Introduction #
The authors of the Photometric Stereo Training Data Set with Annotated Leaf Masks recognized the necessity for accurate and quantitative methods to predict plant growth in dynamic natural environments, a crucial requirement in the face of changing global climate and increasing population. They highlighted the significance of computer vision in driving advancements in plant phenotyping for both research and agriculture applications. To this end, the authors focused on developing imaging technologies for comprehensive plant growth assessment.
A strong indicator of plant yield is above-ground growth, particularly 3D imaging of vegetative growth, which has garnered substantial attention in phenotyping research. They distinguished between passive and active 3D imaging approaches for plant architecture. Passive methods capture plant structures without introducing new energy, employing techniques like multi-view stereo, structure from motion, and light-field cameras. Active 3D imaging methods, like structured light and laser scanners, emit energy to overcome challenges faced by passive methods. Despite the improvements offered by both passive and active 3D imaging, existing techniques still lack in various crucial aspects such as speed, portability, spatial resolution, and cost-effectiveness.
The authors then introduced the concept of photometric stereo (PS), an active imaging technique that offers high image resolutions and rapid capture speeds at a low cost. This technique relies on capturing images under controlled, varied, and directional illumination to generate dense surface normal maps. They noted its potential for encoding complex 3D morphology, essential for recognizing and quantifying growth patterns. The authors presented their novel imaging system called PS-Plant, which leverages photometric stereo to monitor the growth and development of Arabidopsis in three dimensions.
The dataset comprises 21 Arabidopsis thaliana (L. Heynh. Col-0, wild type) plants grown in a growth cabinet at 22° under 150 µmol photons m-2 s-1 in 12/12 hr light/dark cycles. The dataset represents plants at varying time intervals (12 to 48 hours) from 11 and 24 days after germination.
Each Arabidopsis plant from the raw data was individually cropped by specifying the rosette centroid location and the size of the region of interest. All crops have the same resolution (650x560 pixels). The cropped images were named as the parent directory with a _X suffix, where X is the plant number in the tray. The images provided are:
- Annotated image layers — Individual leaf labels for each plant were manually generated using Adobe Photoshop CS6 (Adobe Systems, CA, USA). Every leaf mask was stored in a separate layer in the format of Leaf No. If the leaf was not visible due to occlusions, an empty layer was included with a title of the missing leaf number. Annotated image layers were stored in a psd file format.
- Ground truth (leaf labels) images: .png images generated from the .psd files (B)
- Albedo images (C)
- Surface normal map images (D)
- Grayscale images — mean of various illumination directions (E)
- Shadow images (F)
- Composite images — normals in x and y directions, and albedo (G)
- Foreground_Background images — a foreground (white) and background (black) image mask (H).
Summary #
Photometric Stereo Training Data Set with Annotated Leaf Masks is a dataset for instance segmentation, semantic segmentation, and object detection tasks. It is used in the biological and genetic research.
The dataset consists of 4818 images with 33154 labeled objects belonging to 1 single class (leaf).
Images in the Photometric Stereo Leafs dataset have pixel-level instance segmentation annotations. Due to the nature of the instance segmentation task, it can be automatically transformed into a semantic segmentation (only one mask for every class) or object detection (bounding boxes for every object) tasks. All images are labeled (i.e. with annotations). There are 2 splits in the dataset: test (3492 images) and train (1326 images). Decomposed multi-channel train images are grouped by unique im_id into 221 groups with 6 layers each: Albedo images, Surface normal map images, Grayscale images, Shadow images, Composite images, and Foreground_Background images. Alternatively, the dataset could be split into 4 test images layers: Binary masks (873 images), CroppedAlbedo (873 images), CroppedComposite (873 images), and CroppedGrayscale (873 images). Additionally, images metadata contains information about leaf number, plant number, lights, region of interest, days after germination,interval between imaging, growth light intensity, growth temperature, and the growth light intensity. The dataset was released in 2019 by the University of Edinburgh, Scotland.
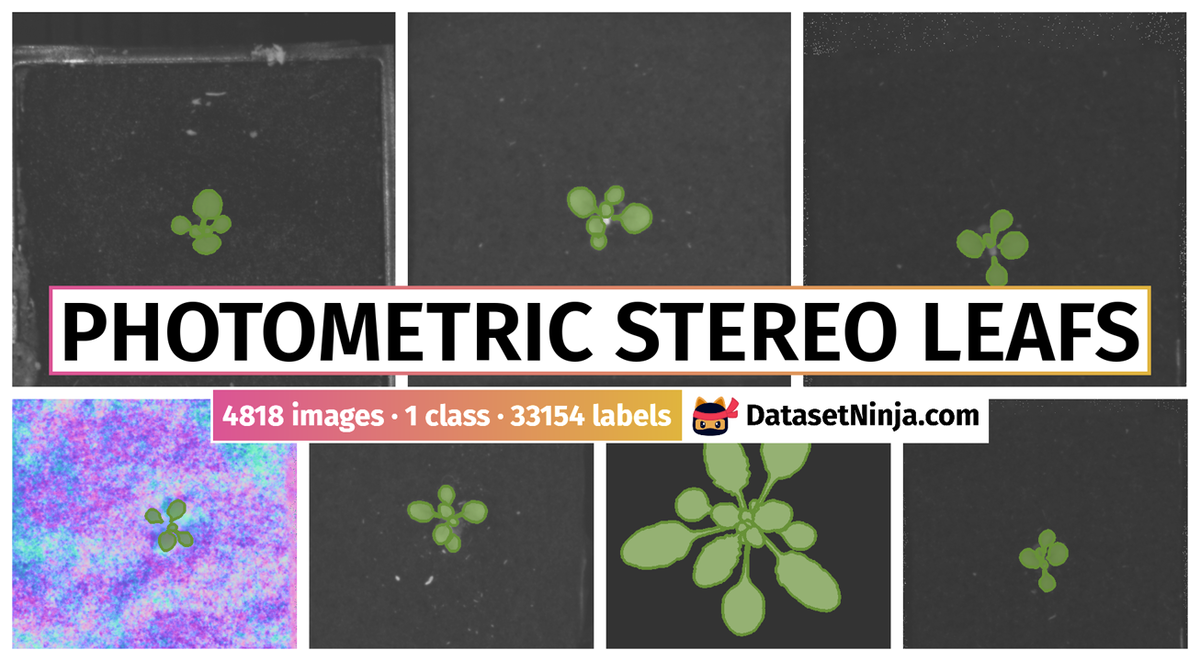
Explore #
Photometric Stereo Leafs dataset has 4818 images. Click on one of the examples below or open "Explore" tool anytime you need to view dataset images with annotations. This tool has extended visualization capabilities like zoom, translation, objects table, custom filters and more. Hover the mouse over the images to hide or show annotations.
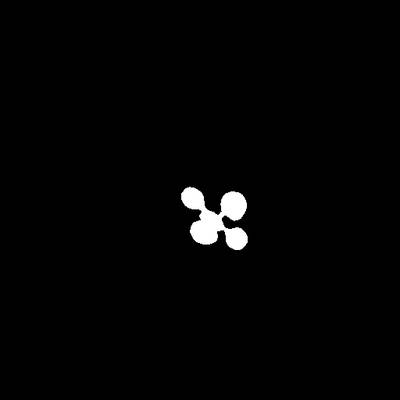
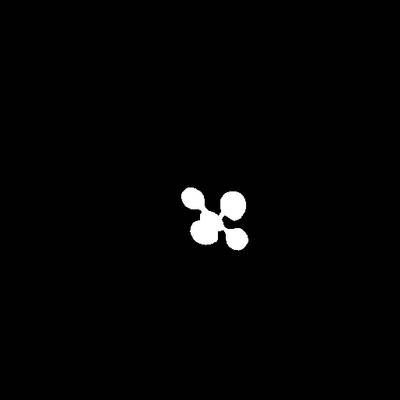
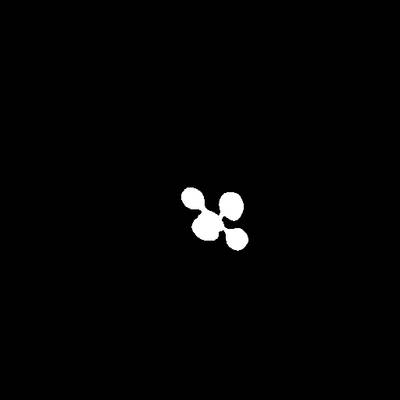
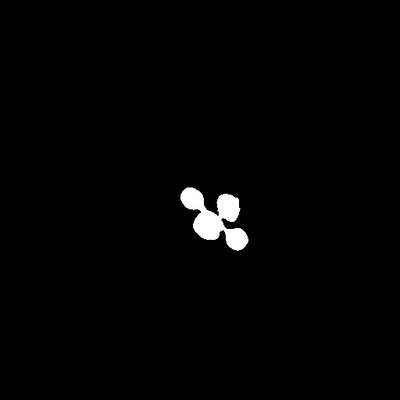
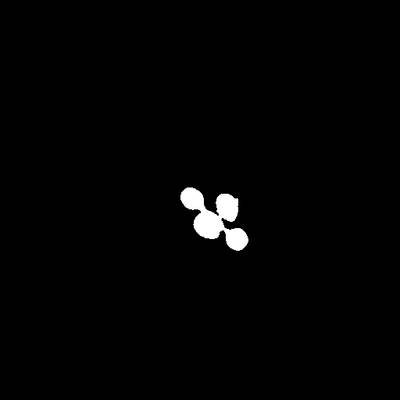
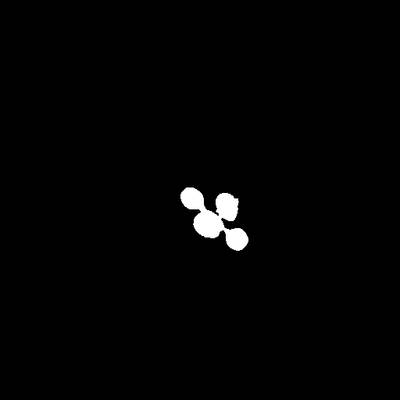
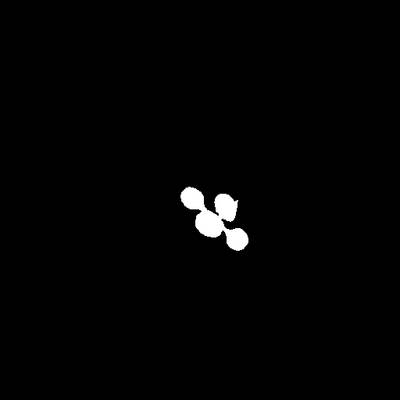
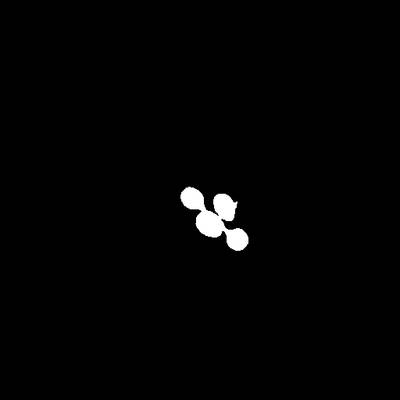
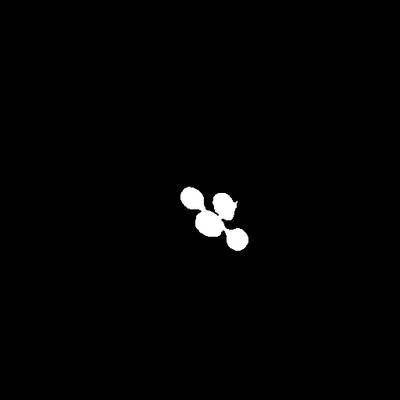
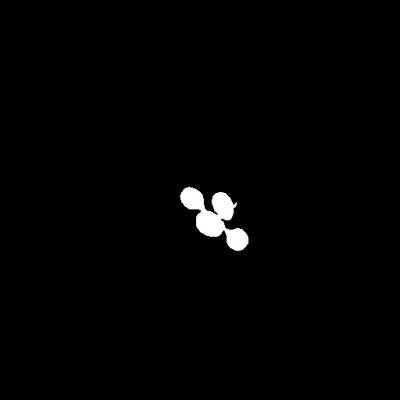
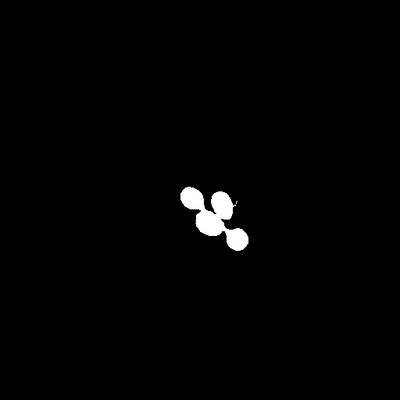
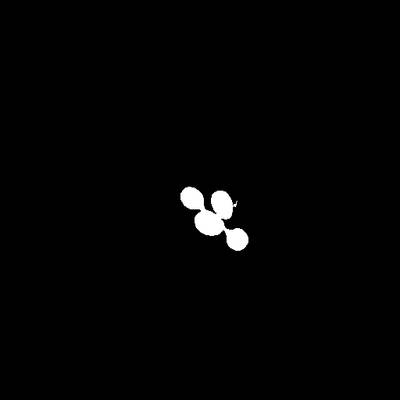

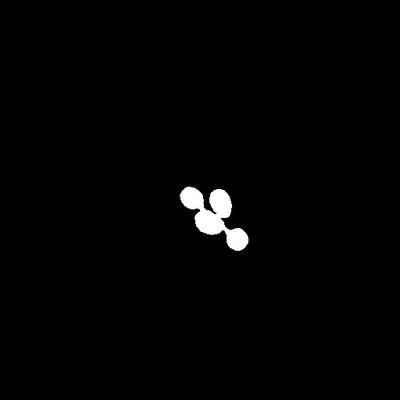
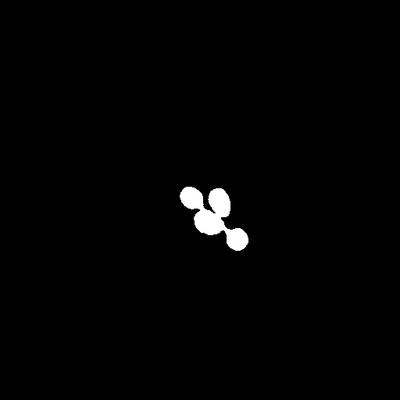
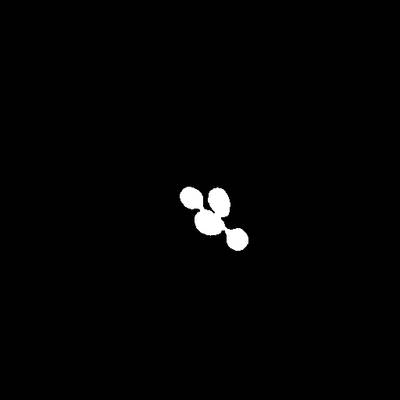
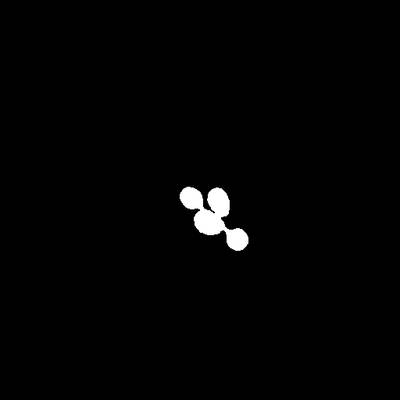
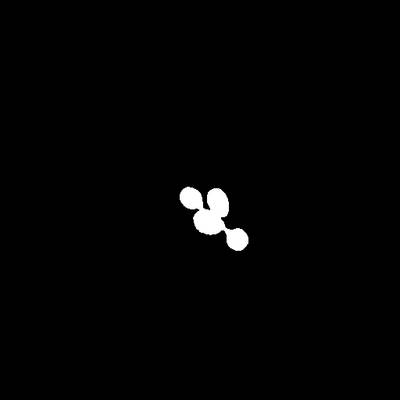
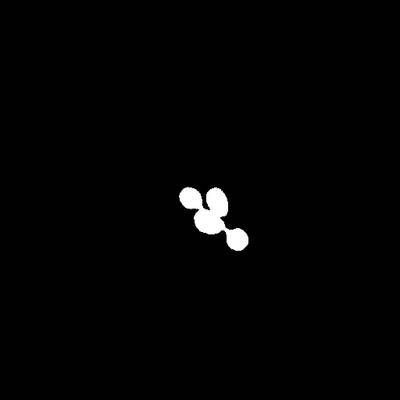

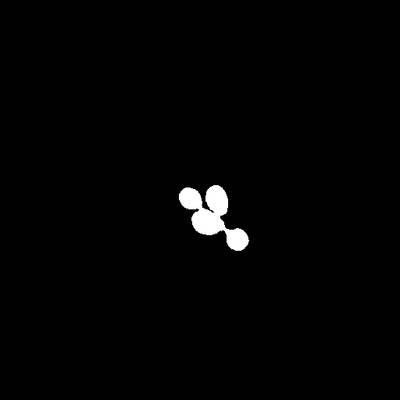
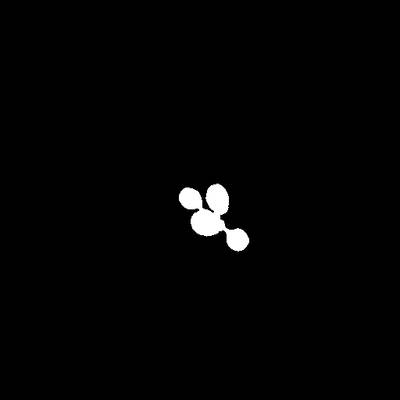
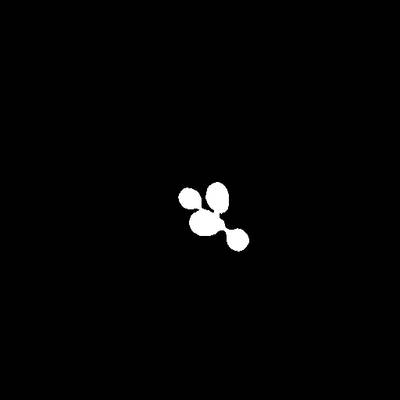
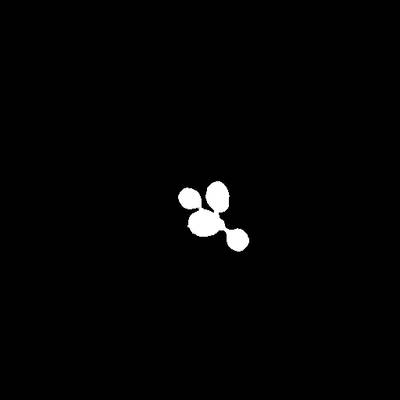
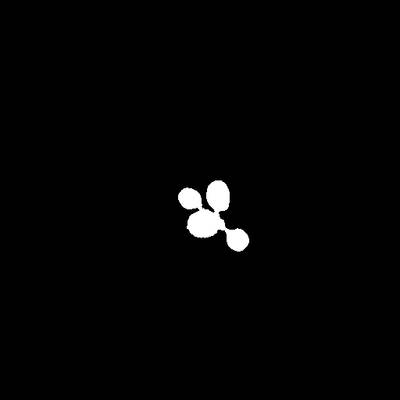
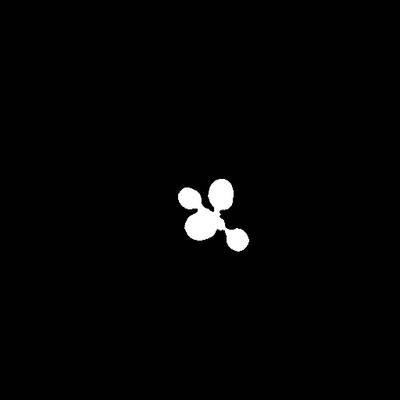
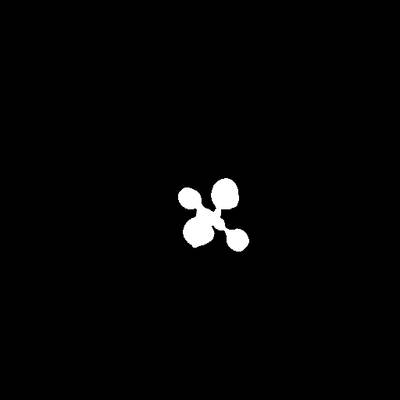
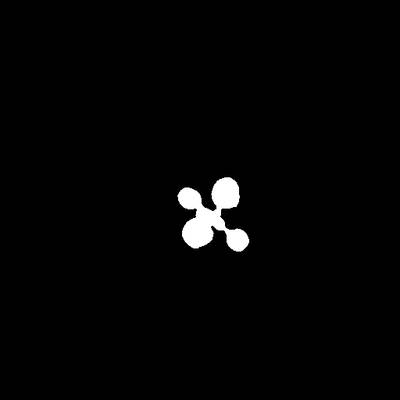

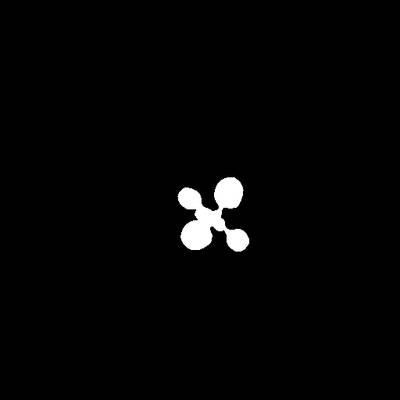
Class balance #
There are 1 annotation classes in the dataset. Find the general statistics and balances for every class in the table below. Click any row to preview images that have labels of the selected class. Sort by column to find the most rare or prevalent classes.
Class ã…¤ | Images ã…¤ | Objects ã…¤ | Count on image average | Area on image average |
---|---|---|---|---|
leafâž” mask | 4818 | 33154 | 6.88 | 3.54% |
Images #
Explore every single image in the dataset with respect to the number of annotations of each class it has. Click a row to preview selected image. Sort by any column to find anomalies and edge cases. Use horizontal scroll if the table has many columns for a large number of classes in the dataset.
Object distribution #
Interactive heatmap chart for every class with object distribution shows how many images are in the dataset with a certain number of objects of a specific class. Users can click cell and see the list of all corresponding images.
Class sizes #
The table below gives various size properties of objects for every class. Click a row to see the image with annotations of the selected class. Sort columns to find classes with the smallest or largest objects or understand the size differences between classes.
Class | Object count | Avg area | Max area | Min area | Min height | Min height | Max height | Max height | Avg height | Avg height | Min width | Min width | Max width | Max width |
---|---|---|---|---|---|---|---|---|---|---|---|---|---|---|
leaf mask | 33154 | 0.51% | 6.25% | 0% | 1px | 0.18% | 276px | 49.29% | 44px | 8.08% | 1px | 0.15% | 326px | 50.15% |
Spatial Heatmap #
The heatmaps below give the spatial distributions of all objects for every class. These visualizations provide insights into the most probable and rare object locations on the image. It helps analyze objects' placements in a dataset.
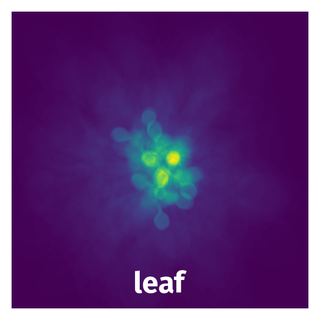
Objects #
Table contains all 33154 objects. Click a row to preview an image with annotations, and use search or pagination to navigate. Sort columns to find outliers in the dataset.
Object ID ã…¤ | Class ã…¤ | Image name click row to open | Image size height x width | Height ã…¤ | Height ã…¤ | Width ã…¤ | Width ã…¤ | Area ã…¤ |
---|---|---|---|---|---|---|---|---|
1âž” | leaf mask | 1_2019-01-27_11-00-16_VISNIR_albedo.png | 512 x 512 | 35px | 6.84% | 56px | 10.94% | 0.48% |
2âž” | leaf mask | 1_2019-01-27_11-00-16_VISNIR_albedo.png | 512 x 512 | 40px | 7.81% | 66px | 12.89% | 0.6% |
3âž” | leaf mask | 1_2019-01-27_11-00-16_VISNIR_albedo.png | 512 x 512 | 18px | 3.52% | 18px | 3.52% | 0.09% |
4âž” | leaf mask | 1_2019-01-27_11-00-16_VISNIR_albedo.png | 512 x 512 | 23px | 4.49% | 27px | 5.27% | 0.18% |
5âž” | leaf mask | 1_2019-01-27_11-00-16_VISNIR_albedo.png | 512 x 512 | 41px | 8.01% | 27px | 5.27% | 0.26% |
6âž” | leaf mask | 1_2019-01-27_11-00-16_VISNIR_albedo.png | 512 x 512 | 45px | 8.79% | 28px | 5.47% | 0.26% |
7âž” | leaf mask | 1_2019-01-26_07-30-23_VISNIR_albedo.png | 512 x 512 | 34px | 6.64% | 36px | 7.03% | 0.35% |
8âž” | leaf mask | 1_2019-01-26_07-30-23_VISNIR_albedo.png | 512 x 512 | 34px | 6.64% | 31px | 6.05% | 0.32% |
9âž” | leaf mask | 1_2019-01-26_07-30-23_VISNIR_albedo.png | 512 x 512 | 39px | 7.62% | 28px | 5.47% | 0.25% |
10âž” | leaf mask | 1_2019-01-26_07-30-23_VISNIR_albedo.png | 512 x 512 | 42px | 8.2% | 26px | 5.08% | 0.25% |
License #
Citation #
If you make use of the Photometric stereo leafs data, please cite the following reference:
Scorza, Livia; Bernotas, Gytis; McCormick, Alistair. (2019).
Photometric stereo training data set with annotated leaf masks, [dataset].
University of Edinburgh. School of Biological Sciences. Institute of Molecular Plant Sciences.
https://doi.org/10.7488/ds/2514.
If you are happy with Dataset Ninja and use provided visualizations and tools in your work, please cite us:
@misc{ visualization-tools-for-photometric-stereo-leafs-dataset,
title = { Visualization Tools for Photometric Stereo Leafs Dataset },
type = { Computer Vision Tools },
author = { Dataset Ninja },
howpublished = { \url{ https://datasetninja.com/photometric-stereo-leafs } },
url = { https://datasetninja.com/photometric-stereo-leafs },
journal = { Dataset Ninja },
publisher = { Dataset Ninja },
year = { 2025 },
month = { apr },
note = { visited on 2025-04-26 },
}
Download #
Dataset Photometric Stereo Leafs can be downloaded in Supervisely format:
As an alternative, it can be downloaded with dataset-tools package:
pip install --upgrade dataset-tools
… using following python code:
import dataset_tools as dtools
dtools.download(dataset='Photometric Stereo Leafs', dst_dir='~/dataset-ninja/')
Make sure not to overlook the python code example available on the Supervisely Developer Portal. It will give you a clear idea of how to effortlessly work with the downloaded dataset.
The data in original format can be downloaded here:
Disclaimer #
Our gal from the legal dep told us we need to post this:
Dataset Ninja provides visualizations and statistics for some datasets that can be found online and can be downloaded by general audience. Dataset Ninja is not a dataset hosting platform and can only be used for informational purposes. The platform does not claim any rights for the original content, including images, videos, annotations and descriptions. Joint publishing is prohibited.
You take full responsibility when you use datasets presented at Dataset Ninja, as well as other information, including visualizations and statistics we provide. You are in charge of compliance with any dataset license and all other permissions. You are required to navigate datasets homepage and make sure that you can use it. In case of any questions, get in touch with us at hello@datasetninja.com.