Introduction #
The authors of the UAVid: A Semantic Segmentation Dataset for UAV Imagery dataset discussed the significance of semantic segmentation, a crucial aspect of visual scene understanding, with applications in fields such as robotics and autonomous driving. They noted that the success of semantic segmentation owes much to large-scale datasets, particularly for deep learning methods. While several datasets existed for semantic segmentation in complex urban scenes, capturing side views of objects from mounted cameras on driving cars, there was a dearth of datasets capturing urban scenes from an oblique Unmanned Aerial Vehicle (UAV) perspective. Such oblique views provide both top and side views of objects, offering richer information for object recognition. To address this gap, the authors introduced the UAVid dataset, which presented new challenges, including variations in scale, moving object recognition, and maintaining temporal consistency.
The UAVid dataset comprised 30 video sequences capturing high-resolution images from oblique UAV perspectives. A total of 300 images were densely labeled with annotations for eight semantic classes. The authors also provided several deep learning baseline methods with pre-training.
They considered various factors when creating the dataset, including the oblique view from the UAV platform, high resolution, consecutive labeling, complex and dynamic scenes, data variation across 30 different places, and the use of modern lightweight drones for data collection.
The authors highlighted the higher scene complexity of the UAVid dataset compared to existing UAV semantic segmentation datasets, particularly in terms of the number of objects and object configurations. They noted that their dataset was moderately sized but had a comparable or larger number of labeled pixels compared to well-known semantic segmentation datasets.
They defined eight semantic classes for the dataset:
- building: living houses, garages, skyscrapers, security booths, and
buildings under construction. Freestanding walls and fences are not
included. - road: road or bridge surface that cars can run on legally. Parking
lots are not included. - tree: tall trees that have canopies and main trunks.
- low vegetation: grass, bushes and shrubs.
- static car: cars that are not moving, including static buses, trucks,
automobiles, and tractors. Bicycles and motorcycles are not included. - moving car: cars that are moving, including moving buses, trucks,
automobiles, and tractors. Bicycles and motorcycles are not included. - human: pedestrians, bikers, and all other humans occupied by different activities.
- clutter: all objects not belonging to any of the classes above.
The car class was deliberately divided into moving car and static car. Moving car is such a special class designed for moving object segmentation. Other classes can be inferred from their appearance and context, while the moving car class may need additional temporal information in order to be appropriately separated from static car class. Achieving high accuracy for both static and moving car classes is one possible research goal for the dataset.
The authors described their annotation methodology, which included pixel-level, super-pixel level, and polygon-level annotation methods. These methods allowed annotators to efficiently label objects with different characteristics, such as trees with sawtooth boundaries and buildings with straight boundaries. The labeling tool also provided video play functionality for annotators to inspect object motion.
Lastly, they detailed the dataset splits, dividing the 30 video sequences into training, validation, and test splits. The data was split at the sequence level, ensuring that each split represented the scene variability adequately. The test split contained withheld labels for benchmarking purposes, while the training and validation splits were made publicly available, comprising a total of 200 labeled images. The size ratios among the splits were maintained at 3:1:2 (training:validation:test).
Summary #
UAVid: A Semantic Segmentation Dataset for UAV Imagery is a dataset for instance segmentation, semantic segmentation, and object detection tasks. It is used in the drone inspection domain, and in the surveillance, traffic monitoring, and smart city industries.
The dataset consists of 420 images with 43104 labeled objects belonging to 8 different classes including tree, low vegetation, building, and other: road, static car, human, moving car, and background clutter.
Images in the UAVid dataset have pixel-level instance segmentation annotations. Due to the nature of the instance segmentation task, it can be automatically transformed into a semantic segmentation (only one mask for every class) or object detection (bounding boxes for every object) tasks. There are 150 (36% of the total) unlabeled images (i.e. without annotations). There are 3 splits in the dataset: train (200 images), test (150 images), and val (70 images). Additionally, every image contains the id of its video sequence (total 30). The dataset was released in 2020 by the University of Twente, Netherlands, Wuhan University, China, and Ohio State University, USA.
Here is the visualized example grid with animated annotations:
Explore #
UAVid dataset has 420 images. Click on one of the examples below or open "Explore" tool anytime you need to view dataset images with annotations. This tool has extended visualization capabilities like zoom, translation, objects table, custom filters and more. Hover the mouse over the images to hide or show annotations.
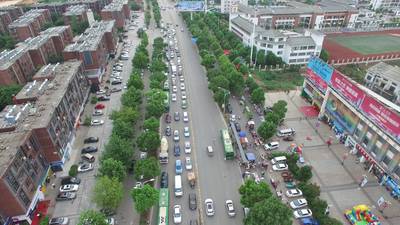
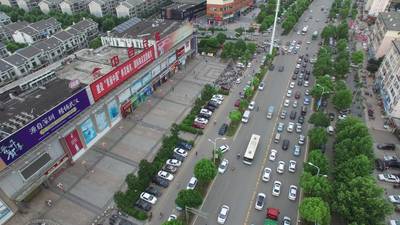
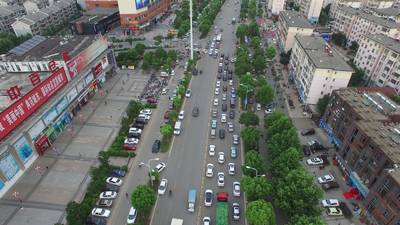
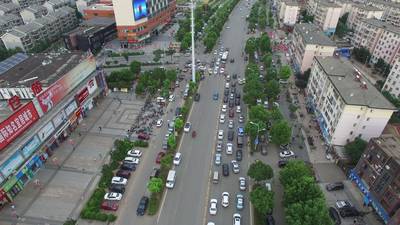
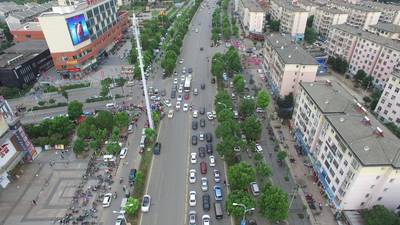
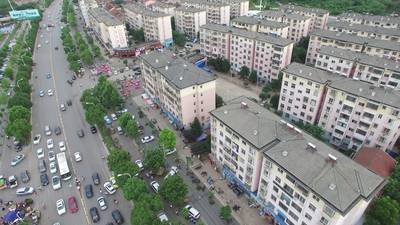

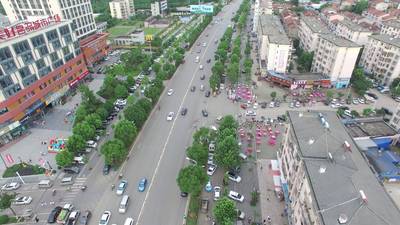
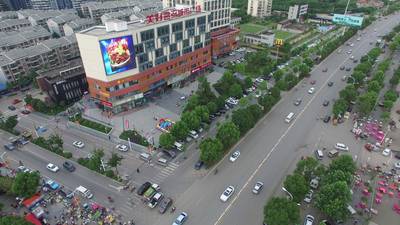
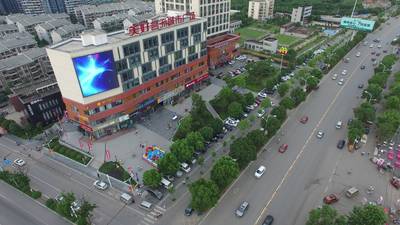
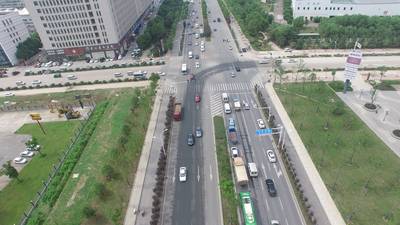
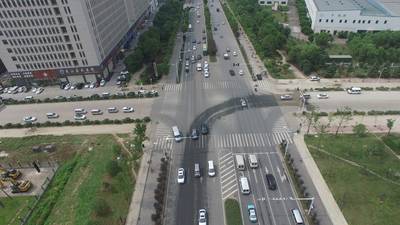
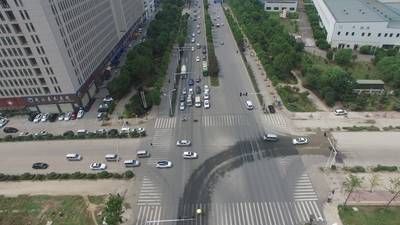
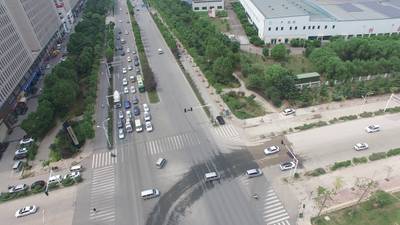
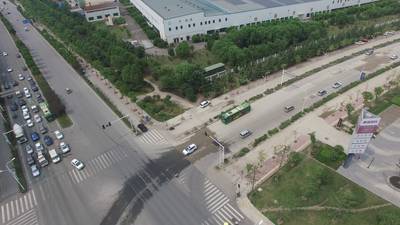

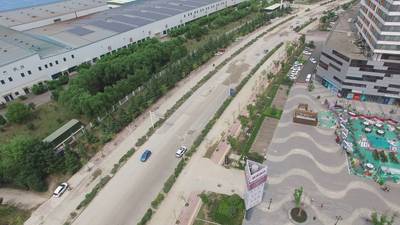
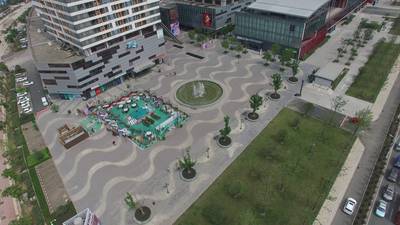
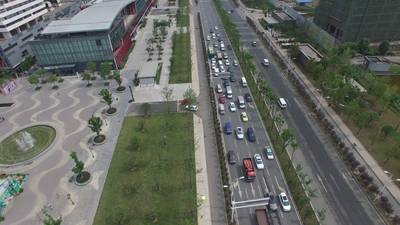
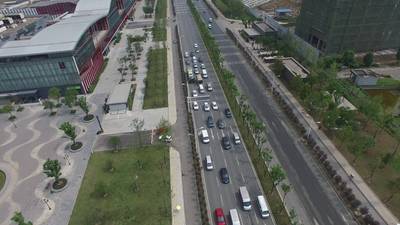
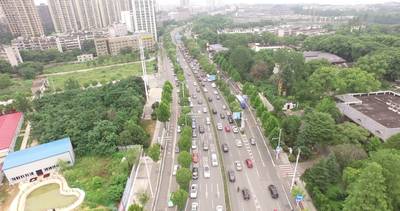

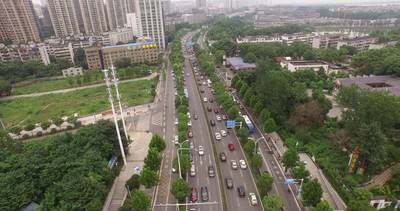
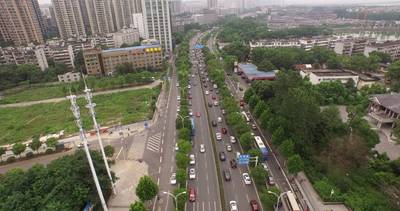
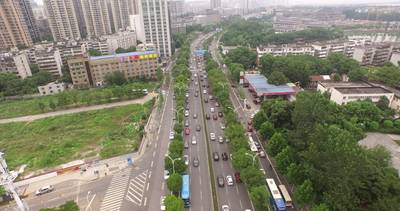
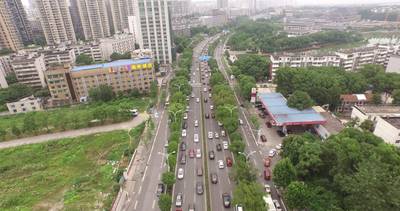
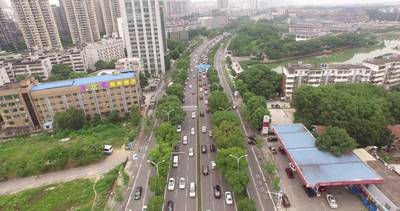
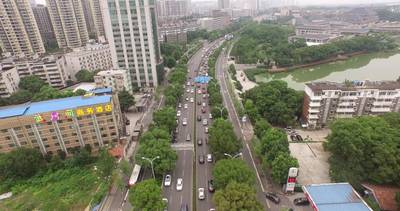


Class balance #
There are 8 annotation classes in the dataset. Find the general statistics and balances for every class in the table below. Click any row to preview images that have labels of the selected class. Sort by column to find the most rare or prevalent classes.
Class ã…¤ | Images ã…¤ | Objects ã…¤ | Count on image average | Area on image average |
---|---|---|---|---|
treeâž” mask | 270 | 11503 | 42.6 | 24.84% |
low vegetationâž” mask | 270 | 8118 | 30.07 | 13.72% |
roadâž” mask | 269 | 2980 | 11.08 | 12.1% |
buildingâž” mask | 269 | 2672 | 9.93 | 30.01% |
static carâž” mask | 261 | 5541 | 21.23 | 1.16% |
humanâž” mask | 232 | 4655 | 20.06 | 0.15% |
moving carâž” mask | 205 | 7635 | 37.24 | 1.19% |
background clutterâž” mask | 0 | 0 | 0 | 0% |
Co-occurrence matrix #
Co-occurrence matrix is an extremely valuable tool that shows you the images for every pair of classes: how many images have objects of both classes at the same time. If you click any cell, you will see those images. We added the tooltip with an explanation for every cell for your convenience, just hover the mouse over a cell to preview the description.
Images #
Explore every single image in the dataset with respect to the number of annotations of each class it has. Click a row to preview selected image. Sort by any column to find anomalies and edge cases. Use horizontal scroll if the table has many columns for a large number of classes in the dataset.
Class sizes #
The table below gives various size properties of objects for every class. Click a row to see the image with annotations of the selected class. Sort columns to find classes with the smallest or largest objects or understand the size differences between classes.
Class | Object count | Avg area | Max area | Min area | Min height | Min height | Max height | Max height | Avg height | Avg height | Min width | Min width | Max width | Max width |
---|---|---|---|---|---|---|---|---|---|---|---|---|---|---|
tree mask | 11503 | 0.58% | 62.35% | 0% | 5px | 0.23% | 2160px | 100% | 180px | 8.35% | 5px | 0.12% | 4096px | 100% |
low vegetation mask | 8118 | 0.46% | 92.94% | 0% | 2px | 0.09% | 2160px | 100% | 172px | 7.95% | 3px | 0.08% | 4096px | 100% |
moving car mask | 7635 | 0.03% | 1.05% | 0% | 7px | 0.32% | 612px | 28.33% | 58px | 2.69% | 8px | 0.2% | 659px | 17.16% |
static car mask | 5541 | 0.05% | 2.13% | 0% | 7px | 0.32% | 850px | 39.35% | 68px | 3.16% | 10px | 0.24% | 1366px | 33.35% |
human mask | 4655 | 0.01% | 0.19% | 0% | 11px | 0.51% | 245px | 11.34% | 37px | 1.7% | 6px | 0.15% | 266px | 6.93% |
road mask | 2980 | 1.09% | 42.36% | 0% | 2px | 0.09% | 2160px | 100% | 293px | 13.54% | 3px | 0.08% | 4096px | 100% |
building mask | 2672 | 3.02% | 82.22% | 0% | 1px | 0.05% | 2160px | 100% | 330px | 15.26% | 1px | 0.03% | 4096px | 100% |
Spatial Heatmap #
The heatmaps below give the spatial distributions of all objects for every class. These visualizations provide insights into the most probable and rare object locations on the image. It helps analyze objects' placements in a dataset.
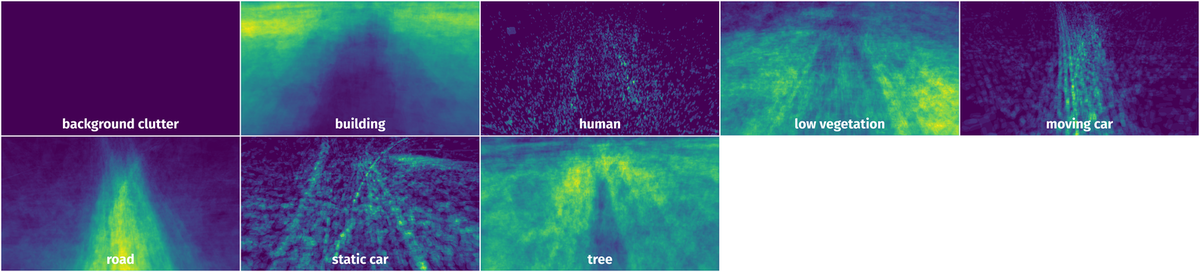
Objects #
Table contains all 43104 objects. Click a row to preview an image with annotations, and use search or pagination to navigate. Sort columns to find outliers in the dataset.
Object ID ã…¤ | Class ã…¤ | Image name click row to open | Image size height x width | Height ã…¤ | Height ã…¤ | Width ã…¤ | Width ã…¤ | Area ã…¤ |
---|---|---|---|---|---|---|---|---|
1âž” | tree mask | seq31_000200.png | 2160 x 4096 | 38px | 1.76% | 65px | 1.59% | 0.01% |
2âž” | tree mask | seq31_000200.png | 2160 x 4096 | 40px | 1.85% | 33px | 0.81% | 0% |
3âž” | tree mask | seq31_000200.png | 2160 x 4096 | 69px | 3.19% | 154px | 3.76% | 0.07% |
4âž” | tree mask | seq31_000200.png | 2160 x 4096 | 139px | 6.44% | 342px | 8.35% | 0.33% |
5âž” | tree mask | seq31_000200.png | 2160 x 4096 | 137px | 6.34% | 131px | 3.2% | 0.05% |
6âž” | tree mask | seq31_000200.png | 2160 x 4096 | 136px | 6.3% | 222px | 5.42% | 0.28% |
7âž” | tree mask | seq31_000200.png | 2160 x 4096 | 465px | 21.53% | 201px | 4.91% | 0.37% |
8âž” | tree mask | seq31_000200.png | 2160 x 4096 | 416px | 19.26% | 424px | 10.35% | 1.06% |
9âž” | tree mask | seq31_000200.png | 2160 x 4096 | 124px | 5.74% | 99px | 2.42% | 0.09% |
10âž” | tree mask | seq31_000200.png | 2160 x 4096 | 65px | 3.01% | 122px | 2.98% | 0.06% |
License #
UAVid: A Semantic Segmentation Dataset for UAV Imagery is under CC BY-NC-SA 4.0 license.
Citation #
If you make use of the UAVid data, please cite the following reference:
@article{LYU2020108,
author = "Ye Lyu and George Vosselman and Gui-Song Xia and Alper Yilmaz and Michael Ying Yang",
title = "UAVid: A semantic segmentation dataset for UAV imagery",
journal = "ISPRS Journal of Photogrammetry and Remote Sensing",
volume = "165",
pages = "108 - 119",
year = "2020",
issn = "0924-2716",
doi = "https://doi.org/10.1016/j.isprsjprs.2020.05.009",
url = "http://www.sciencedirect.com/science/article/pii/S0924271620301295",
}
When using the UAVid-depth dataset in your research, please cite:
@article{uaviddepth21,
Author = {Logambal Madhuanand and Francesco Nex and Michael Ying Yang},
Title = {Self-supervised monocular depth estimation from oblique UAV videos},
journal = {ISPRS Journal of Photogrammetry and Remote Sensing},
year = {2021},
volume = {176},
pages = {1-14},
}
If you are happy with Dataset Ninja and use provided visualizations and tools in your work, please cite us:
@misc{ visualization-tools-for-uavid-dataset,
title = { Visualization Tools for UAVid Dataset },
type = { Computer Vision Tools },
author = { Dataset Ninja },
howpublished = { \url{ https://datasetninja.com/uavid } },
url = { https://datasetninja.com/uavid },
journal = { Dataset Ninja },
publisher = { Dataset Ninja },
year = { 2025 },
month = { apr },
note = { visited on 2025-04-02 },
}
Download #
Dataset UAVid can be downloaded in Supervisely format:
As an alternative, it can be downloaded with dataset-tools package:
pip install --upgrade dataset-tools
… using following python code:
import dataset_tools as dtools
dtools.download(dataset='UAVid', dst_dir='~/dataset-ninja/')
Make sure not to overlook the python code example available on the Supervisely Developer Portal. It will give you a clear idea of how to effortlessly work with the downloaded dataset.
The data in original format can be downloaded here.
Disclaimer #
Our gal from the legal dep told us we need to post this:
Dataset Ninja provides visualizations and statistics for some datasets that can be found online and can be downloaded by general audience. Dataset Ninja is not a dataset hosting platform and can only be used for informational purposes. The platform does not claim any rights for the original content, including images, videos, annotations and descriptions. Joint publishing is prohibited.
You take full responsibility when you use datasets presented at Dataset Ninja, as well as other information, including visualizations and statistics we provide. You are in charge of compliance with any dataset license and all other permissions. You are required to navigate datasets homepage and make sure that you can use it. In case of any questions, get in touch with us at hello@datasetninja.com.