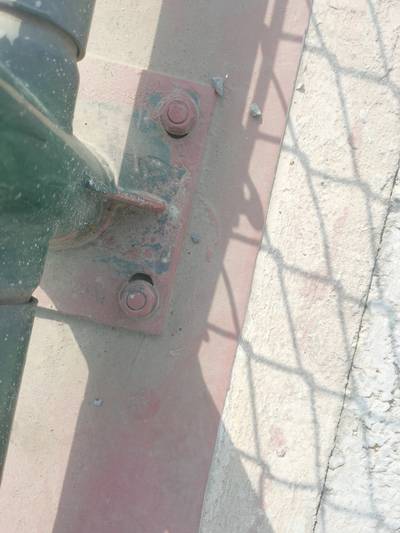
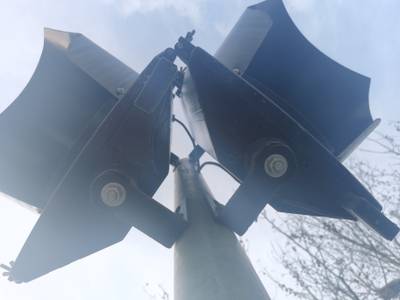
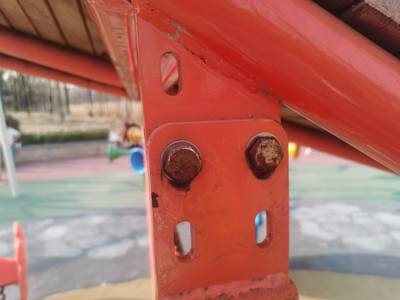
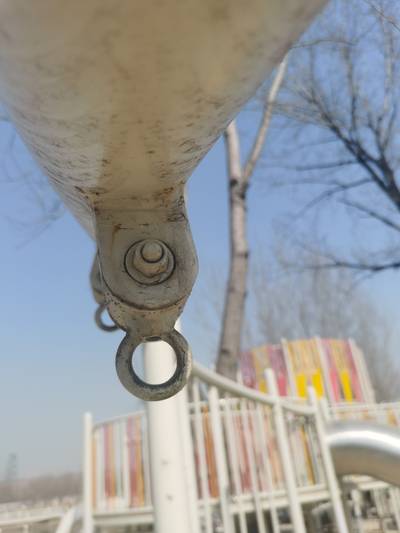
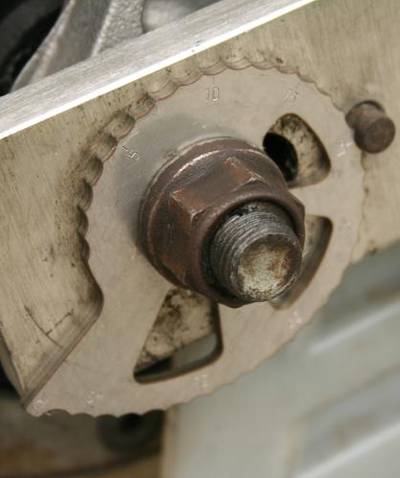
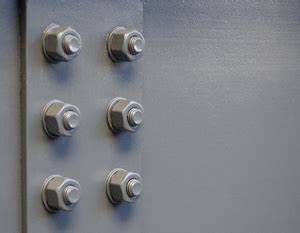
Class balance #
There are 4 annotation classes in the dataset. Find the general statistics and balances for every class in the table below. Click any row to preview images that have labels of the selected class. Sort by column to find the most rare or prevalent classes.
Class ã…¤ | Images ã…¤ | Objects ã…¤ | Count on image average | Area on image average |
---|---|---|---|---|
bolt nutâž” rectangle | 248 | 768 | 3.1 | 6.09% |
bolt headâž” rectangle | 103 | 314 | 3.05 | 2.76% |
blur boltâž” rectangle | 45 | 132 | 2.93 | 0.79% |
bolt sideâž” rectangle | 27 | 61 | 2.26 | 1.62% |
Co-occurrence matrix #
Co-occurrence matrix is an extremely valuable tool that shows you the images for every pair of classes: how many images have objects of both classes at the same time. If you click any cell, you will see those images. We added the tooltip with an explanation for every cell for your convenience, just hover the mouse over a cell to preview the description.
Images #
Explore every single image in the dataset with respect to the number of annotations of each class it has. Click a row to preview selected image. Sort by any column to find anomalies and edge cases. Use horizontal scroll if the table has many columns for a large number of classes in the dataset.
Object distribution #
Interactive heatmap chart for every class with object distribution shows how many images are in the dataset with a certain number of objects of a specific class. Users can click cell and see the list of all corresponding images.
Class sizes #
The table below gives various size properties of objects for every class. Click a row to see the image with annotations of the selected class. Sort columns to find classes with the smallest or largest objects or understand the size differences between classes.
Class | Object count | Avg area | Max area | Min area | Min height | Min height | Max height | Max height | Avg height | Avg height | Min width | Min width | Max width | Max width |
---|---|---|---|---|---|---|---|---|---|---|---|---|---|---|
bolt nut rectangle | 768 | 1.97% | 70.17% | 0.02% | 22px | 1.34% | 1935px | 81.84% | 176px | 12.17% | 18px | 1.68% | 1920px | 85.75% |
bolt head rectangle | 314 | 0.91% | 13.4% | 0.03% | 24px | 1.54% | 851px | 43.75% | 158px | 8.8% | 23px | 1.67% | 951px | 34.76% |
blur bolt rectangle | 132 | 0.27% | 2.88% | 0.02% | 20px | 1.07% | 344px | 21.67% | 72px | 5.15% | 20px | 1.61% | 324px | 13.28% |
bolt side rectangle | 61 | 0.72% | 6.78% | 0.04% | 16px | 1.29% | 374px | 34.65% | 130px | 7.9% | 18px | 1.53% | 566px | 20.69% |
Spatial Heatmap #
The heatmaps below give the spatial distributions of all objects for every class. These visualizations provide insights into the most probable and rare object locations on the image. It helps analyze objects' placements in a dataset.
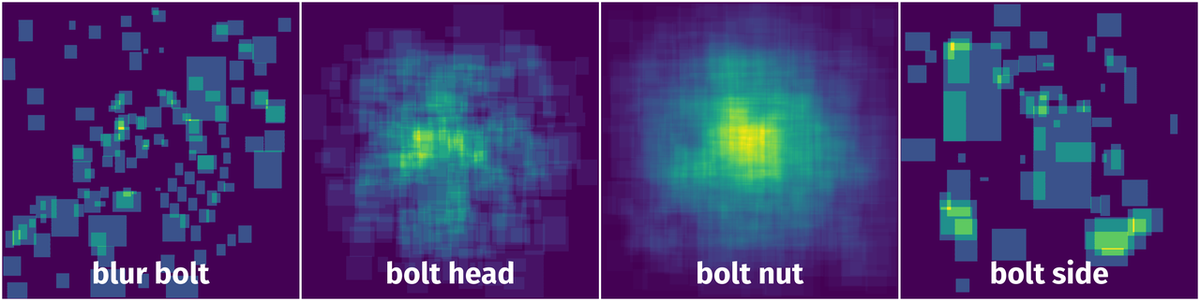
Objects #
Table contains all 1275 objects. Click a row to preview an image with annotations, and use search or pagination to navigate. Sort columns to find outliers in the dataset.
Object ID ã…¤ | Class ã…¤ | Image name click row to open | Image size height x width | Height ã…¤ | Height ã…¤ | Width ã…¤ | Width ã…¤ | Area ã…¤ |
---|---|---|---|---|---|---|---|---|
1âž” | bolt nut rectangle | AUT-0160.jpg | 2736 x 3648 | 213px | 7.79% | 203px | 5.56% | 0.43% |
2âž” | bolt nut rectangle | AUT-0160.jpg | 2736 x 3648 | 226px | 8.26% | 229px | 6.28% | 0.52% |
3âž” | bolt nut rectangle | AUT-0160.jpg | 2736 x 3648 | 232px | 8.48% | 230px | 6.3% | 0.53% |
4âž” | bolt nut rectangle | AUT-0132.jpg | 2736 x 3648 | 267px | 9.76% | 275px | 7.54% | 0.74% |
5âž” | bolt nut rectangle | AUT-0132.jpg | 2736 x 3648 | 281px | 10.27% | 293px | 8.03% | 0.82% |
6âž” | bolt nut rectangle | WEB-0083.jpg | 480 x 640 | 98px | 20.42% | 96px | 15% | 3.06% |
7âž” | bolt nut rectangle | AUT-0109.jpg | 2736 x 3648 | 247px | 9.03% | 270px | 7.4% | 0.67% |
8âž” | bolt head rectangle | AUT-0109.jpg | 2736 x 3648 | 133px | 4.86% | 129px | 3.54% | 0.17% |
9âž” | bolt head rectangle | AUT-0109.jpg | 2736 x 3648 | 139px | 5.08% | 138px | 3.78% | 0.19% |
10âž” | bolt nut rectangle | WEB-0112.jpg | 800 x 600 | 350px | 43.75% | 313px | 52.17% | 22.82% |
License #
Citation #
If you make use of the NPU-BOLT data, please cite the following reference:
@dataset{NPU-BOLT,
author={Yadian Zhao and Zhenglin Yang and Chao Xu},
title={NPU-BOLT: A Dataset for Bolt Object Detection in Natural Scene Images},
year={2022},
url={https://www.kaggle.com/datasets/yartinz/npu-bolt}
}
If you are happy with Dataset Ninja and use provided visualizations and tools in your work, please cite us:
@misc{ visualization-tools-for-npu-bolt-dataset,
title = { Visualization Tools for NPU-BOLT Dataset },
type = { Computer Vision Tools },
author = { Dataset Ninja },
howpublished = { \url{ https://datasetninja.com/npu-bolt } },
url = { https://datasetninja.com/npu-bolt },
journal = { Dataset Ninja },
publisher = { Dataset Ninja },
year = { 2025 },
month = { apr },
note = { visited on 2025-04-03 },
}
Download #
Dataset NPU-BOLT can be downloaded in Supervisely format:
As an alternative, it can be downloaded with dataset-tools package:
pip install --upgrade dataset-tools
… using following python code:
import dataset_tools as dtools
dtools.download(dataset='NPU-BOLT', dst_dir='~/dataset-ninja/')
Make sure not to overlook the python code example available on the Supervisely Developer Portal. It will give you a clear idea of how to effortlessly work with the downloaded dataset.
The data in original format can be downloaded here.
Disclaimer #
Our gal from the legal dep told us we need to post this:
Dataset Ninja provides visualizations and statistics for some datasets that can be found online and can be downloaded by general audience. Dataset Ninja is not a dataset hosting platform and can only be used for informational purposes. The platform does not claim any rights for the original content, including images, videos, annotations and descriptions. Joint publishing is prohibited.
You take full responsibility when you use datasets presented at Dataset Ninja, as well as other information, including visualizations and statistics we provide. You are in charge of compliance with any dataset license and all other permissions. You are required to navigate datasets homepage and make sure that you can use it. In case of any questions, get in touch with us at hello@datasetninja.com.